Analysis of Gait Data Based on Deep Learning to Predict Multiple Balance Scale Scores
IEEE SENSORS JOURNAL(2024)
摘要
Unbalanced walking is increasingly common among older adults; therefore, routinely assessing the balance of older adults is crucial. The traditional method of assessing balance uses scales, requires the supervision of a physical therapist (PT), and is time-consuming. The present study, therefore, proposes a deep learning (DL) model architecture that combines a convolutional neural network (CNN) and a long short-term memory (LSTM) network model to predict the scores of three scales, namely the Berg balance scale (BBS), timed up and go (TUG), and single-leg stance (SLS). The gait data of 15 m of walking were collected from seven inertial measurement units (IMUs) and input into the CNN-LSTM model for evaluation. The BBS and TUG only require the participants to wear two IMUs on the left and right thighs, respectively, for accurate predictions. The mean absolute errors (MAEs) of predicting the scores of the BBS and TUG are 1.2562 and 1.4016 s, respectively; however, the MAE of score predictions of SLS is higher than that of the BBS and TUG, indicating that gait data cannot be used for assessing SLS; moreover, participants only wear one IMU on the right calf for BBS and TUG evaluations, which yield MAEs of 1.4334 and 1.5229 s, respectively. The proposed system can quickly and accurately predict the scores of the BBS and TUG. The proposed model can assist PTs with making clinical decisions.
更多查看译文
关键词
Berg balance scale (BBS),deep learning (DL),gait,single-leg stance (SLS),timed up and go (TUG)
AI 理解论文
溯源树
样例
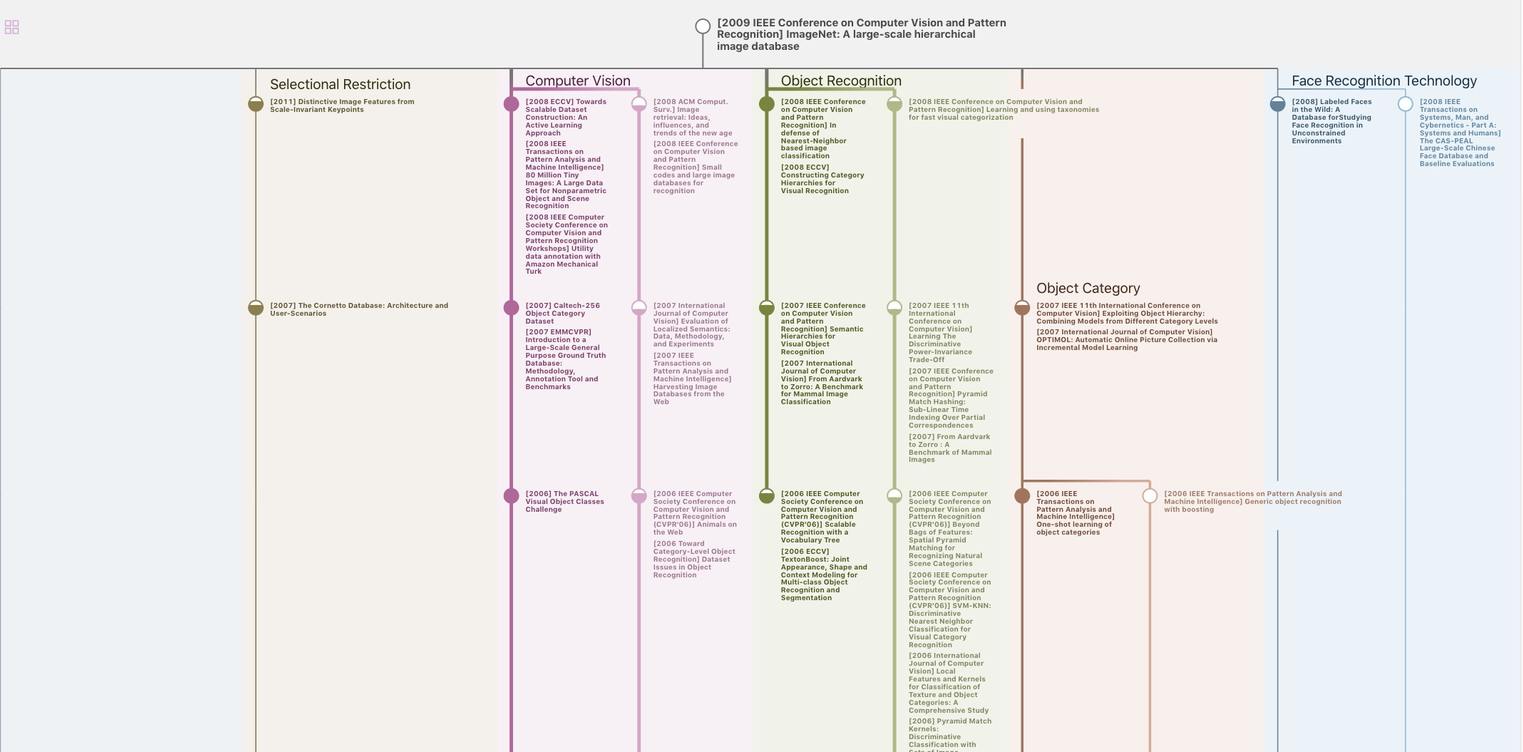
生成溯源树,研究论文发展脉络
Chat Paper
正在生成论文摘要