The Effect of Resampling on Classifier Performance: an Empirical Study.
Knowledge Engineering and Data Science(2022)
摘要
An imbalanced class on a dataset is a common classification problem. The effect of using imbalanced class datasets can cause a decrease in the performance of the classifier. Resampling is one of the solutions to this problem. This study used 100 datasets from 3 websites: UCI Machine Learning, Kaggle, and OpenML. Each dataset will go through 3 processing stages: the resampling process, the classification process, and the significance testing process between performance evaluation values of the combination of classifier and the resampling using paired t-test. The resampling used in the process is Random Undersampling, Random Oversampling, and SMOTE. The classifier used in the classification process is Naïve Bayes Classifier, Decision Tree, and Neural Network. The classification results in accuracy, precision, recall, and f-measure values are tested using paired t-tests to determine the significance of the classifier's performance from datasets that were not resampled and those that had applied the resampling. The paired t-test is also used to find a combination between the classifier and the resampling that gives significant results. This study obtained two results. The first result is that resampling on imbalanced class datasets can substantially affect the classifier's performance more than the classifier's performance from datasets that are not applied the resampling technique. The second result is that combining the Neural Network Algorithm without the resampling provides significance based on the accuracy value. Combining the Neural Network Algorithm with the SMOTE technique provides significant performance based on the amount of precision, recall, and f-measure.
更多查看译文
关键词
Imbalanced Data,Logistic Regression,Naive Bayes Classifier,Ensemble Methods,Cost-Sensitive Learning
AI 理解论文
溯源树
样例
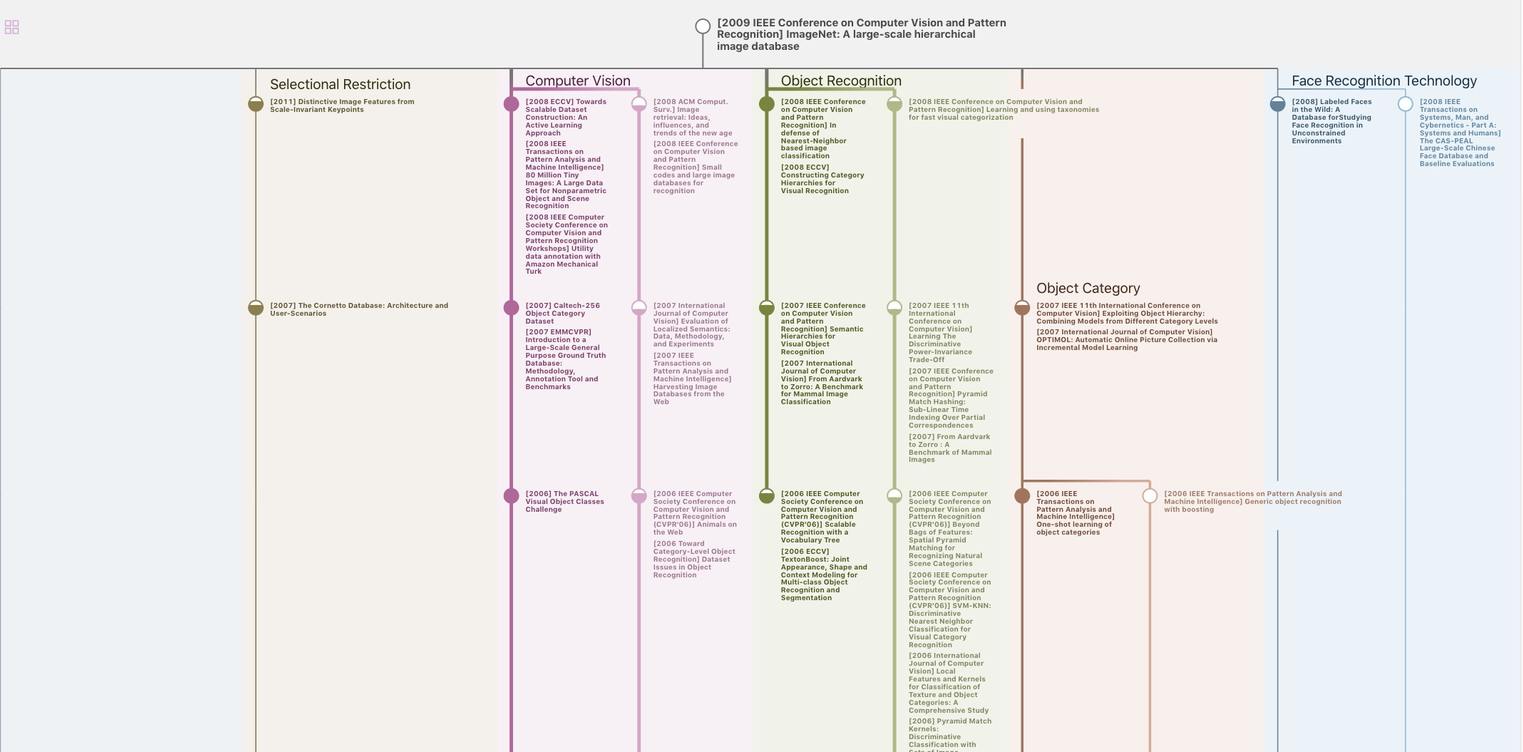
生成溯源树,研究论文发展脉络
Chat Paper
正在生成论文摘要