MDTL-ACP: Anticancer Peptides Prediction Based on Multi-Domain Transfer Learning.
IEEE journal of biomedical and health informatics(2023)
摘要
Anticancer peptides (ACPs) have emerged as one of the most promising therapeutic agents for cancer treatment. They are bioactive peptides featuring broad-spectrum activity and low drug-resistance. The discovery of ACPs via traditional biochemical methods is laborious and costly. Accordingly, various computational methods have been developed to facilitate the discovery of ACPs. However, the data resources and knowledge of ACPs are still very scarce, and only a few of them are clinically verified, which limits the competence of computational methods. To address this issue, in this paper, we propose an ACP prediction model based on multi-domain transfer learning, namely MDTL-ACP, to discriminate novel ACPs from plentiful inactive peptides. In particular, we collect abundant antimicrobial peptides (AMPs) from four well-studied peptide domains and extract their inherent features as the input of MDTL-ACP. The features learned from multiple source domains of AMPs are then transferred into the target prediction task of ACPs via artificial neural network-based shared-extractor and task-specific classifiers in MDTL-ACP. The knowledge captured in the transferred features enhances the prediction of ACPs in the target domain. Experimental results demonstrate that MDTL-ACP can outperform the traditional and state-of-the-art ACP prediction methods. The source code of MDTL-ACP and the data used in this study are available at https://github.com/JunhangCao/MTL-ACP.
更多查看译文
关键词
Anticancer peptide,antimicrobial peptide,transfer learning,deep learning,interpretability
AI 理解论文
溯源树
样例
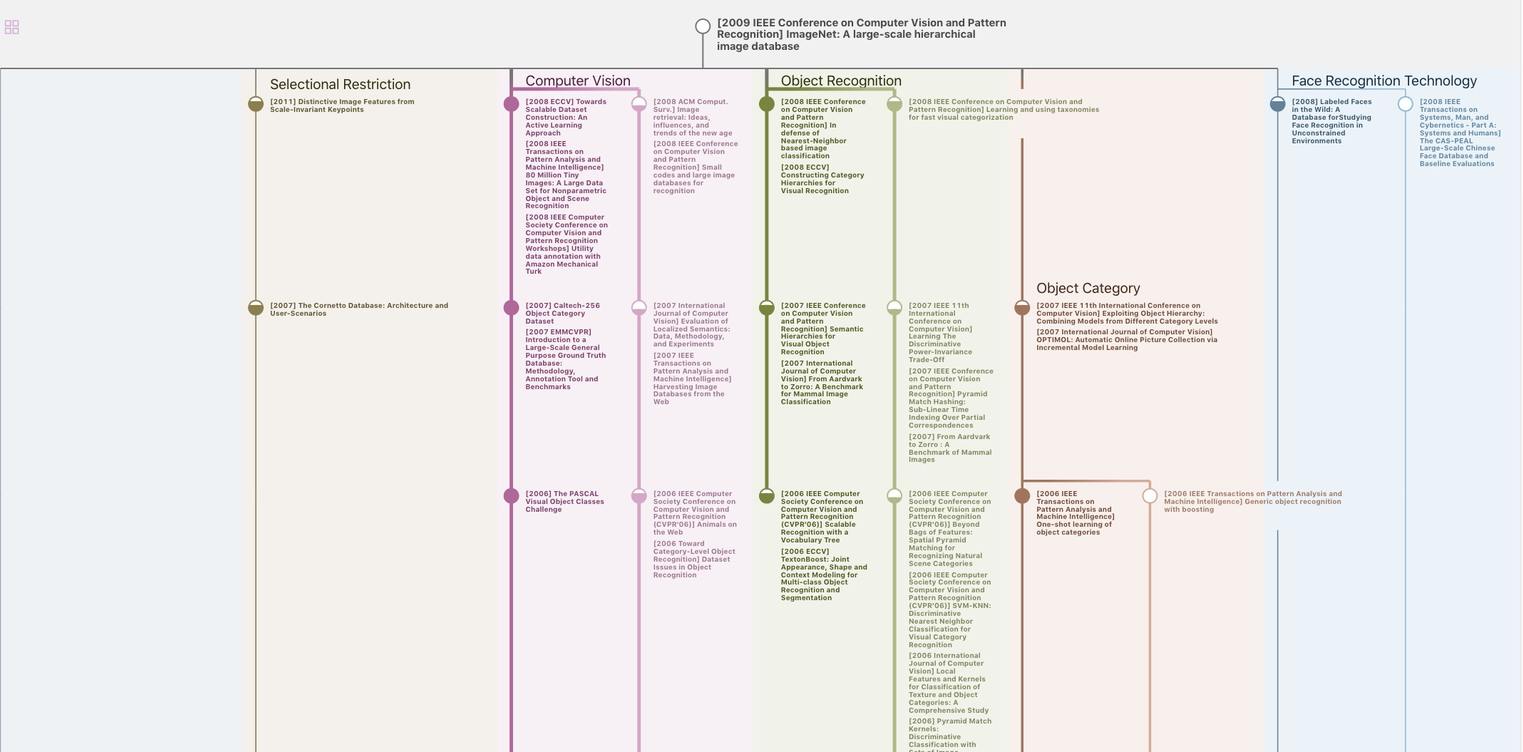
生成溯源树,研究论文发展脉络
Chat Paper
正在生成论文摘要