Spectroscopy data calibration using stacked ensemble machine learning
IIUM ENGINEERING JOURNAL(2024)
摘要
Near infrared spectroscopy (NIRS) is a widely used analytical technique for non-destructive analysis of various materials including food fraud detection. However, the accurate calibration of NIRS data can be challenging due to the complexity of the underlying relationships between the spectral data and the target variables of interest. Ensemble learning, which combines multiple models to make predictions, has been shown to improve the accuracy and robustness of predictive models in various domains. This paper proposes stacking ensemble machine learning (SEML) for calibration of NIRS data with two levels of learning involved. Eight (8) spectroscopy datasets from public repository and previously published works by the authors are used as the case study. The model well generalized the data in the respective regression tasks with R-2 of at least approximate to 0.8 in the test samples and in the respective classification tasks with classification accuracy (CA) of at least approximate to 0.8 also. In addition, the proposed SEML can improve, or at least reach par with, the accuracy of individual base learners in both train and test samples for all cases of regression and classification datasets. It shows superior performance in test samples for both regression and classification datasets with respectively R-2 ranging from 0.86 to nearly 1 and CA ranging from 0.89 to 1.
更多查看译文
关键词
chemometrics calibration,stacking ensemble machine learning,near infrared spectroscopy,food fraud detection,food safety and security
AI 理解论文
溯源树
样例
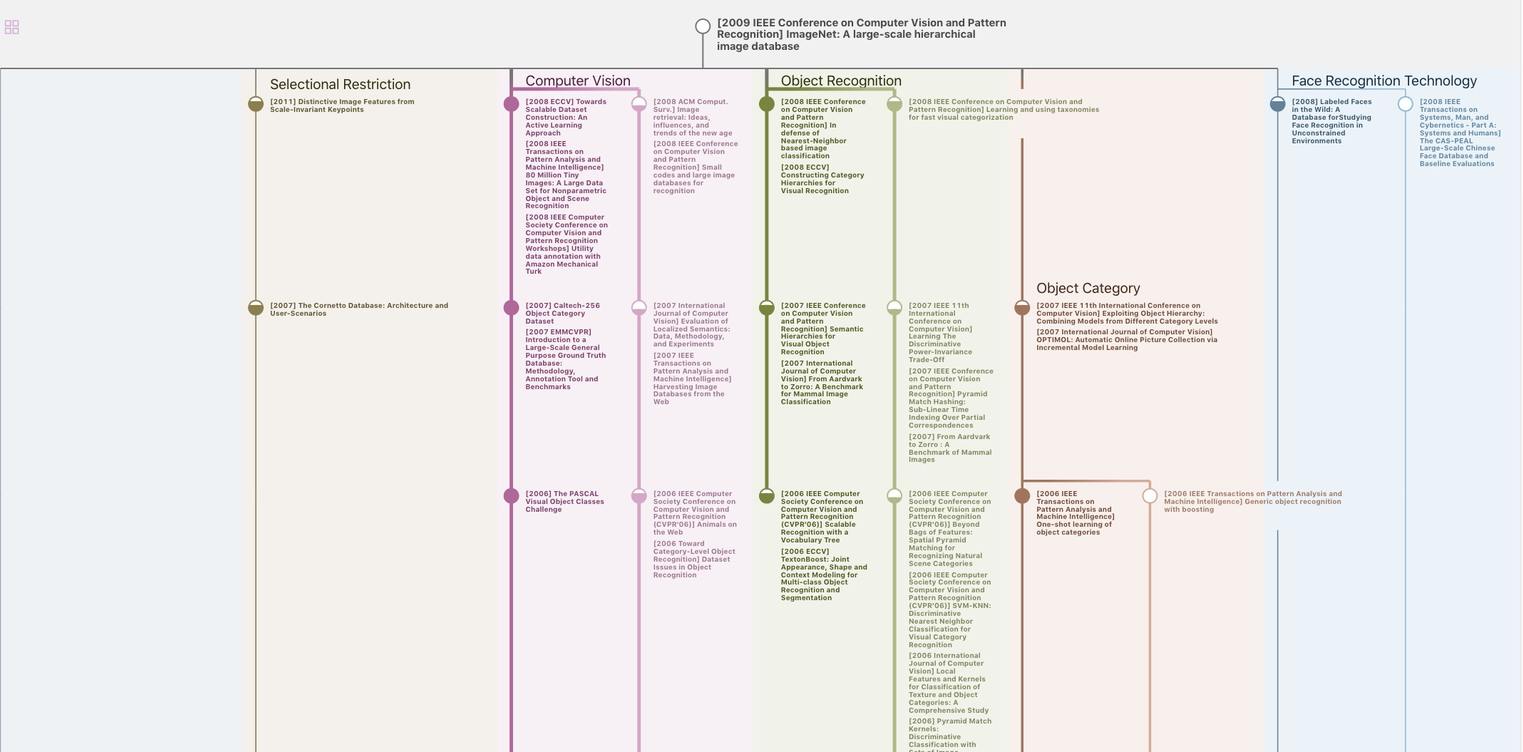
生成溯源树,研究论文发展脉络
Chat Paper
正在生成论文摘要