Opinion Classifier Transfer Learning from Review Data.
2023 IEEE Symposium Series on Computational Intelligence (SSCI)(2023)
Abstract
Companies use users' opinions to improve their products and marketing activities. In recent years, the development of Internet technology has made it possible to extract users' opinions from text on the Web. There are many ways for users to post their opinions on the Internet, and Twitter is considered to be a platform that allows users to easily tweet their opinions. However, manually extracting opinions from Twitter is time-consuming, costly, and labor-intensive due to the relatively low percentage of opinions. Therefore, some companies aim to efficiently extract user opinions from Twitter using machine learning. However, the attempt to create a dataset for building a machine learning system produced an unbalanced dataset that does not extract opinions with sufficient accuracy because the proportion of views on Twitter is small. There are solutions to this problem of insufficient teacher data, such as utilizing knowledge from other domains through transfer learning. Although transfer learning is sometimes used to solve such problems, accuracy cannot be improved if the knowledge domains are far apart before and after the transfer. Therefore, we proposed a new method called OTR, which stands for Opinion classifier Transferred from Review data. OTR transfers knowledge of review submissions that are considered to be close in domain to opinion extraction. However, since the phrasing of review sentences and that of Social Networking Service (SNS) such as Twitter are different, there is a possibility that sufficient knowledge transfer cannot be achieved. In order to address this problem, we proposed an Opinion classifier Transferred from Review data with Pseudo-labels (OTR-P), a method that brings the domains of the source and target tasks closer. Here, the target task discriminated opinions regarding leisure facilities, and the source task estimated review ratings using Rakuten travel review data. And while performing these tasks, we attempted to bring the domains closer by attaching pseudo-labels to the tweet data. This approach improved accuracy compared to the conventional method of shifting Bidirectional Encoder Representations from Transformers (BERT).
MoreTranslated text
Key words
Transfer Learning,Pseudo-labels,Opinion Mining,Domain Adaptation
AI Read Science
Must-Reading Tree
Example
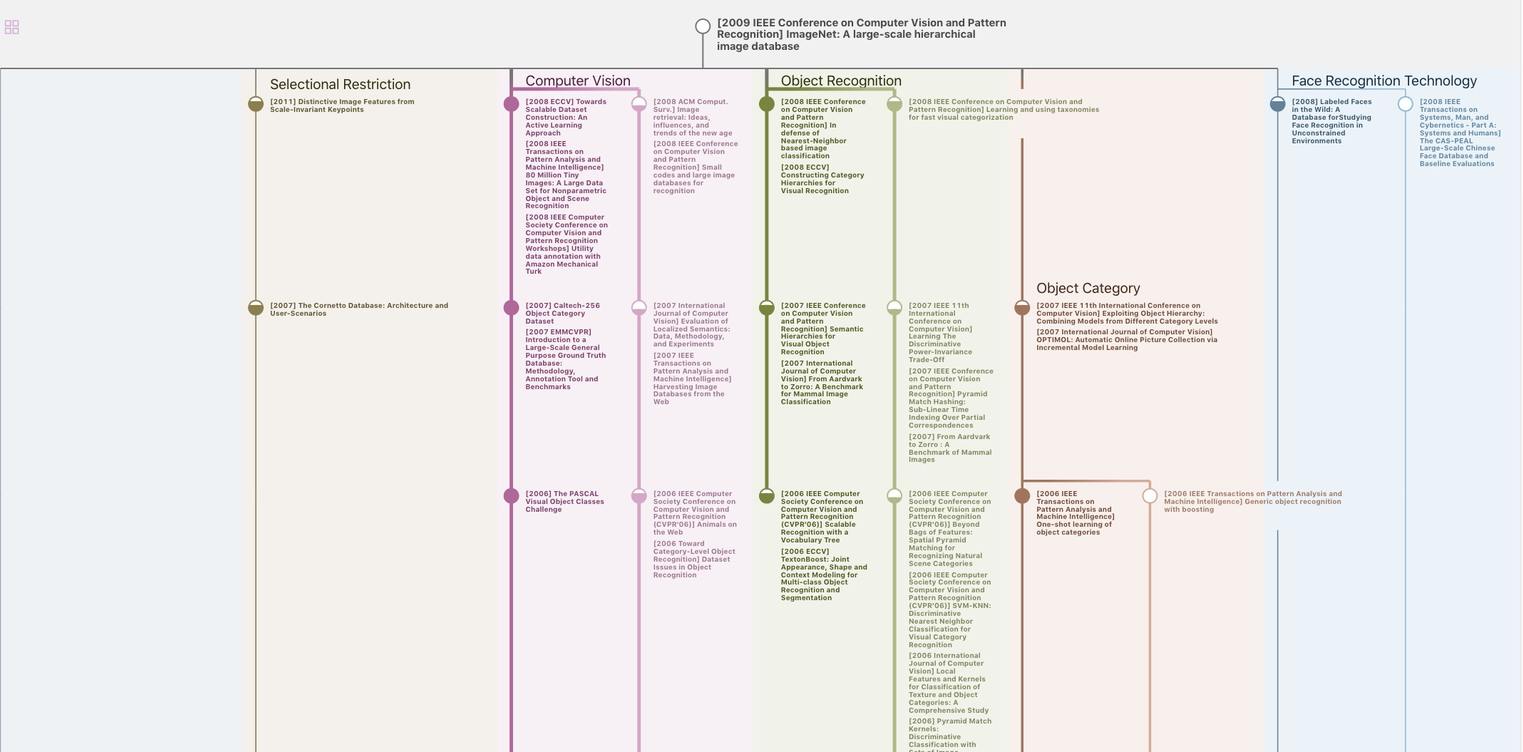
Generate MRT to find the research sequence of this paper
Chat Paper
Summary is being generated by the instructions you defined