Enhancing dysarthria speech feature representation with empirical mode decomposition and Walsh-Hadamard transform
CoRR(2023)
摘要
Dysarthria speech contains the pathological characteristics of vocal tract
and vocal fold, but so far, they have not yet been included in traditional
acoustic feature sets. Moreover, the nonlinearity and non-stationarity of
speech have been ignored. In this paper, we propose a feature enhancement
algorithm for dysarthria speech called WHFEMD. It combines empirical mode
decomposition (EMD) and fast Walsh-Hadamard transform (FWHT) to enhance
features. With the proposed algorithm, the fast Fourier transform of the
dysarthria speech is first performed and then followed by EMD to get intrinsic
mode functions (IMFs). After that, FWHT is used to output new coefficients and
to extract statistical features based on IMFs, power spectral density, and
enhanced gammatone frequency cepstral coefficients. To evaluate the proposed
approach, we conducted experiments on two public pathological speech databases
including UA Speech and TORGO. The results show that our algorithm performed
better than traditional features in classification. We achieved improvements of
13.8
incorporation of an imbalanced classification algorithm to address data
imbalance has resulted in a 12.18
algorithm effectively addresses the challenges of the imbalanced dataset and
non-linearity in dysarthric speech and simultaneously provides a robust
representation of the local pathological features of the vocal folds and
tracts.
更多查看译文
AI 理解论文
溯源树
样例
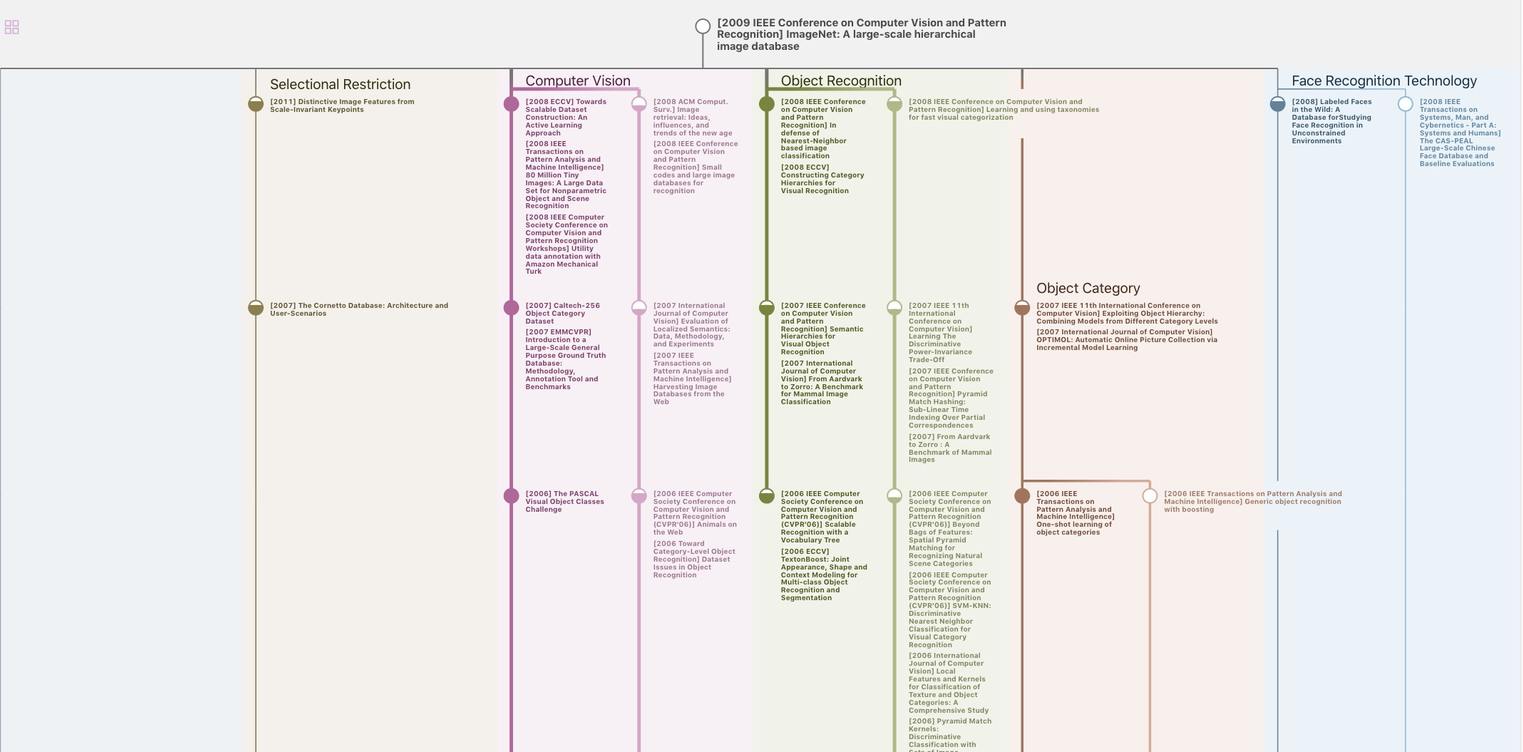
生成溯源树,研究论文发展脉络
Chat Paper
正在生成论文摘要