Pushing Boundaries: Exploring Zero Shot Object Classification with Large Multimodal Models
2023 Tenth International Conference on Social Networks Analysis, Management and Security (SNAMS)(2023)
Abstract
The synergy of language and vision models has given rise to Large Language
and Vision Assistant models (LLVAs), designed to engage users in rich
conversational experiences intertwined with image-based queries. These
comprehensive multimodal models seamlessly integrate vision encoders with Large
Language Models (LLMs), expanding their applications in general-purpose
language and visual comprehension. The advent of Large Multimodal Models (LMMs)
heralds a new era in Artificial Intelligence (AI) assistance, extending the
horizons of AI utilization. This paper takes a unique perspective on LMMs,
exploring their efficacy in performing image classification tasks using
tailored prompts designed for specific datasets. We also investigate the LLVAs
zero-shot learning capabilities. Our study includes a benchmarking analysis
across four diverse datasets: MNIST, Cats Vs. Dogs, Hymnoptera (Ants Vs. Bees),
and an unconventional dataset comprising Pox Vs. Non-Pox skin images. The
results of our experiments demonstrate the model's remarkable performance,
achieving classification accuracies of 85%, 100%, 77%, and 79% for the
respective datasets without any fine-tuning. To bolster our analysis, we assess
the model's performance post fine-tuning for specific tasks. In one instance,
fine-tuning is conducted over a dataset comprising images of faces of children
with and without autism. Prior to fine-tuning, the model demonstrated a test
accuracy of 55%, which significantly improved to 83% post fine-tuning. These
results, coupled with our prior findings, underscore the transformative
potential of LLVAs and their versatile applications in real-world scenarios.
MoreTranslated text
Key words
Large Language Models,Large Multimodal Models,Prompt Engineering,Classification
AI Read Science
Must-Reading Tree
Example
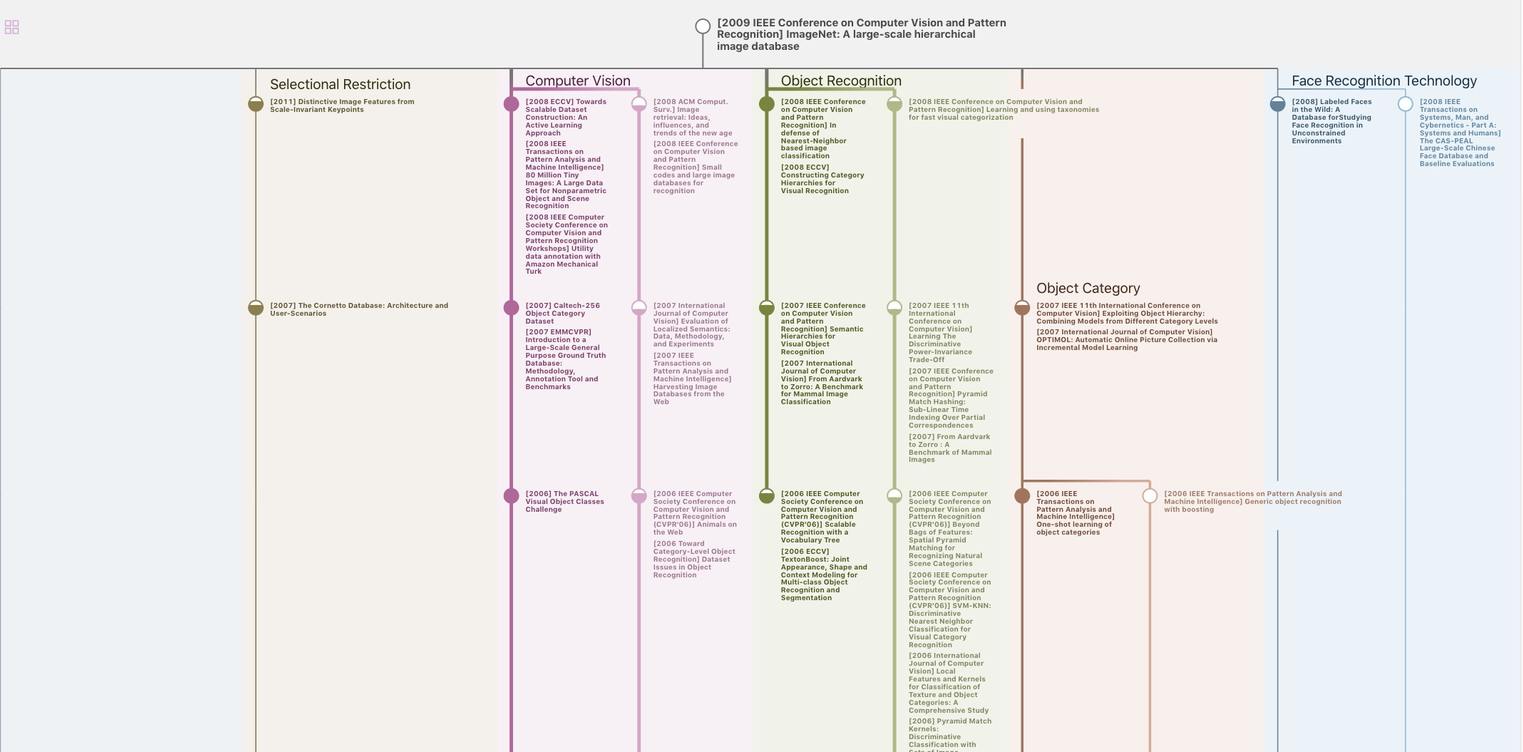
Generate MRT to find the research sequence of this paper
Chat Paper
Summary is being generated by the instructions you defined