Side-Channel Attacks Based on Multi-Loss Regularized Denoising AutoEncoder
IEEE TRANSACTIONS ON INFORMATION FORENSICS AND SECURITY(2024)
摘要
Recently, researchers have leveraged the Denoising AutoEncoder (DAE) to reduce the noise in side-channel acquisitions (a.k.a. traces) that reduces the effectiveness of key recovery. Taking the ${L}2$ Loss (Mean Square Error, MSE) as the objective function of the DAE, it only aims to lessen the Euclidean Distance (ED) between the input and output, overlooking the Intra-Data Correlation (IDC) of the trace which includes the timing information. This paper proposes the Multi-Loss Regularized Denoising AutoEncoder (ML-DAE) framework to improve the generalization capability of the DAE. This framework consists of a shared DAE and Multiple Loss (ML) functions that aim to reduce the noise while preserving the excellent IDC of the output. During the training phase, to avoid issues of overfitting and a high number of training parameters, we pre-train the DAE using MSE and then initiate the ML-DAE which contains a multicore Partial Loss (PL) function with parameters transferred from the pre-trained DAE. During the testing phase, the outputs from the multicore PL are fused using an average pooling layer to yield the final predictions. The experiments on highly noisy datasets (XMEGA_ME, DPA_V2, and AES_GPU) and the masked dataset ASCAD demonstrate that ML-DAE achieves an SNR gain of at least four times, hence Deep-Learning based Side-Channel Attacks (DLSCAs) and Template Attacks (TA) with denoising pre-processing reduce of the number of traces needed to recover the key in the attack phase by more than 55%.
更多查看译文
关键词
Deep-learning,side-channel attacks,autoencoder,multi-loss regularized,intra-data correlation
AI 理解论文
溯源树
样例
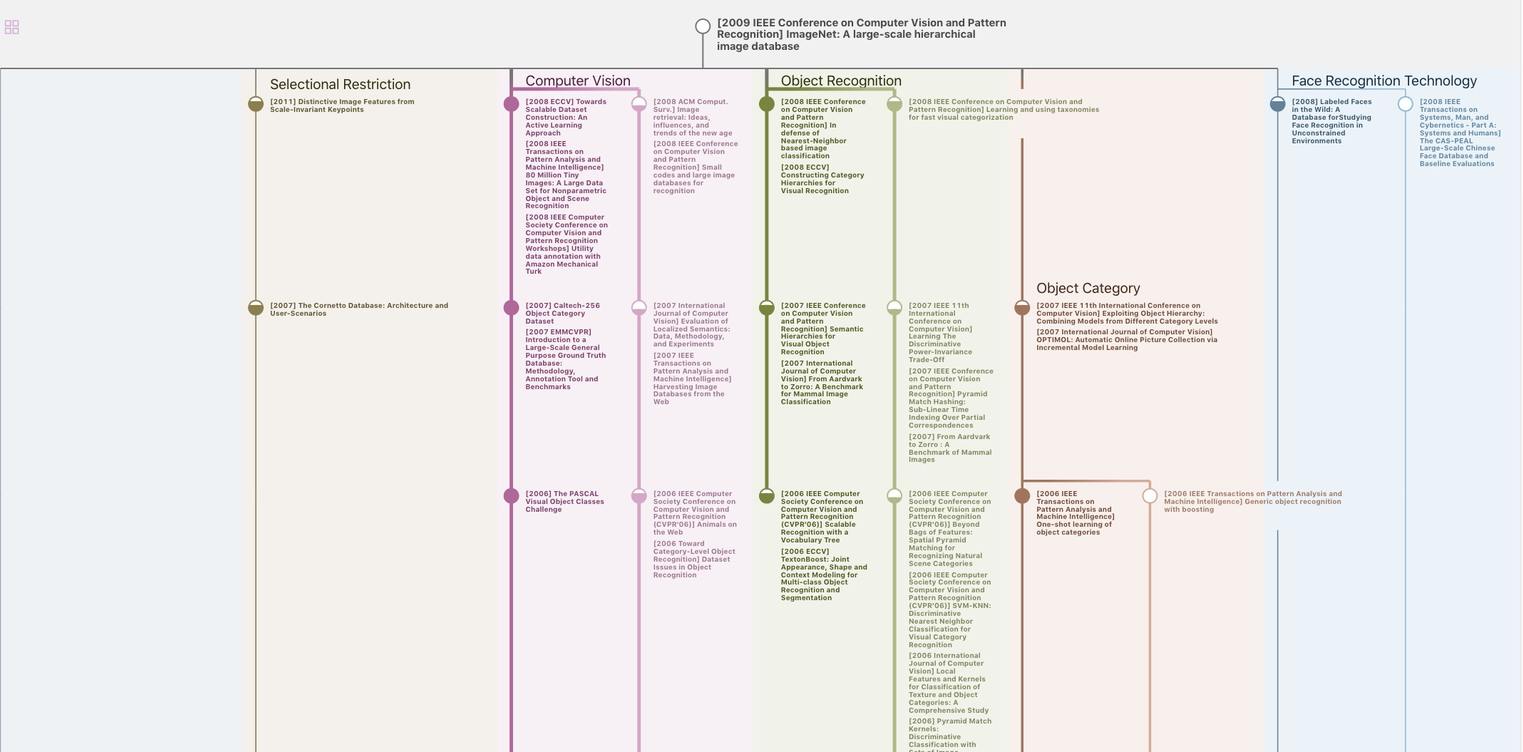
生成溯源树,研究论文发展脉络
Chat Paper
正在生成论文摘要