Frustration Recognition Using Spatio Temporal Data: A Novel Dataset and GCN Model to Recognize In-Vehicle Frustration
IEEE TRANSACTIONS ON AFFECTIVE COMPUTING(2023)
摘要
Frustration is an unpleasant emotion prevalent in several target applications of affective computing, such as human-machine interaction, learning, (online) customer interaction, and gaming. One idea to redeem this issue is to recognize frustration to offer help or mitigation in real-time, e.g., by a personal assistant. However, the recognition of frustration is not limited to these applied contexts but can also inform emotion research in general. This paper presents a dataset of 43 participants who experienced frustration in driving-related situations in a simulator. The data set contains a continuous subjective label, hand-annotated face and body expressions, facial landmark coordinates of two cameras, and the participants' age and sex information. In addition, a descriptive analysis and description of the data's characteristics are provided together with a Graph Convolution Network based model to recognize frustration. Allowing for a tolerance of 10%, the model could correctly identify frustration with a similarity of 79.4 % and a variance of 7.7 %. This work is valuable for researchers of the affective computing community because it provides realistic data with an in-depth description of its characteristics and a benchmark model for automated frustration recognition.
更多查看译文
关键词
Affect-aware systems,frustration recognition,graph convolution network,naturalistic dataset
AI 理解论文
溯源树
样例
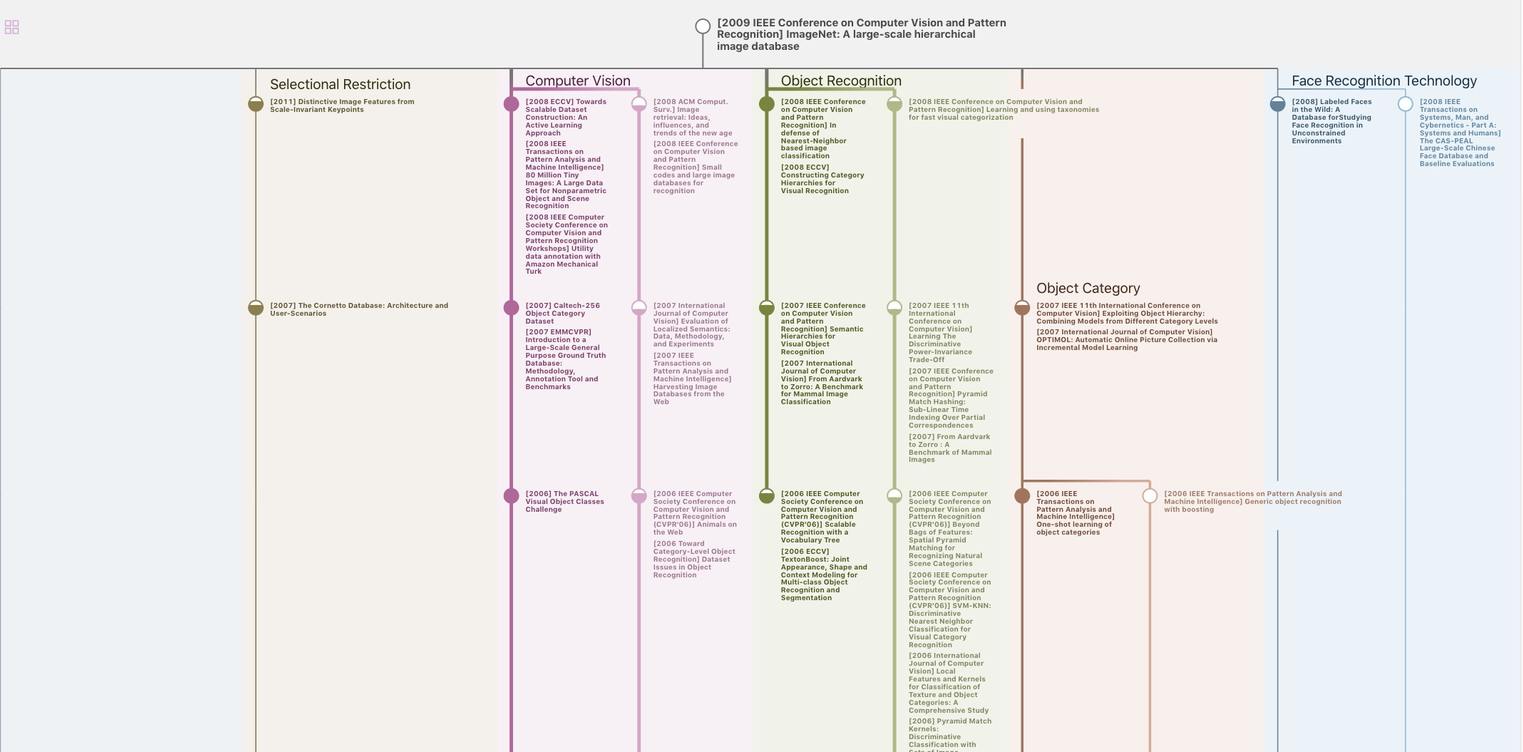
生成溯源树,研究论文发展脉络
Chat Paper
正在生成论文摘要