Multi-domain Integrative Structure-enhanced and Sparse-coding Network for Sparse View CT Reconstruction.
2023 16th International Congress on Image and Signal Processing, BioMedical Engineering and Informatics (CISP-BMEI)(2023)
摘要
In order to decrease X-ray radiation dose and imaging time, it is crucial to employ a significant technique of minimizing the number of projections. Nevertheless, the presence of down sampling projection data may result in a decline in CT image quality caused by prominent streak artifacts. In this study, we present a novel network called MISS-net (multi-domain integrative structure-enhanced and sparse-coding network) to enhance image quality using sparse-view data. To begin with, MISS-net integrates hybrid domain features from sinogram data and CT images through a two-step network structure. Furthermore, in each stage, a dedicated subnetwork for enhancing tiny tissue is utilized to ensure the preservation of texture, while a subnetwork for extracting features through learned convolutional sparse coding is employed. Next, a distinct domain transformation converts the projection into an image, facilitating the exchange of information between the two domains. Experimental results shown that our proposed MISS-net outperformed the comparison methods in terms of image quality, exhibiting enhanced small features and sharp tissue. Additionally, it exhibited minimal structural loss in image quality, as evidenced by the experimental results on 64 views MAYO clinical datasets.
更多查看译文
关键词
CT reconstruction,sprese view,structure enhancement,sparse coding
AI 理解论文
溯源树
样例
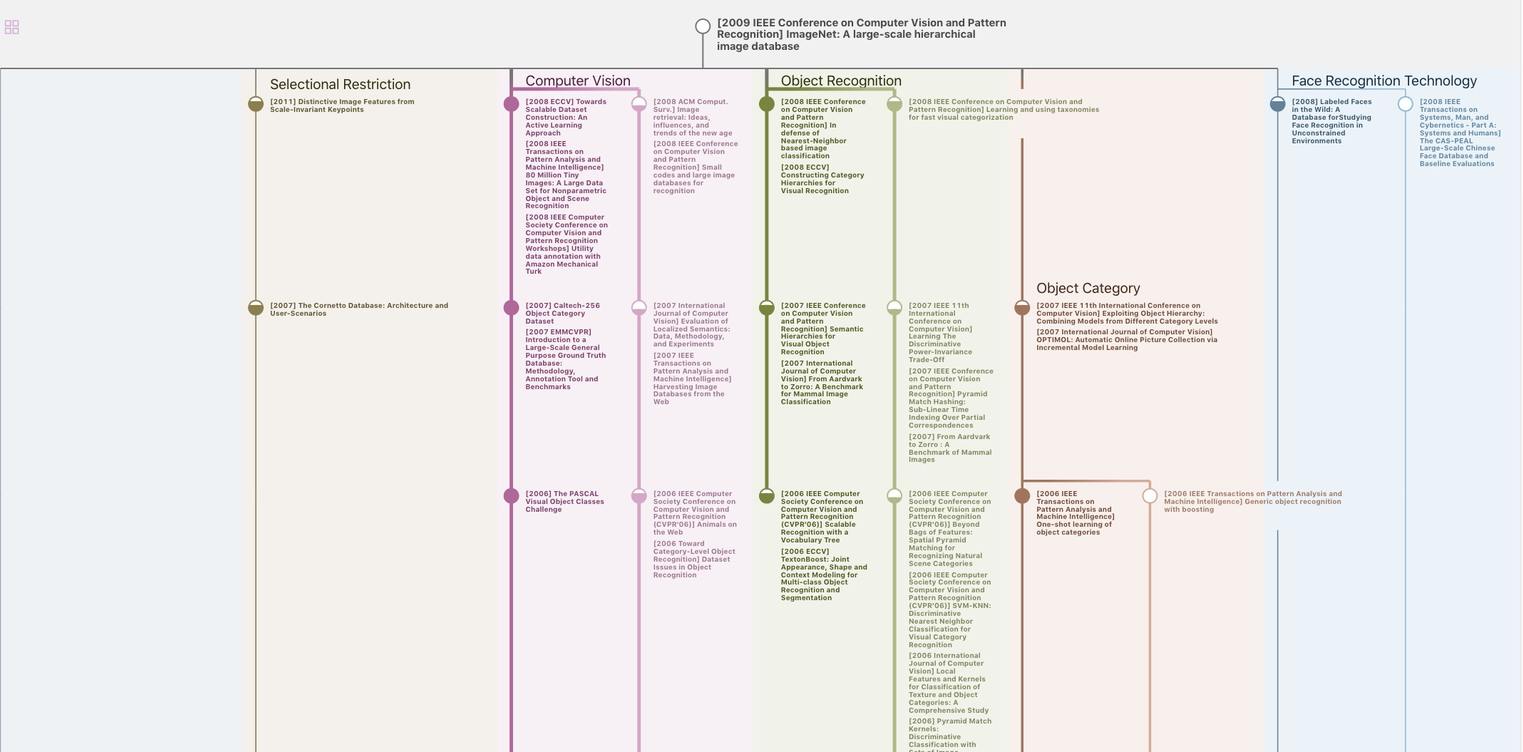
生成溯源树,研究论文发展脉络
Chat Paper
正在生成论文摘要