A Weighted Ensemble of Regression Methods for Gross Error Identification Problem.
2023 IEEE Symposium Series on Computational Intelligence (SSCI)(2023)
摘要
In this study, we proposed a new ensemble method to predict the magnitude of gross errors (GEs) on measurement data obtained from the hydrocarbon and stream processing industries. Our proposed model consists of an ensemble of regressors (EoR) obtained by training different regression algorithms on the training data of measurements and their associated GEs. The predictions of the regressors are aggregated using a weighted combining method to obtain the final GE magnitude prediction. In order to search for optimal weights for combining, we modelled the search problem as an optimisation problem by minimising the difference between GE predictions and corresponding ground truths. We used Genetic Algorithm (GA) to search for the optimal weights associated with each regressor. The experiments were conducted on synthetic measurement data generated from 4 popular systems from the literature. We first conducted experiments in comparing the performances of the proposed ensemble using GA and Particle Swarm Optimisation (PSO), nature-based optimisation algorithms to search for combining weights to show the better performance of the proposed ensemble with GA. We then compared the performance of the proposed ensemble to those of two well-known weighted ensemble methods (Least Square and BEM) and two ensemble methods for regression problems (Random Forest and Gradient Boosting). The experimental results showed that although the proposed ensemble took higher computational time for the training process than those benchmark algorithms, it performed better than them on all experimental datasets.
更多查看译文
关键词
Gross error,ensemble method,regression,Genetic Algorithm,weighted ensemble
AI 理解论文
溯源树
样例
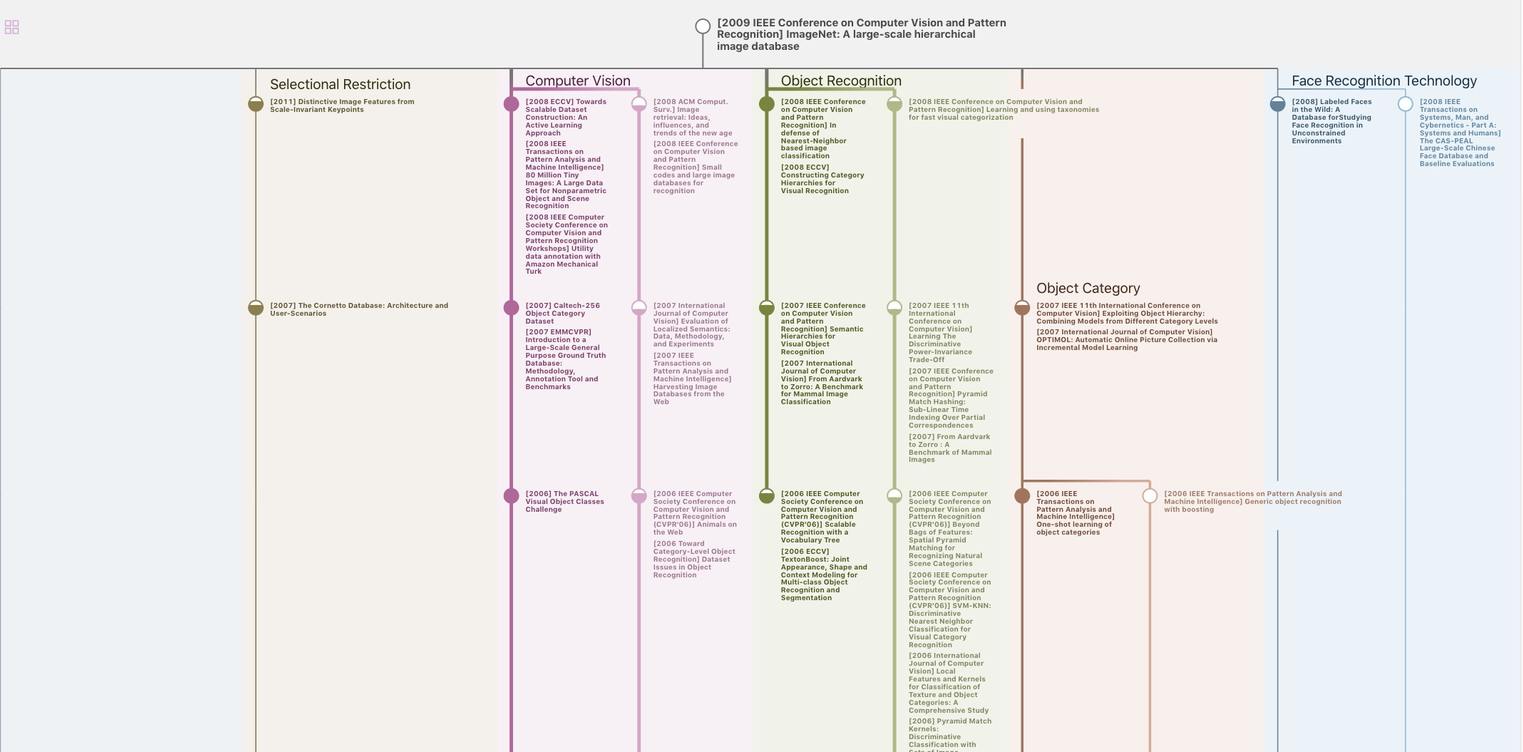
生成溯源树,研究论文发展脉络
Chat Paper
正在生成论文摘要