Memory Shapelet Learning for Early Classification of Streaming Time Series
IEEE TRANSACTIONS ON CYBERNETICS(2024)
摘要
Early classification predicts the class of the incoming sequences before it is completely observed. How to quickly classify streaming time series without losing interpretability through early classification method is a challenging problem. A novel memory shapelet learning framework for early classification is proposed in this article. First, a memory distance matrix is introduced to store the historical characteristics of streaming time series, which can alleviate repetitive calculations caused by the growing length of time series. Second, early interpretable shapelets are extracted in the proposed method by optimizing both accuracy objective and earliness objective simultaneously. The proposed method employs end-to-end learning, which allows the model to directly learn early shapelets without the necessity of searching for numerous candidate shapelets. Third, an objective function of memory shapelet learning is proposed by overall considering accuracy and earliness, which can be optimized by gradient descent algorithm. Finally, experiments are conducted on benchmark dataset UCR, Tennessee Eastman process, and real-world aluminum electrolysis process in China. Comparable results with other state-of-the-art methods demonstrate the superior performance of the proposed method in interpretability, accuracy, earliness, and time complexity.
更多查看译文
关键词
Aluminum electrolysis,early classification,shapelet learning,time series,voltage signals
AI 理解论文
溯源树
样例
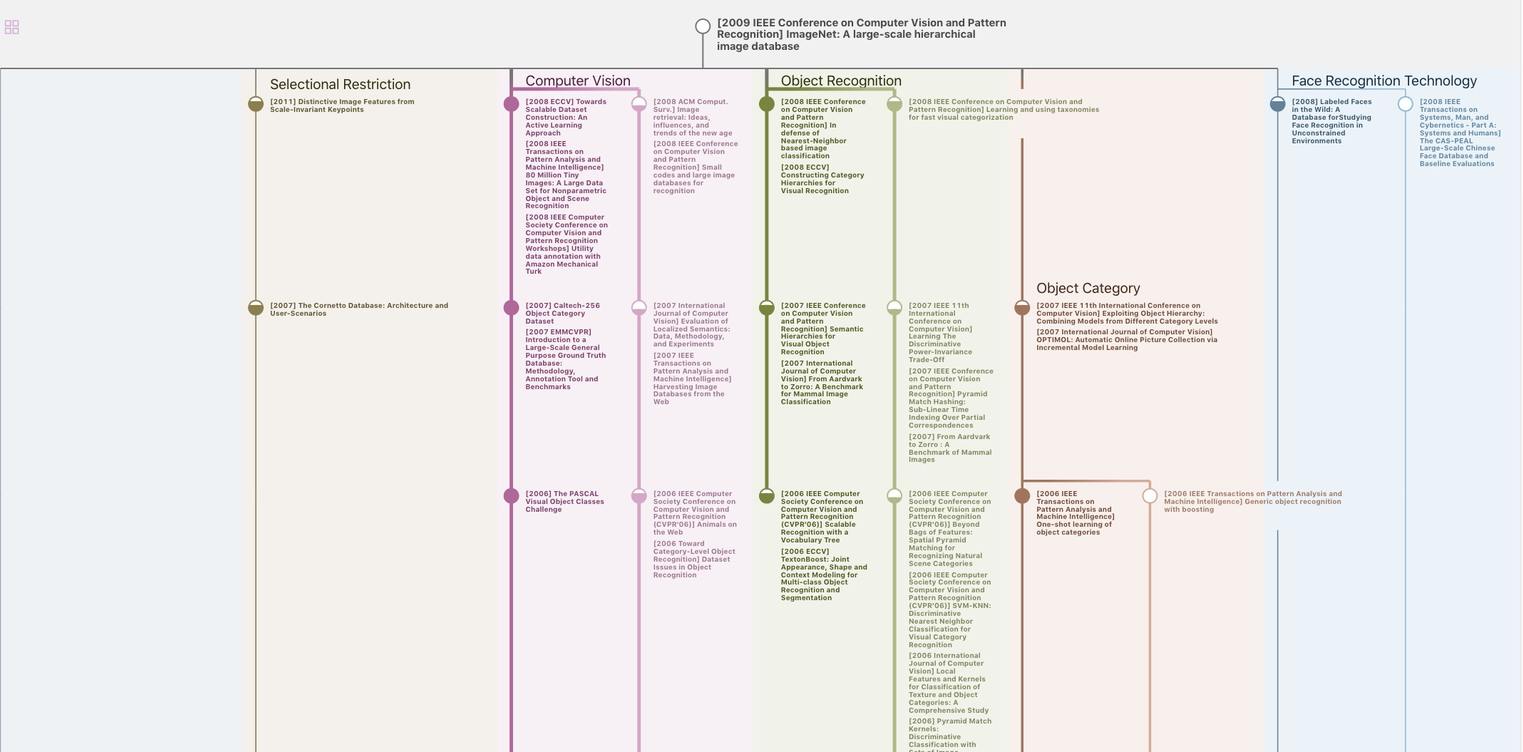
生成溯源树,研究论文发展脉络
Chat Paper
正在生成论文摘要