Efficient Sparse Least Absolute Deviation Regression With Differential Privacy
IEEE TRANSACTIONS ON INFORMATION FORENSICS AND SECURITY(2024)
摘要
In recent years, privacy-preserving machine learning algorithms have attracted increasing attention because of their important applications in many scientific fields. However, in the literature, most privacy-preserving algorithms demand learning objectives to be strongly convex and Lipschitz smooth, which thus cannot cover a wide class of robust loss functions (e.g., quantile/least absolute loss). In this work, we aim to develop a fast privacy-preserving learning solution for a sparse robust regression problem. Our learning loss consists of a robust least absolute loss and an & ell;(1) sparse penalty term. To fast solve the non-smooth loss under a given privacy budget, we develop a Fast Robust And Privacy-Preserving Estimation (FRAPPE) algorithm for least absolute deviation regression. Our algorithm achieves a fast estimation by reformulating the sparse LAD problem as a penalized least square estimation problem and adopts a three-stage noise injection to guarantee the (& varepsilon;,delta) -differential privacy. We show that our algorithm can achieve better privacy and statistical accuracy trade-off compared with the state-of-the-art privacy-preserving regression algorithms. In the end, we conduct experiments to verify the efficiency of our proposed FRAPPE algorithm.
更多查看译文
关键词
Robust regression,sparse learning,differential privacy,least absolute deviation
AI 理解论文
溯源树
样例
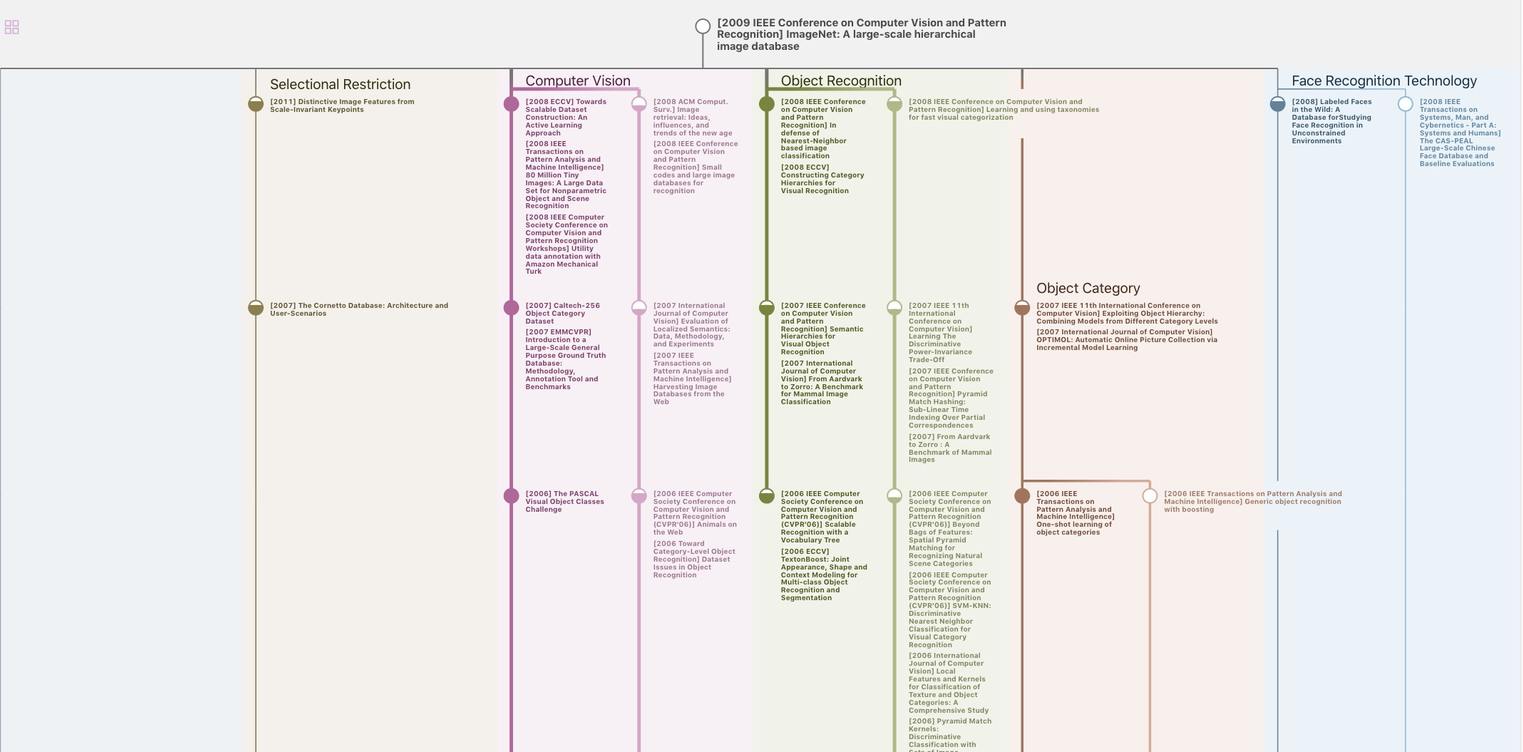
生成溯源树,研究论文发展脉络
Chat Paper
正在生成论文摘要