A Fault Diagnosis Method for Rolling Bearing Based on Deep Adversarial Transfer Learning With Transferability Measurement
IEEE SENSORS JOURNAL(2024)
摘要
The data distribution of rolling bearings varies under different operating conditions, and it is difficult to obtain a large amount of labeled data. Most existing work focuses solely on domain alignment and neglects the assessment of cross-domain transferability. In this article, a fault diagnosis method for rolling bearing based on deep adversarial transfer learning with transferability measurement (DATLTM) is proposed. First, the source domain and target domain data under different working conditions of rolling bearings are divided by empirical wavelet transform (EWT) and input the source domain and its subband data together with the target domain data into the deep neural network to generate a pretrained model, where the network is composed of a feature extraction module constructed by 1-D convolutional neural network (CNN) and a domain adaptive module that uses entropy regularized Wasserstein distance (ERWD) to measure the distribution difference. Subsequently, transferability measurement is conducted based on the logarithm of maximum evidence (LogME) evaluation index and the existing pretrained models. Target domain sample reconstruction is performed, and the source domain data are reintroduced into the network for training. The effectiveness and advantages of the proposed method were demonstrated through variable operating conditions tasks on Case Western Reserve University (CWRU) and self-conducted bearing fault datasets. Among them, on the six transfer tasks of self-conducted bearing fault datasets, compared with other transfer learning diagnosis methods, the proposed method has the highest cross-domain diagnosis accuracy in five tasks.
更多查看译文
关键词
Feature extraction,Training,Transfer learning,Rolling bearings,Entropy,Data models,Adaptation models,Deep adversarial transfer learning,empirical wavelet transform (EWT),entropy regularized Wasserstein distance (ERWD),rolling bearing,transferability measurement
AI 理解论文
溯源树
样例
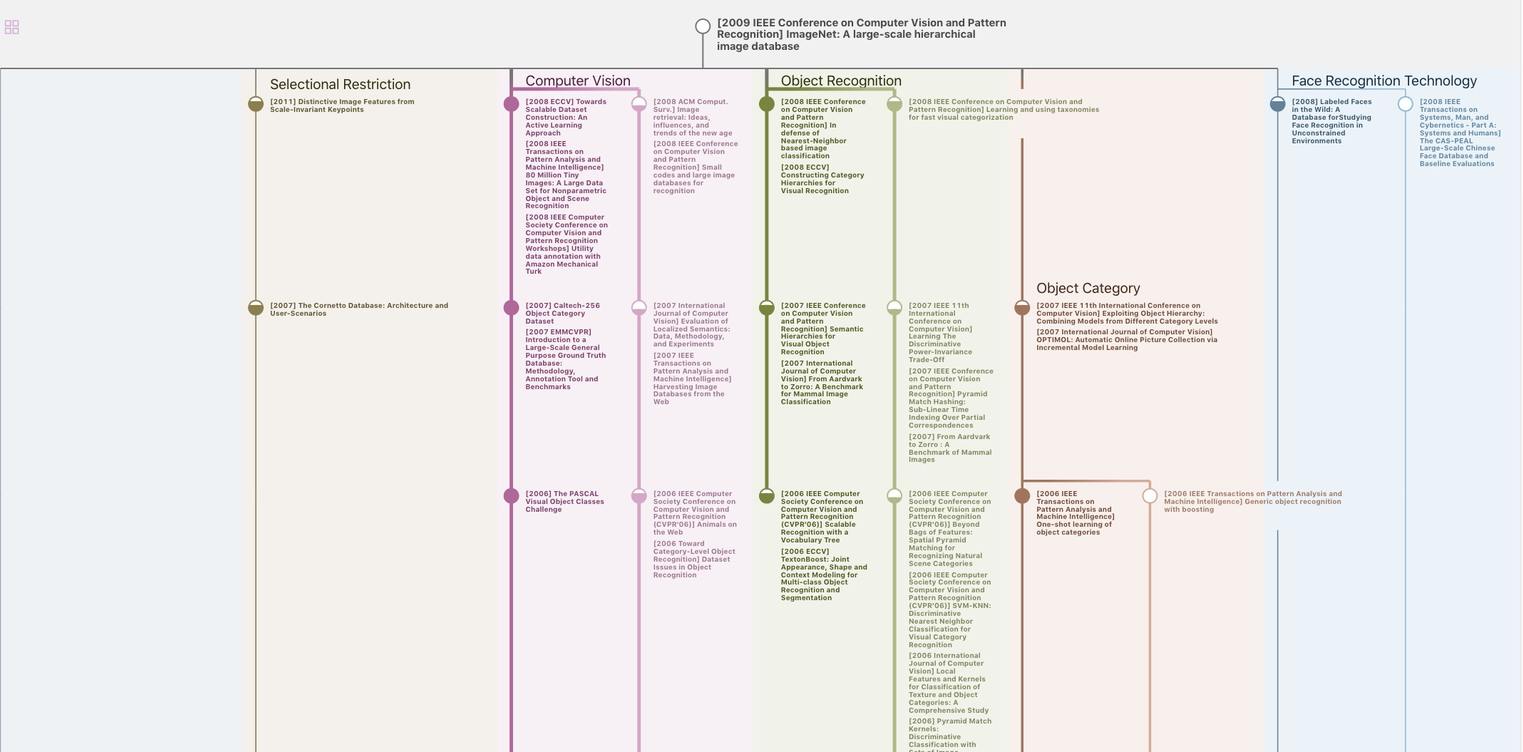
生成溯源树,研究论文发展脉络
Chat Paper
正在生成论文摘要