An Actor-Critic Architecture for Community Detection Ablation Study.
2023 IEEE Symposium Series on Computational Intelligence (SSCI)(2023)
摘要
This article conducts an ablation study of the Actor-Critic Architecture for Community Detection (AC2CD). The AC2CD uses Deep Reinforcement Learning (DRL) and Graph Attention Networks (GAT). Our ablation study method adheres to the principles of explainable artificial intelligence, focusing on assessing performance factors, including execution time, memory usage, and GPU utilization. We carried out experiments using two real-world datasets: Email-Eu-Core (EC), an email network among members of a European research institution (comprising 1,005 nodes, 25,571 edges, and 42 communities) available through the Stanford Snap Project, and a High School contact and friendship network (HS) in Marseilles, France, from December 2013 (comprising 329 nodes, 45,047 edges, and nine communities), obtainable from the Socio Patterns Website. We evaluated performance while considering three hyperparameters: learn_rate (LR), batch_size (BS), and n_games (NG), varying them at 10%, 30%, 50%, and 70%. The LR of 70% yielded optimal results with execution time for both EC and HS datasets. Furthermore, a BS of 70% indicated an ideal balance between execution time, GPU usage, and memory consumption for the HS dataset.
更多查看译文
关键词
Ablation Study,AC2CD,Hyperparameters
AI 理解论文
溯源树
样例
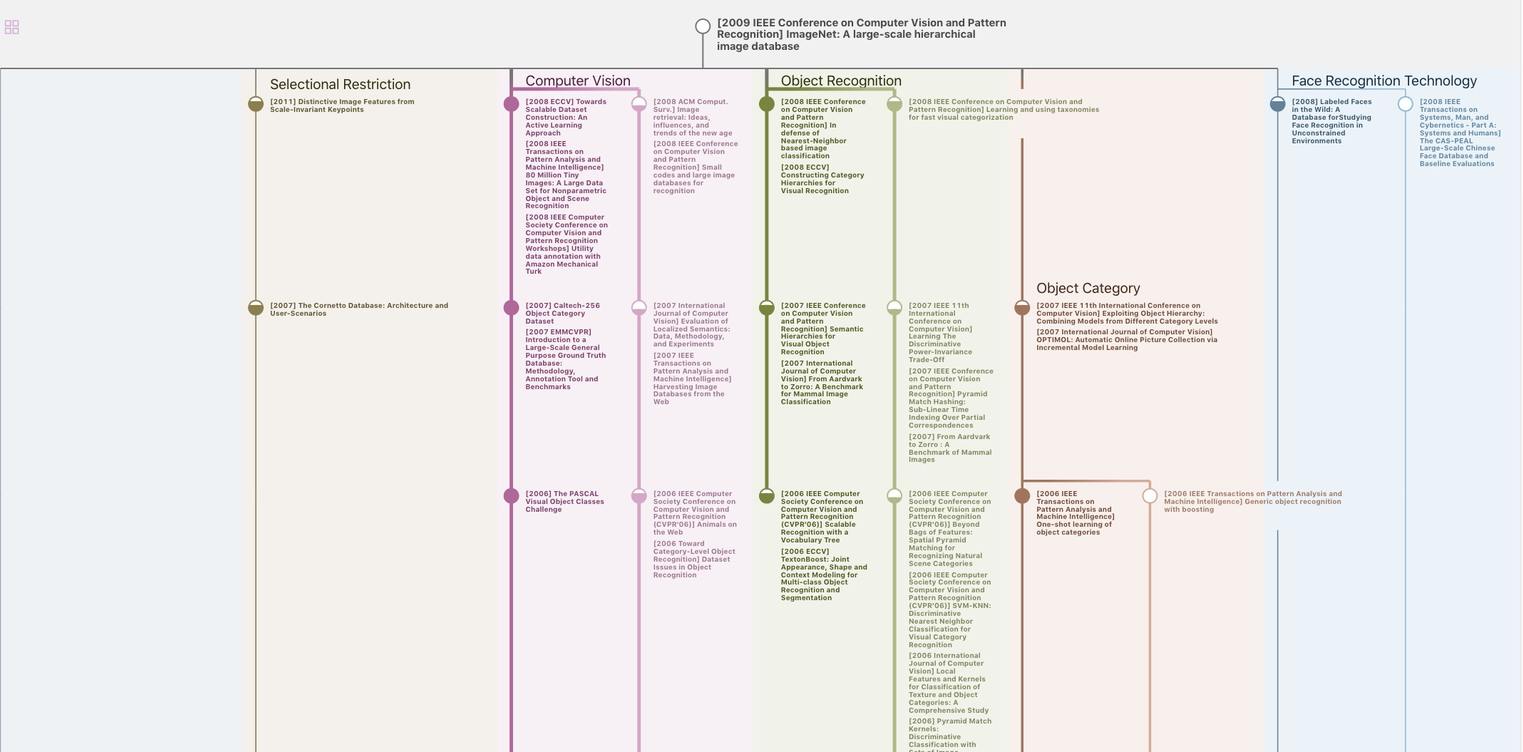
生成溯源树,研究论文发展脉络
Chat Paper
正在生成论文摘要