Statistically assessing vertical change on a sandy beach from permanent laser scanning time series
ISPRS Open Journal of Photogrammetry and Remote Sensing(2024)
摘要
In the view of climate change, understanding and managing effects on coastal areas and adjacent cities is essential. Permanent Laser Scanning (PLS) is a successful technique to not only observe notably sandy coasts incidentally or once every year, but (nearly) continuously over extended periods of time. The collected point cloud observations form a 4D point cloud data set representing the evolution of the coast provide the opportunity to assess change processes at high level of detail. For an exemplary location in Noordwijk, The Netherlands, three years of hourly point clouds were acquired on a 1 km long section of a typical Dutch urban sandy beach. Often, the so-called level of detection is used to assess point cloud differences from two epochs. To explicitly incorporate the temporal dimension of the height estimates from the point cloud data set, we revisit statistical testing theory. We apply multiple hypothesis testing on elevation time series in order to identify different coastal processes, like aeolian sand transport or bulldozer works. We then estimate the minimal detectable bias for different alternative hypotheses, to quantify the minimal elevation change that can be estimated from the PLS observations over a certain period of time. Additionally, we analyse potential error sources and influences on the elevation estimations and provide orders of magnitudes and possible ways to deal with them. Finally we conclude that elevation time series from a long term PLS data set are a suitable input to identify aeolian sand transport with the help of multiple hypothesis testing. In our example case, slopes of 0.032 m/day and sudden changes of 0.031 m can be identified with statistical power of 80% and with 95% significance in 24-h time series on the upper beach. In the intertidal area the presented method allows to classify daily elevation time series over one month according to the dominating model (sudden change or linear trend) in either eroding or accreting behaviour.
更多查看译文
AI 理解论文
溯源树
样例
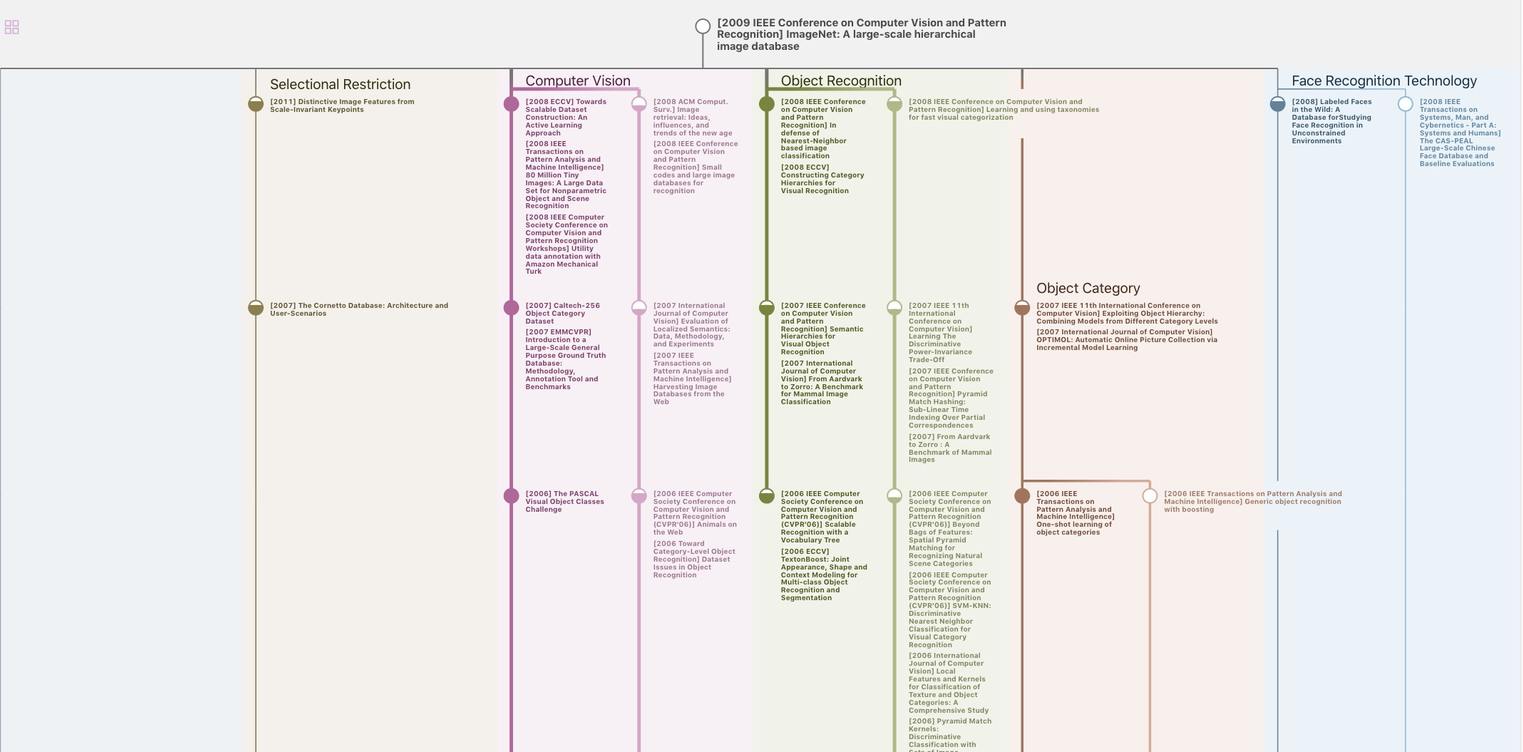
生成溯源树,研究论文发展脉络
Chat Paper
正在生成论文摘要