A Large-Scale Re-identification Analysis in Sporting Scenarios: the Betrayal of Reaching a Critical Point
CoRR(2023)
摘要
Re-identifying participants in ultra-distance running competitions can be
daunting due to the extensive distances and constantly changing terrain. To
overcome these challenges, computer vision techniques have been developed to
analyze runners' faces, numbers on their bibs, and clothing. However, our study
presents a novel gait-based approach for runners' re-identification (re-ID) by
leveraging various pre-trained human action recognition (HAR) models and loss
functions. Our results show that this approach provides promising results for
re-identifying runners in ultra-distance competitions. Furthermore, we
investigate the significance of distinct human body movements when athletes are
approaching their endurance limits and their potential impact on re-ID
accuracy. Our study examines how the recognition of a runner's gait is affected
by a competition's critical point (CP), defined as a moment of severe fatigue
and the point where the finish line comes into view, just a few kilometers away
from this location. We aim to determine how this CP can improve the accuracy of
athlete re-ID. Our experimental results demonstrate that gait recognition can
be significantly enhanced (up to a 9
this point. This highlights the potential of utilizing gait recognition in
real-world scenarios, such as ultra-distance competitions or long-duration
surveillance tasks.
更多查看译文
关键词
Loss Function,Real-world Scenarios,Action Recognition,Finish Line,Human Activity Recognition,Model Performance,Convolutional Neural Network,Mass Media,Positive Samples,Feature Maps,Negative Samples,Large-scale Datasets,Video Clips,Video Frames,Average Precision,Optical Flow,Radio Frequency Identification,Batch Normalization Layer,Value Of Map,Triplet Loss,Pre-trained Encoder,Human Gait,Margin Parameter,Non-local Block,Percentage Of Matches,Re-identification Task,10-fold Cross-validation,Skeleton Data
AI 理解论文
溯源树
样例
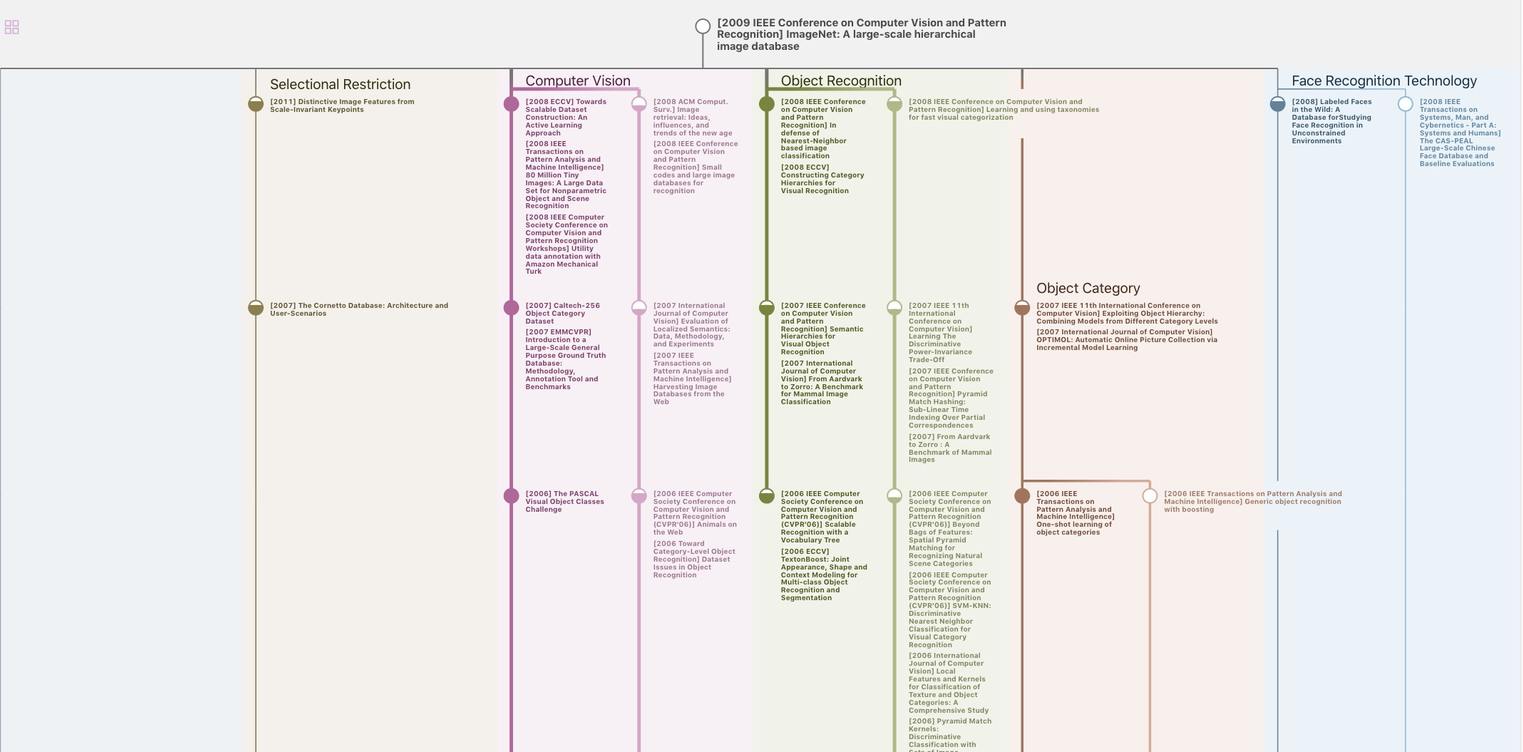
生成溯源树,研究论文发展脉络
Chat Paper
正在生成论文摘要