A comparative analysis between two convolutional networks architectures for semantic segmentation of histopathology breast cancer images.
2023 19th International Symposium on Medical Information Processing and Analysis (SIPAIM)(2023)
摘要
This study presents a comparative analysis efficacy between Fully Convolutional Networks (FCN) and U-Net architectures for semantic segmentation of tissues in histopathology breast cancer images. The imperative nature of precise and reliable image segmentation within the realm of computational pathology is highlighted, given its pivotal role in automating tasks involving structure detection within Whole-Slide Images (WSIs). Leveraging a publicly available dataset of breast cancer histopathology images from the Breast Cancer Semantic Segmentation (BCSS) challenge, the research evaluates the performance of FCN and U-Net models. The experimental results show that the best model was U-Net, under a binary cross entropy training, which achieves a performance among tissue classes (tumor and stroma) for semantic segmentation in terms of average accuracy of 0.8162, a Dice score of 0.6785 and Jaccard index of 0.5140. In contrast, FCN achieves an average accuracy of 0.7556, a Dice score of 0.6625 and a Jaccard index of 0.4956. Thus, the U-Net’s superior segmentation performance is evident, wherein its predicted masks exhibit a noticeable resemblance to actual segmented masks for both tumor and stroma classes.
更多查看译文
关键词
Computational pathology,semantic segmentation,breast cancer,FCN,U-Net,histopathology
AI 理解论文
溯源树
样例
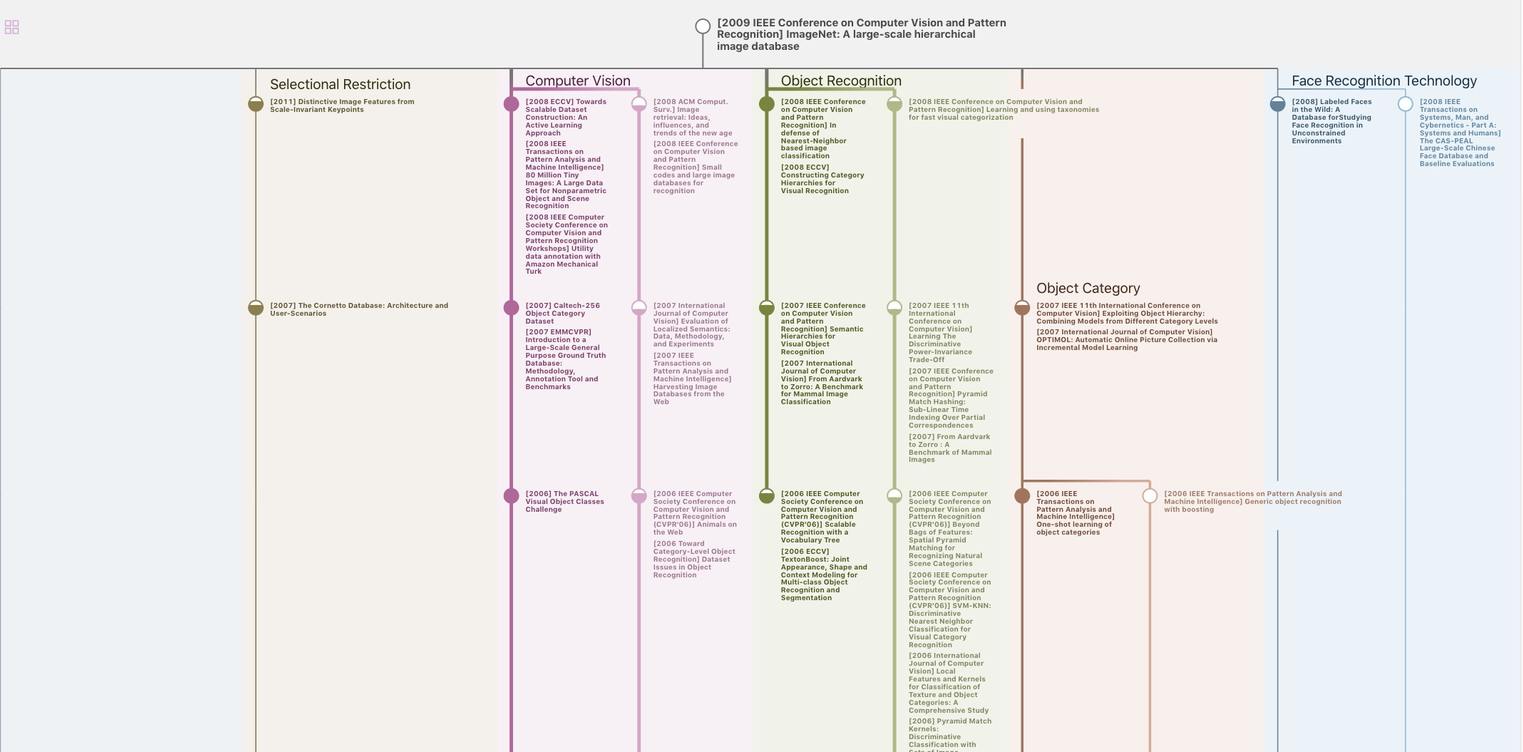
生成溯源树,研究论文发展脉络
Chat Paper
正在生成论文摘要