An Adaptive Local Context Extraction Method for Motion Prediction and Planning.
2023 IEEE International Conference on Cybernetics and Intelligent Systems (CIS) and IEEE Conference on Robotics, Automation and Mechatronics (RAM)(2023)
摘要
Learning-based motion prediction and planning algorithms can efficiently extract and effectively represent the agent-map relationships in high-dimensional space. Generally, the performance is highly related to the neural network structure and quality of input data. However, existing works are more focused on exploring novel network structures while ignoring the form of data input. Typically, for a target agent to predict or plan, they simply input all road polylines within its R-meters radius as local context information and then use a network such as a cross-attention module to filter out the unimportant and irrelevant part of the local context. This simple strategy not only burdens the network in spatial and temporal computation consumption but also leads to much redundancy and overlapping when two target agents are very close. We propose our Adaptive Breadth-First Search (ABFS) local context extraction method to tackle these issues. ABFS inherits from breadth-first search (BFS), adding adaptive search depth based on the agent’s current state and changing the weights of the search direction (more focused on the roads forward). We compare our methods with previous radius search (RS) based and depth-first search (DFS) based context extraction methods. Systematic experiments are done based on the Differentiable Integrated Motion Prediction and Planning (DIPP) algorithm using Waymo motion dataset. We give both qualitative and quantitative results to show our method’s superiority.
更多查看译文
关键词
Autonomous driving,Adaptive Breadth First Search (AFBS),Motion Prediction and Planning
AI 理解论文
溯源树
样例
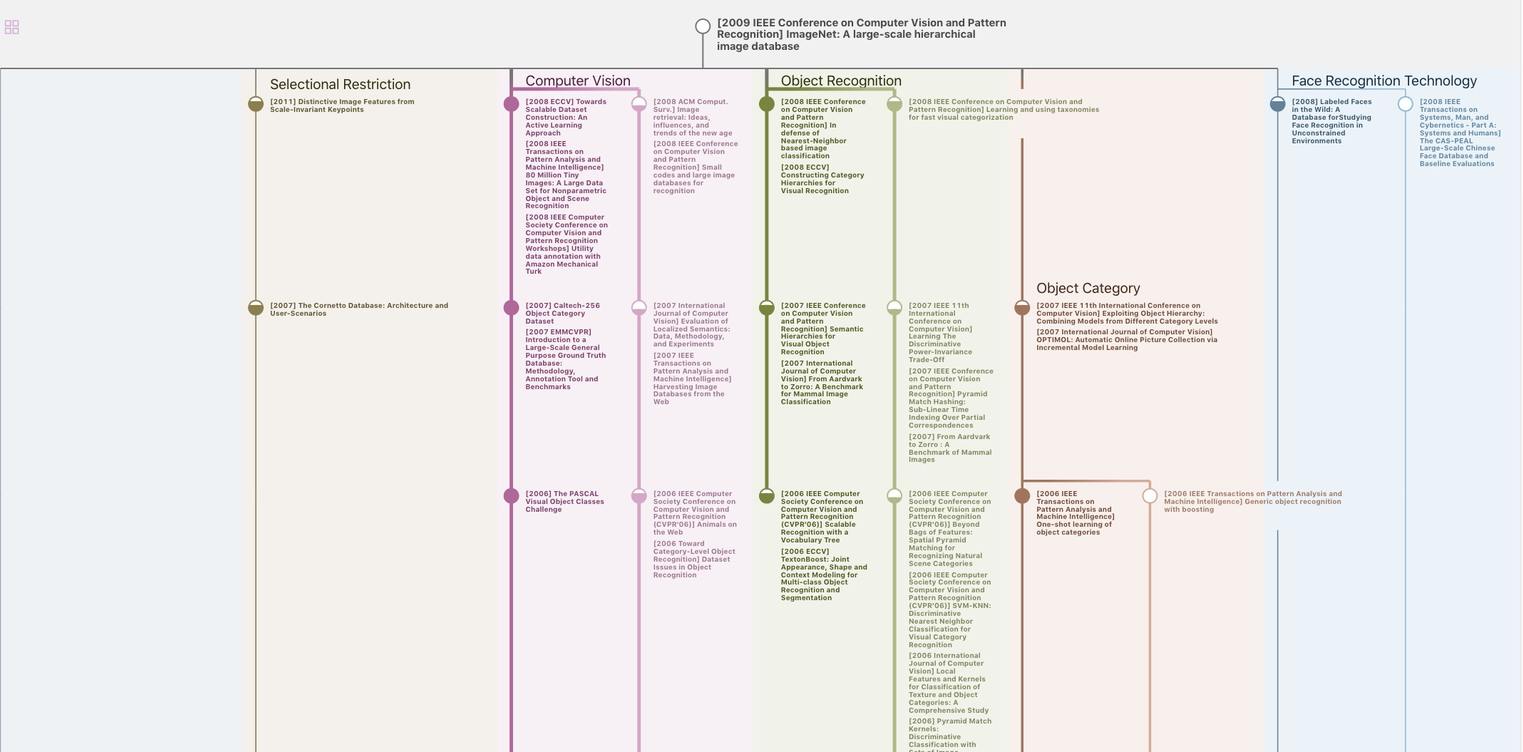
生成溯源树,研究论文发展脉络
Chat Paper
正在生成论文摘要