Exploiting classifier inter-level features for efficient out-of-distribution detection
IMAGE AND VISION COMPUTING(2024)
摘要
Deep learning approaches have achieved state-of-the-art performance in a wide range of applications. Most often, however, it is falsely assumed that samples at inference follow a similar distribution as the training data. This assumption impairs models' ability to handle Out-of-Distribution (OOD) data during deployment. While several OOD detection approaches mostly focus on outputs of the last layer, we propose a novel mechanism that exploits features extracted from intermediate layers of a deep classifier. Specifically, we train an off-the-shelf auxiliary network using features of early layers to learn distinctive representations that improve OOD detection. The proposed network can be appended to any classification model without imposing any modification to its original architecture. Additionally, the mechanism does not require access to OOD data during training. We evaluate the performance of the mechanism on a variety of backbone architectures and datasets for near-OOD and far-OOD scenarios. The results demonstrate improvements in OOD detection compared to other state-of-the-art approaches. In particular, our proposed mechanism improves AUROC by 14.2% and 8.3% in comparison to the strong OOD baseline method, and by 3.2% and 3.9% in comparison to the second-best performing approach, on CIFAR-10 and CIFAR-100 datasets respectively.
更多查看译文
关键词
Out -of -distribution detection,Deep learning -based classification,Machine learning,Feature exploitation,Intermediate feature extraction
AI 理解论文
溯源树
样例
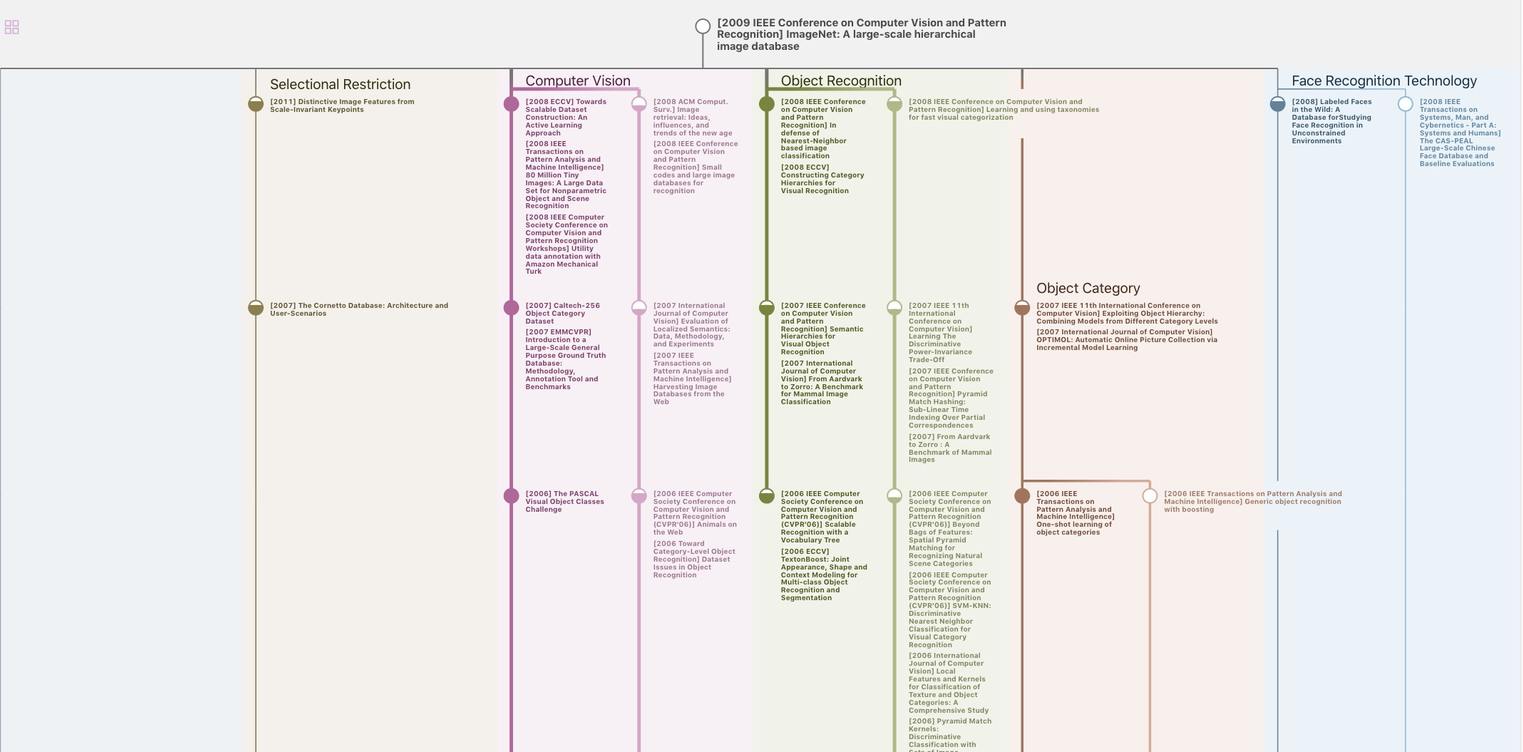
生成溯源树,研究论文发展脉络
Chat Paper
正在生成论文摘要