Zero-Shot Position Debiasing for Large Language Models
CoRR(2024)
摘要
Fine-tuning has been demonstrated to be an effective method to improve the
domain performance of large language models (LLMs). However, LLMs might fit the
dataset bias and shortcuts for prediction, leading to poor generation
performance. Previous works have proven that LLMs are prone to exhibit position
bias, i.e., leveraging information positioned at the beginning or end, or
specific positional cues within the input. Existing debiasing methods for LLMs
require external bias knowledge or annotated non-biased samples, which is
lacking for position debiasing and impractical in reality. In this work, we
propose a zero-shot position debiasing (ZOE) framework to mitigate position
bias for LLMs. ZOE leverages unsupervised responses from pre-trained LLMs for
debiasing without relying on any external knowledge. To improve the quality of
unsupervised responses, we propose a MSA module to prune these responses.
Experiments on eight datasets and five tasks show that ZOE consistently
outperforms existing methods in mitigating three types of position biases.
Besides, ZOE achieves this by sacrificing only a small performance on biased
samples, which is general and effective. To facilitate the reproducibility of
the results, we share the code of all methods and datasets on
https://anonymous.4open.science/r/ZOE-F06B.
更多查看译文
AI 理解论文
溯源树
样例
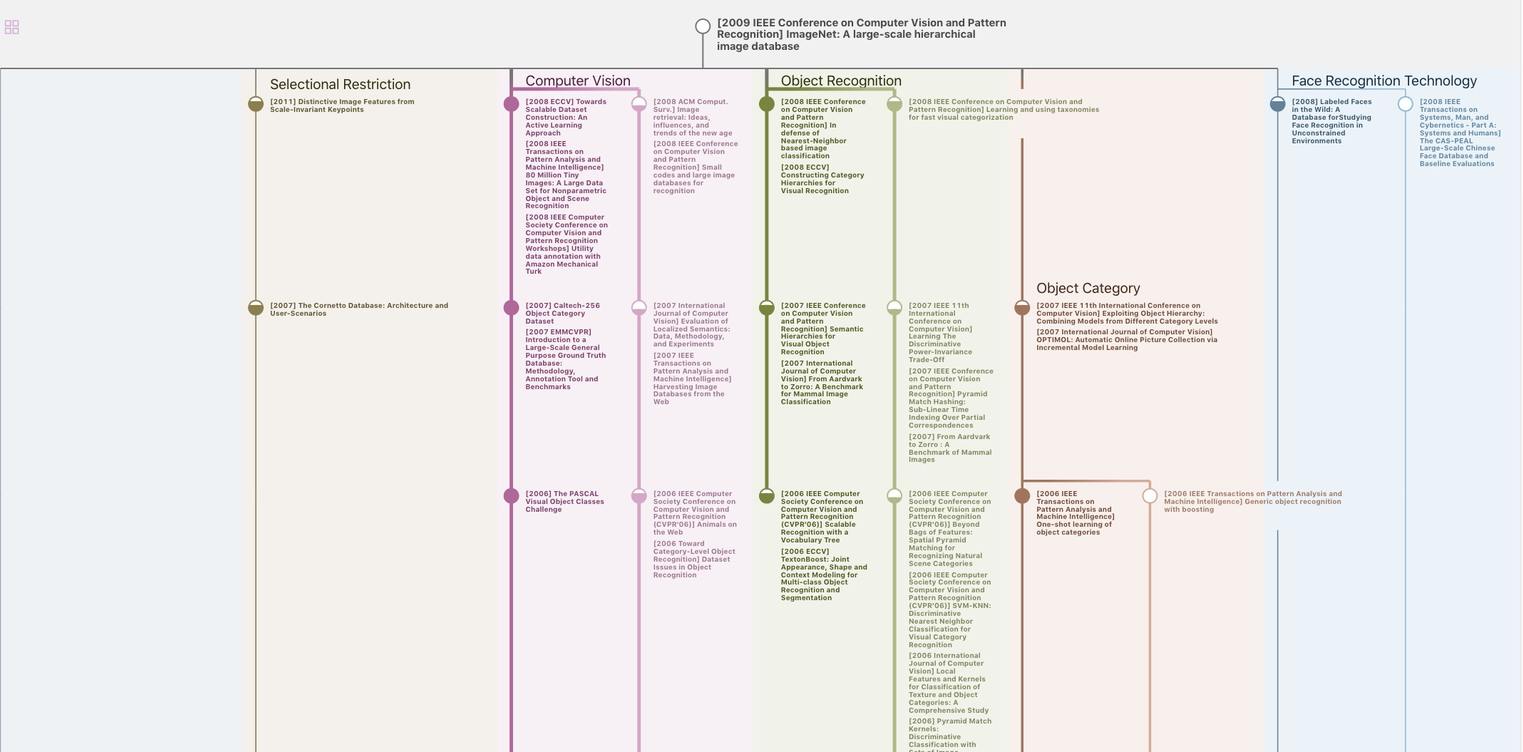
生成溯源树,研究论文发展脉络
Chat Paper
正在生成论文摘要