Topology-Aware GAN (TopoGAN): Transforming Medical Imaging Advances.
2023 Tenth International Conference on Social Networks Analysis, Management and Security (SNAMS)(2023)
Abstract
Generative Adversarial Networks (GANs) have gained prominence in medical imaging due to their ability to generate realistic images. Traditional GANs, however, often fail to capture intricate topological features such as holes and connectivity components in real images. This study applies TopoGAN, a recently developed model tailored for medical imaging. TopoGAN dynamically learns and incorporates topological features like connectedness and loops, addressing a real-world medical data augmentation problem. Utilizing a topological GAN loss function based on Persistent Homology and a new success metric, TopoGAN minimizes the topological discrepancy between synthetic and actual images. Experimental results, showcasing a Wasserstein distance of 0.0021 and a Dice coefficient of 0.995, highlight the model's efficacy in producing qualitatively rich synthetic images. This approach not only improves the realism of generated images but also enhances performance in downstream tasks such as image segmentation, offering a groundbreaking solution with significant implications for medical image analysis, diagnosis, and treatment planning.
MoreTranslated text
Key words
Topological data analysis,Persistent Homology,Generative Adversarial Network,Mathematics,Medical imaging
AI Read Science
Must-Reading Tree
Example
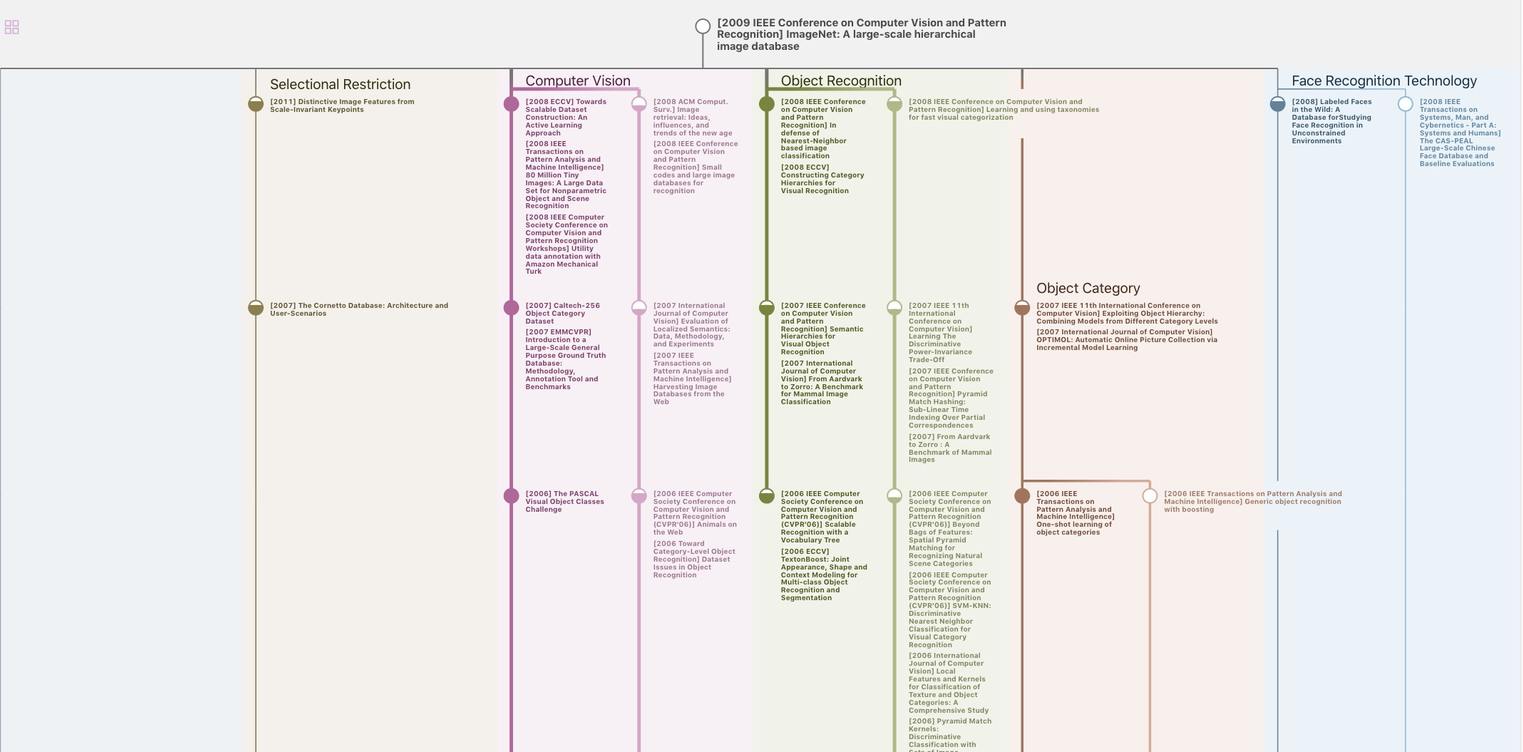
Generate MRT to find the research sequence of this paper
Chat Paper
Summary is being generated by the instructions you defined