Generating Realistic and Representative Trajectories with Mobility Behavior Clustering
31ST ACM SIGSPATIAL INTERNATIONAL CONFERENCE ON ADVANCES IN GEOGRAPHIC INFORMATION SYSTEMS, ACM SIGSPATIAL GIS 2023(2023)
Abstract
Accessing realistic human movements (aka trajectories) is essential for many application domains, such as urban planning, transportation, and public health. However, due to privacy and commercial concerns, real-world trajectories are not readily available, giving rise to an important research area of generating synthetic but realistic trajectories. Inspired by the success of deep neural networks (DNN), data-driven methods learn the underlying human decision-making mechanisms and generate synthetic trajectories by directly fitting real-world data. However, these DNN-based approaches do not exploit people's moving behaviors (e.g., work commute, shopping purpose), significantly influencing human decisions during the generation process. This paper proposes MBP-GAIL, a novel framework based on generative adversarial imitation learning that synthesizes realistic trajectories that preserve moving behavior patterns in real data. MBP-GAIL models temporal dependencies by Recurrent Neural Networks (RNN) and combines the stochastic constraints from moving behavior patterns and spatial constraints in the learning process. Through comprehensive experiments, we demonstrate that MBP-GAIL outperforms state-of-the-art methods and can better support decision making in trajectory simulations.
MoreTranslated text
Key words
trajectory generation,GAIL
AI Read Science
Must-Reading Tree
Example
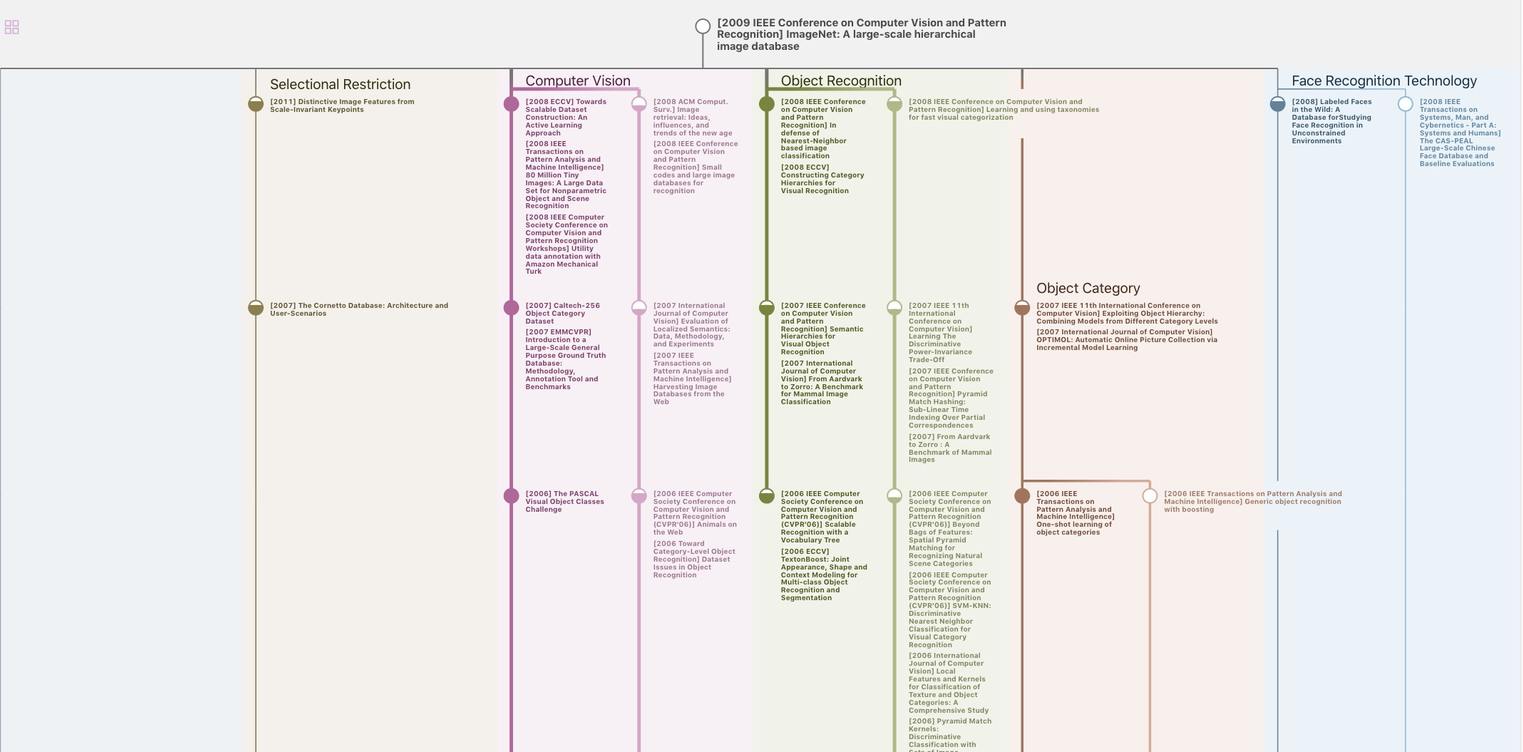
Generate MRT to find the research sequence of this paper
Chat Paper
Summary is being generated by the instructions you defined