Classification of attention deficit hyperactivity disorder using machine learning on an EEG dataset
APPLIED NEUROPSYCHOLOGY-CHILD(2023)
Abstract
The neurodevelopmental disorder, Attention Deficit Hyperactivity Disorder (ADHD), frequently affecting youngsters, is characterized by persistent patterns of inattention, hyperactivity, and impulsivity, the etiology of which may involve a variety of genetic, environmental, and neurological factors. Electroencephalography (EEG) measures the electrical activity in the brain through neuronal activity, which is a function of cognitive processes. In this study, a previously recorded sample set of 121 children containing unbiased data from both ADHD and control group classes and EEG signals were analyzed to classify the ADHD patients. The samples were tested under different cognitive conditions, and multiple features were extracted using Euclidean distance. Many machine learning algorithms use Euclidean distance as their default distance metric to compare two recorded data points. The extracted features were trained using four supervised machine learning algorithms (linear regression, random forest, extreme gradient boosting, and K nearest neighbor (KNN)) based on the results of various frequency bands. The results suggest that the KNN algorithm produces the highest accuracy over other machine learning approaches, and results can be further improved with the application of hyperparameter tuning and used for classifying sub-groups of ADHD to identify the severity of the disorder.
MoreTranslated text
Key words
Attention deficit hyperactivity disorder,electroencephalography,K nearest neighbor,machine learning
AI Read Science
Must-Reading Tree
Example
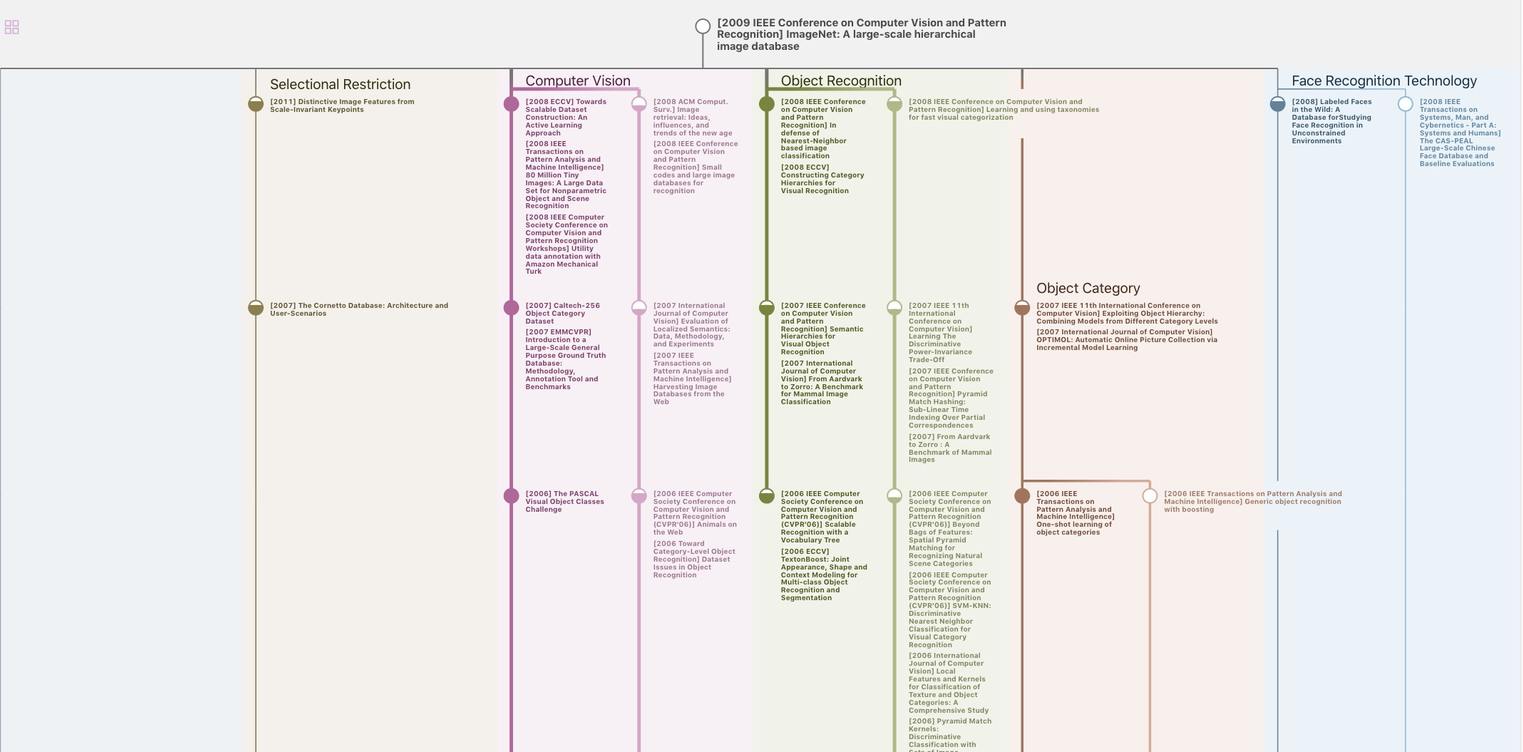
Generate MRT to find the research sequence of this paper
Chat Paper
Summary is being generated by the instructions you defined