Enhancing Automatic Modulation Recognition through Robust Global Feature Extraction
CoRR(2024)
摘要
Automatic Modulation Recognition (AMR) plays a crucial role in wireless
communication systems. Deep learning AMR strategies have achieved tremendous
success in recent years. Modulated signals exhibit long temporal dependencies,
and extracting global features is crucial in identifying modulation schemes.
Traditionally, human experts analyze patterns in constellation diagrams to
classify modulation schemes. Classical convolutional-based networks, due to
their limited receptive fields, excel at extracting local features but struggle
to capture global relationships. To address this limitation, we introduce a
novel hybrid deep framework named TLDNN, which incorporates the architectures
of the transformer and long short-term memory (LSTM). We utilize the
self-attention mechanism of the transformer to model the global correlations in
signal sequences while employing LSTM to enhance the capture of temporal
dependencies. To mitigate the impact like RF fingerprint features and channel
characteristics on model generalization, we propose data augmentation
strategies known as segment substitution (SS) to enhance the model's robustness
to modulation-related features. Experimental results on widely-used datasets
demonstrate that our method achieves state-of-the-art performance and exhibits
significant advantages in terms of complexity. Our proposed framework serves as
a foundational backbone that can be extended to different datasets. We have
verified the effectiveness of our augmentation approach in enhancing the
generalization of the models, particularly in few-shot scenarios. Code is
available at .
更多查看译文
AI 理解论文
溯源树
样例
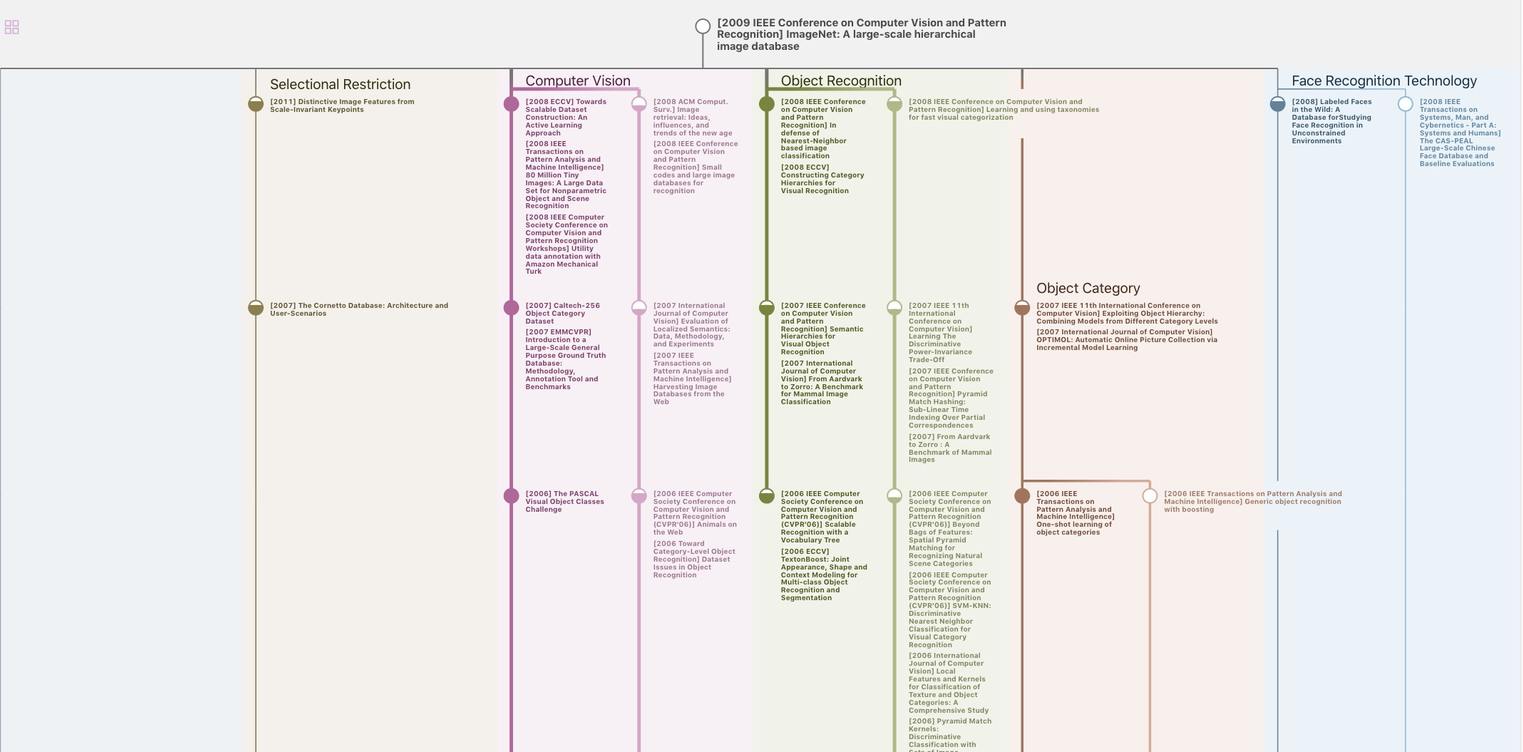
生成溯源树,研究论文发展脉络
Chat Paper
正在生成论文摘要