Fixed and random effect selections in generalized linear mixed models
STATISTICAL METHODS IN MEDICAL RESEARCH(2024)
Abstract
Generalized linear mixed models are commonly used to describe relationships between correlated responses and covariates in medical research. In this paper, we propose a simple and easily implementable regularized estimation approach to select both fixed and random effects in generalized linear mixed model. Specifically, we propose to construct and optimize the objective functions using the confidence distributions of model parameters, as opposed to using the observed data likelihood functions, to perform effect selections. Two estimation methods are developed. The first one is to use the joint confidence distribution of model parameters to perform simultaneous fixed and random effect selections. The second method is to use the marginal confidence distributions of model parameters to perform the selections of fixed and random effects separately. With a proper choice of regularization parameters in the adaptive LASSO framework, we show the consistency and oracle properties of the proposed regularized estimators. Simulation studies have been conducted to assess the performance of the proposed estimators and demonstrate computational efficiency. Our method has also been applied to two longitudinal cancer studies to identify demographic and clinical factors associated with patient health outcomes after cancer therapies.
MoreTranslated text
Key words
Confidence distribution,generalized linear mixed model,variable selection,regularization,adaptive Lasso
AI Read Science
Must-Reading Tree
Example
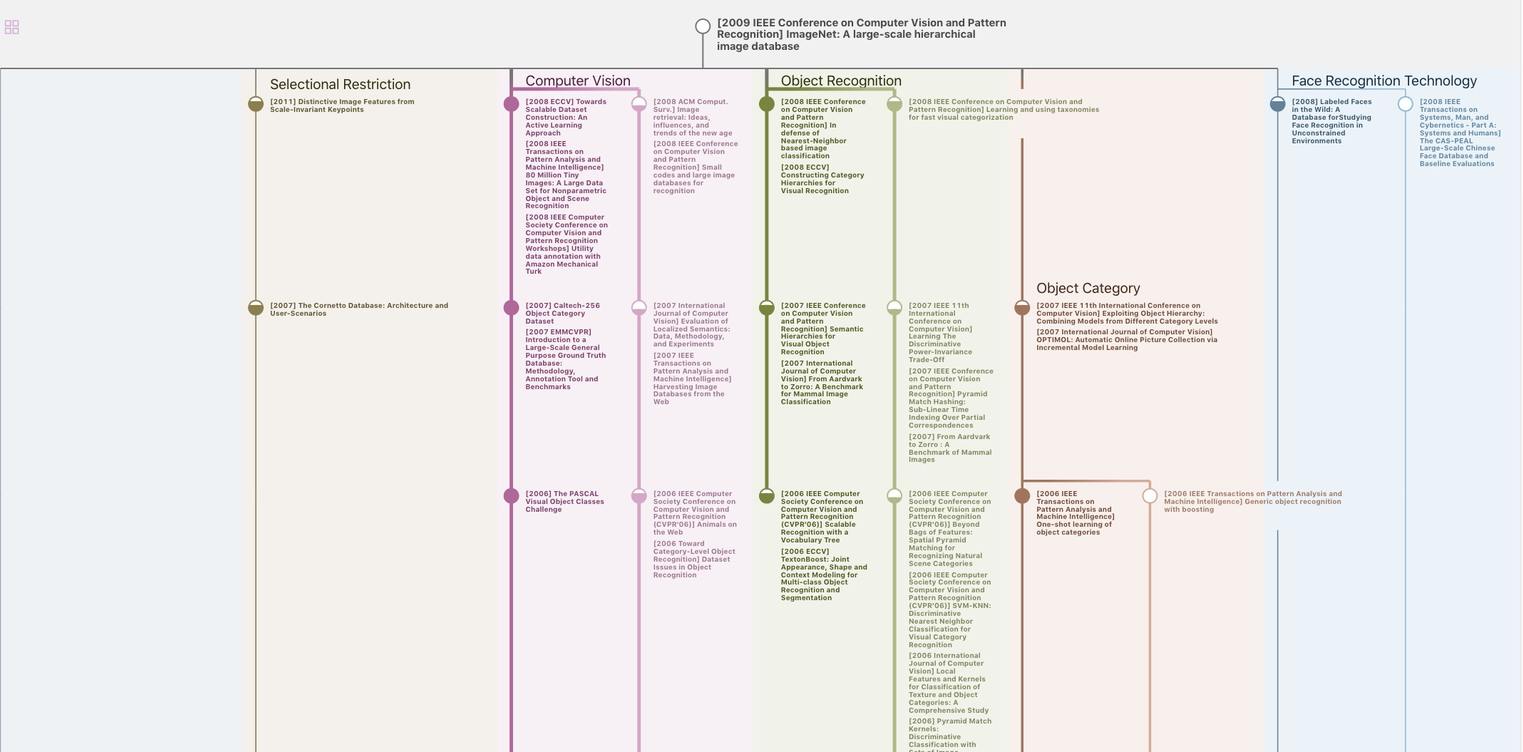
Generate MRT to find the research sequence of this paper
Chat Paper
Summary is being generated by the instructions you defined