Unsupervised harmonization of brain MRI using 3D CycleGANs and its effect on brain age prediction
2023 19TH INTERNATIONAL SYMPOSIUM ON MEDICAL INFORMATION PROCESSING AND ANALYSIS, SIPAIM(2023)
摘要
Deep learning methods trained on brain MRI data from one scanner or imaging protocol can fail catastrophically when tested on data from other sites or protocols - a problem known as domain shift. To address this, here we propose a domain adaptation method that trains a 3D CycleGAN (cycle-consistent generative adversarial network) to harmonize brain MRI data from 5 diverse sources (ADNI, WHIMS, OASIS, AIBL, and the UK Biobank - a total of N=4,941 MRIs, age range: 46-96 years). The approach uses 2 generators and 2 discriminators to generate an image harmonized to a specific target dataset given an image from the source domain distribution and vice versa. We train the CycleGAN to jointly optimize an adversarial loss and cyclic consistency. We use a patch-based discriminator and impose identity loss to further regularize model training. To test the benefit of the harmonization, we show that brain age estimation - a common benchmarking task - is more accurate in GAN-harmonized versus raw data. t-SNE maps show the improved distributional overlap of the harmonized data in the latent space.
更多查看译文
AI 理解论文
溯源树
样例
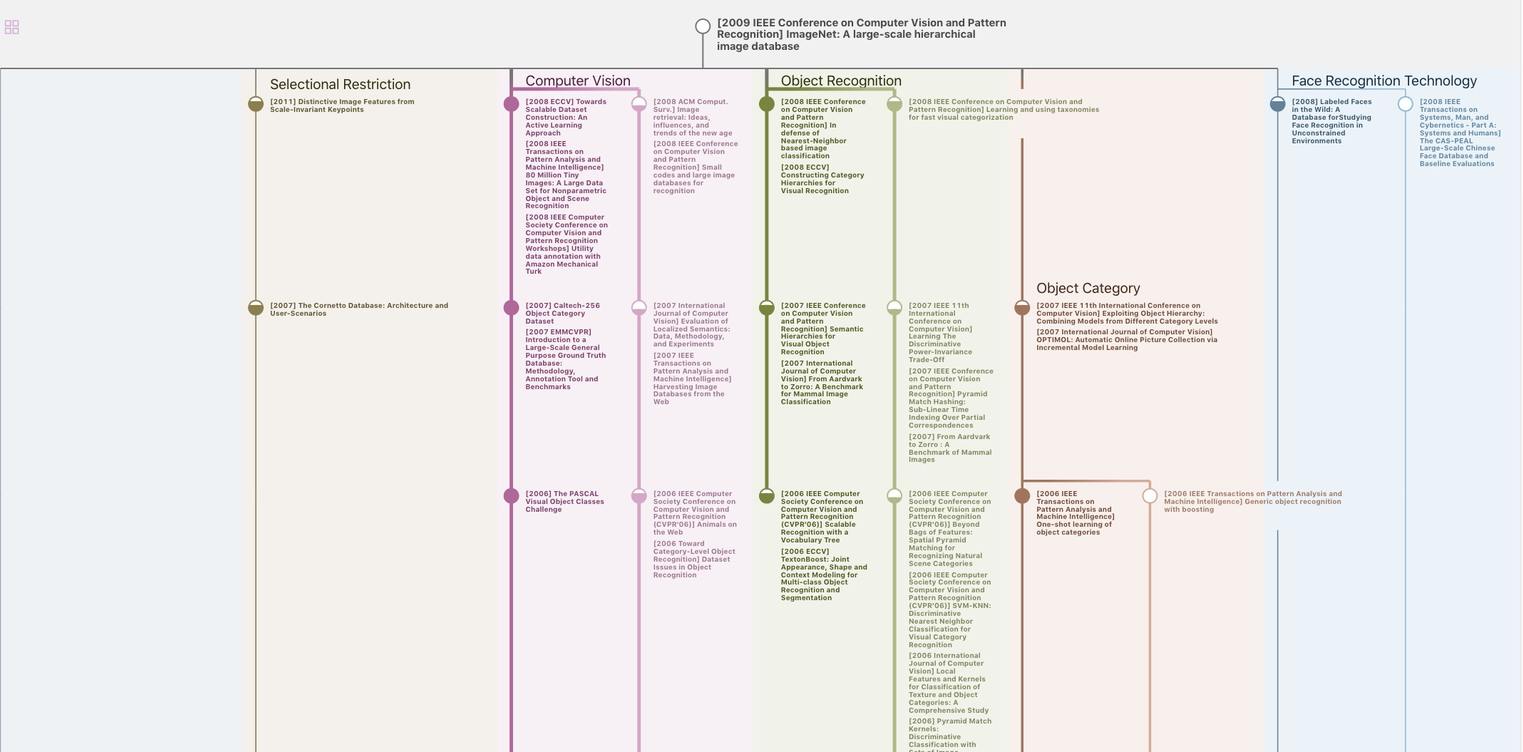
生成溯源树,研究论文发展脉络
Chat Paper
正在生成论文摘要