CalAttnMix: An instance-attention based data augmentation method for calcification detection in mammograms
BIOMEDICAL SIGNAL PROCESSING AND CONTROL(2024)
摘要
Data augmentation is widely used as a pre-processing step to solve limited training data and class imbalance problems in deep-learning based calcification detection methods. However, current augmentation methods in breast mammograms fail to guarantee the class balance and diversity of generated calcifications, especially that randomly fusing instances with specified regions results in unrealistic images, further degrading the performances. Here, we propose an instance-attention based data augmentation method, named Calcification-Attention-Mix (CalAttnMix), which adaptively transfers multiple source calcification instances into diverse breast tissue backgrounds, aiding breast calcification detection. First, to seek feasible breast tissue candidate backgrounds, an Augmentation Region Proposal Mechanism (ARPM) is devised based on morphology constraints of calcifications. Then, without segmentation masks of calcification foregrounds, we design a transformer-based Foreground Prediction Module (FPM), along with a multi-task learning strategy, to localize instances accurately in a weakly-supervised manner. Finally, to generate diverse instances, an attention mix module is introduced to dynamically mix instances with background candidates, simulating various breast tissue surroundings. By focusing on the minor class, CalAttnMix generates diverse and balanced datasets for calcification detection. Experiments on the public and clinical dataset show that CalAttnMix achieves the lowest FID 22.152 and cosine similarity score 0.304, respectively, confirming the realism and diversity of generated images. CalAttnMix outperforms SOTA augmentation methods by 3.40% / 2.30% and 1.40% / 5.00% in the average recall/mAP, demonstrating the best detection results.
更多查看译文
关键词
Calcification detection,Mammogram,Data augmentation,Instance-attention,Weakly-supervised
AI 理解论文
溯源树
样例
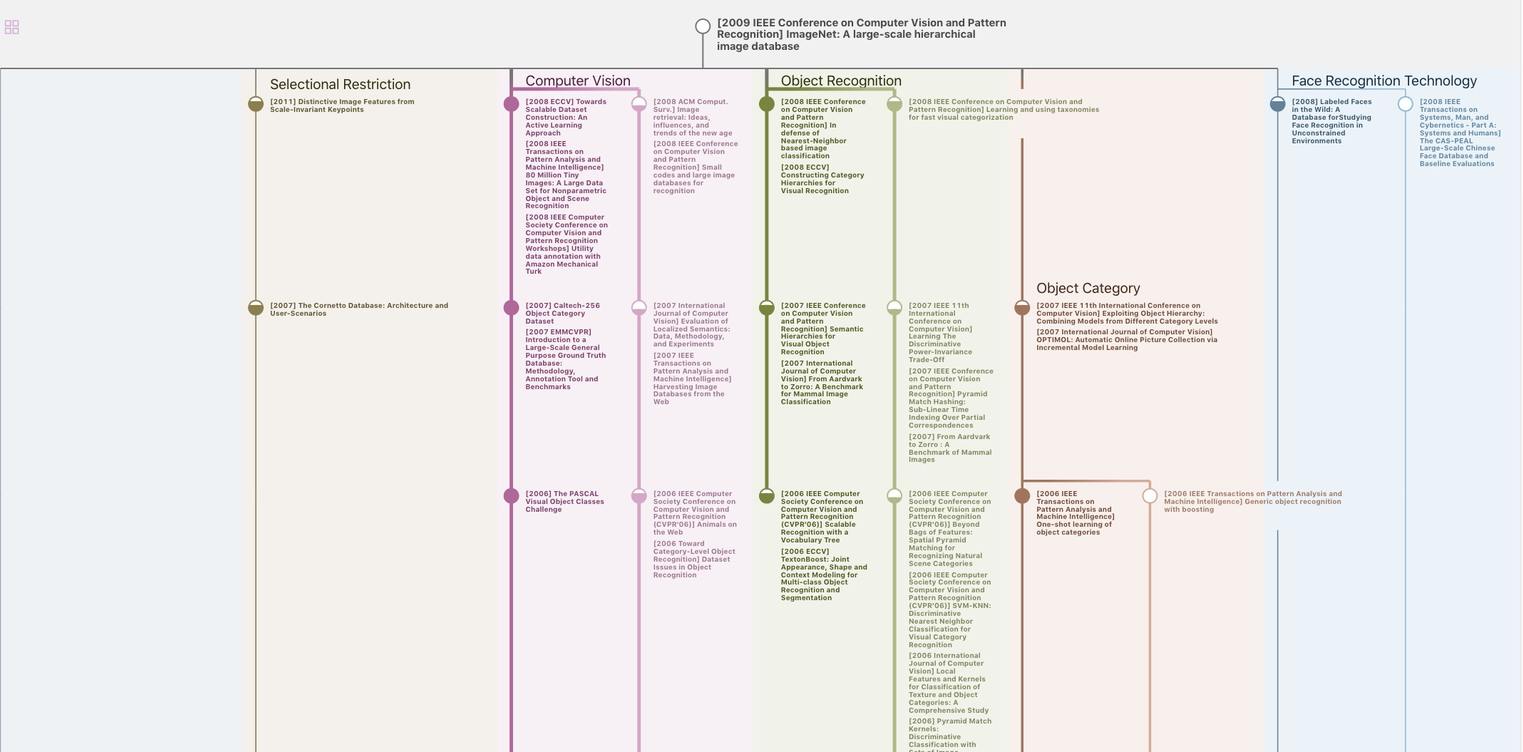
生成溯源树,研究论文发展脉络
Chat Paper
正在生成论文摘要