Finger Knuckle Print Classification Using Pretrained Vision Models
2023 IEEE 20th International Conference on Smart Communities: Improving Quality of Life using AI, Robotics and IoT (HONET)(2023)
摘要
Privacy and security are significant issues in the field of biometric traits in today's world. This research paper presents a comprehensive study that utilizes seven different deep learning models to classify Finger Knuckle Prints (FKP). The main aim of this study is to examine the efficacy of fine-tuning pretrained vision models in adapting to the specific dataset being analyzed. The models employed in this study include AlexNet, DensNet, EfficientNet, GoogleNet, Shallow Convolutional Neural Networks (SCNNs), ResNet50, and VisionTransformer. The models underwent training and testing procedures utilizing a comprehensive dataset obtained from 165 volunteers by Hong Kong Polytechnic University (Poly U). This dataset consisted of about 7,920 photos depicting the FKP gestures. A series of experiments were done to investigate the impact of alterations to the architectural design parameters of the models on the achievement of optimal recognition accuracy. The findings from our investigation indicate that the SCNNs and AlexNet had remarkably high accuracy rates of 98.3% and 96.224%, outperformed all other models. The accuracy rates reached by different models are as follows: EfficientNet achieved an accuracy rate of 98.176%, AlexNet achieved 96.224%, GoogleNet attained 95.601 %, ResNet50 achieved 92.598%, DenseNet achieved 81.224%, and VisionTransformer gave the lowest accuracy of 79.513%.
更多查看译文
关键词
Finger Knuckle Print,Vision Models,AlexNet,DensNet,EfficientNet,GoogleNet,shallow Convolutional Neural Network,ResNet50,VisionTransformer
AI 理解论文
溯源树
样例
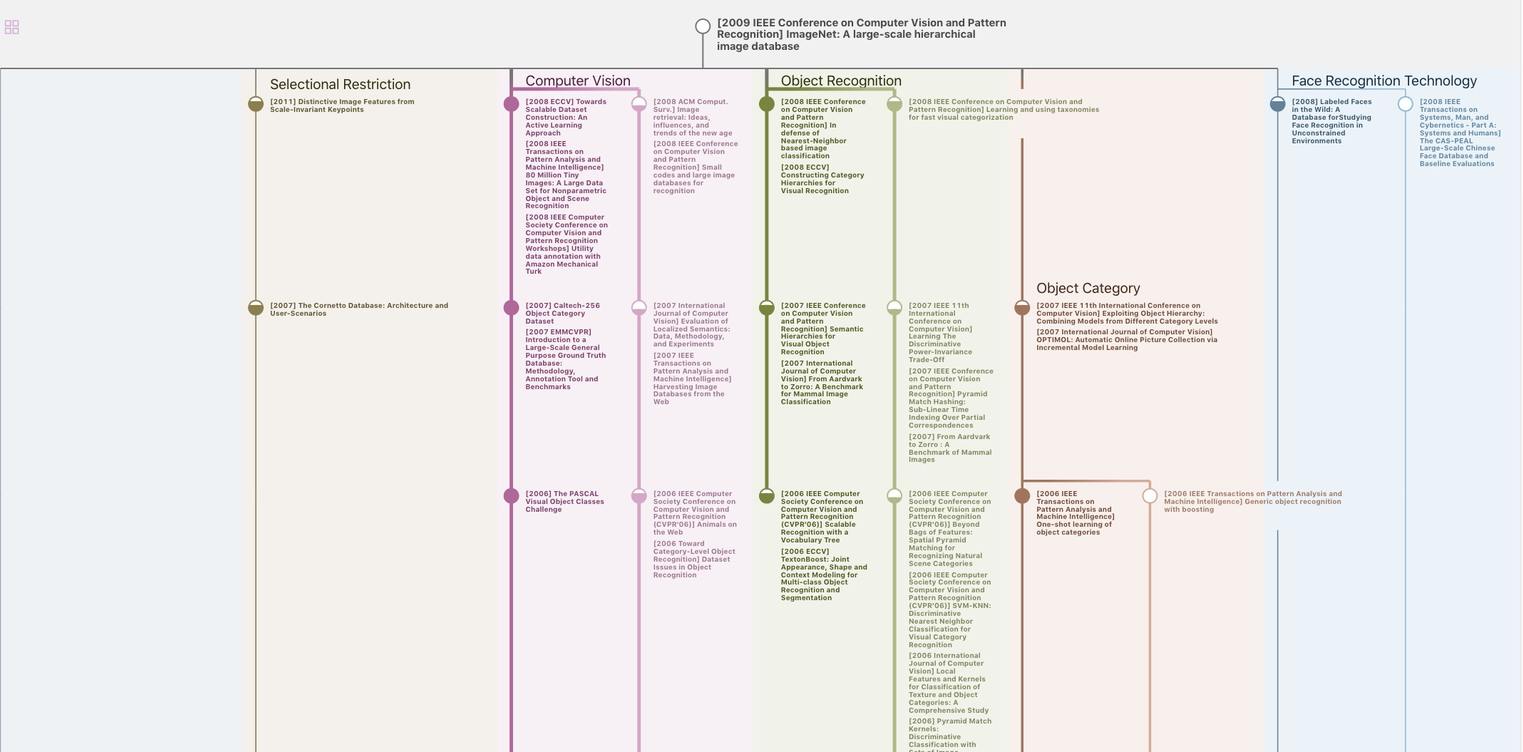
生成溯源树,研究论文发展脉络
Chat Paper
正在生成论文摘要