Space-time Adaptive Processing Using a Model-based Deep Learning Method
2023 IEEE International Radar Conference (RADAR)(2023)
摘要
Space-time adaptive processing (STAP) is critical for clutter suppression in airborne radar, but limited training data is a challenge. Sparse recovery (SR) STAP has emerged to address this, but most existing approaches are model-based and require complex parameter tuning. To overcome this, we propose a novel framework that combines model-driven and learning-based approaches using a deep convolutional neural network based on the STAP principle and the half quadratic splitting algorithm. Our approach outputs the minimum variance distortionless response spectrum for clutter suppression, resulting in superior performance and computational efficiency compared to existing SR STAP methods. Our contribution lies in the novelty of the proposed framework for combining model-driven and learning-based approaches to effectively address the challenges of STAP. Moreover, we propose a novel data mapping that guarantees the accuracy of the spectrum estimation. The simulation results confirm the effectiveness of our proposed method.
更多查看译文
关键词
Space-time adaptive processing,sparse recovery,convolutional neural network,spectrum estimation
AI 理解论文
溯源树
样例
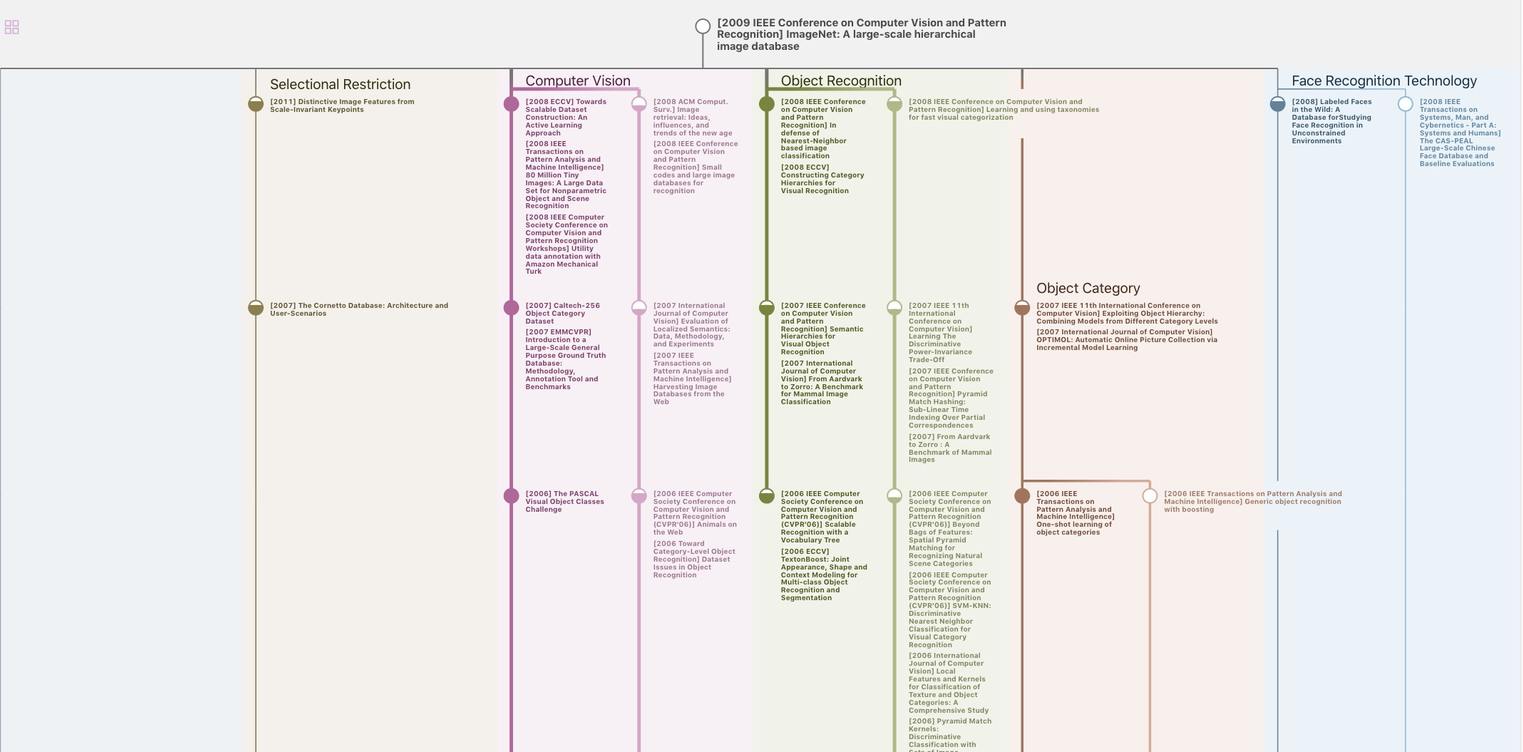
生成溯源树,研究论文发展脉络
Chat Paper
正在生成论文摘要