Dynamic pruning-based Bayesian support vector regression for reliability analysis
RELIABILITY ENGINEERING & SYSTEM SAFETY(2024)
摘要
Adaptive surrogate-based reliability analysis methods have garnered significant attention due to their potential to enhance computational efficiency in accurately estimating failure probability. However, the candidate sample pool remains constant for most surrogate-based reliability methods, and traversing the candidate sample pool one by one will reduce the efficiency of surrogate modeling. More importantly, maintaining a static sample pool may lead to the inclusion of samples that contribute minimally to the construction of the surrogate modeling, thereby impacting the accuracy and efficiency of the reliability analysis. Accordingly, this paper leverages the robust performance of Bayesian support vector regression to propose a dynamic pruning strategy for the candidate sample pool to estimate failure probability efficiently. A dynamic pruning strategy is presented to streamline the process further, iteratively reducing the candidate sample pool. An adaptive learning algorithm is then introduced, integrating the U function and the sparsity of training samples. This is complemented by a formulated convergence condition, contributing to an ideal surrogate model. The proposed approaches showcase superior efficiency and accuracy through illustrations using well-known benchmark problems and complex reliability analysis problems involving small failure probability and high-dimensional limit state function.
更多查看译文
关键词
Reliability analysis,Bayesian support vector regression,Adaptive learning algorithm,Dynamic pruning strategy
AI 理解论文
溯源树
样例
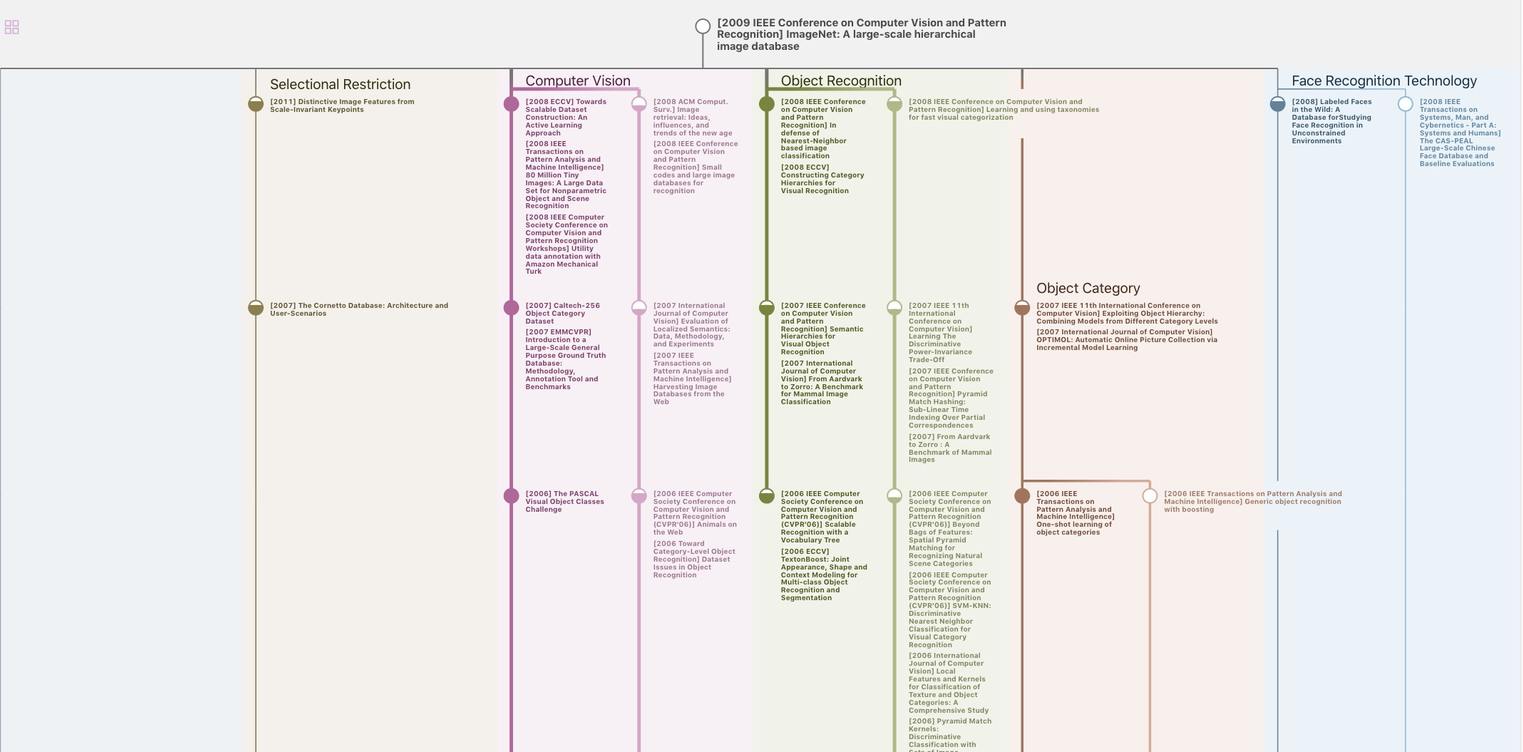
生成溯源树,研究论文发展脉络
Chat Paper
正在生成论文摘要