Human-Machine Hybrid Strategy for Defect Semantic Segmentation With Limited Data
IEEE TRANSACTIONS ON INSTRUMENTATION AND MEASUREMENT(2024)
摘要
In the field of industrial intelligent inspection, two problems need to be solved urgently. One is how to integrate complex industrial inspection expertise into algorithms. The second is how to predict other similar products that have not been seen through prior knowledge of existing products. Solving these two problems can effectively solve the problems of insufficient samples and poor adaptability. Therefore, we propose a human-machine hybrid strategy to construct a promptable industrial scene segmentation task by human input of easy-to-obtain defect-free images or text as guidance information. Specifically, for unseen categories in unseen scenes, we propose to use a defect-free image as the guidance information, establish accurate pixel-level correspondence between defect-free and query images, and decode correlation maps through the 3-D residual convolutional network. For unseen categories in seen scenes, we propose a text-image model based on contrastive language-image pretraining (CLIP) to extract visual features aligned with the text. To establish more accurate text guidance, we obtain the holistic pattern of text features through global self-attention and establish global guidance between text and visual features. Extensive experiments demonstrate that our proposed approach, requiring no additional training or fine-tuning and solely relying on defect-free images and text as guidance, effectively segments previously unseen defect categories in both seen and unseen scenes. This method efficiently addresses the challenge of limited defect samples in the industrial domain.
更多查看译文
关键词
Defect segmentation,few-shot learning,human-machine hybrid,multimodal data,neural network application
AI 理解论文
溯源树
样例
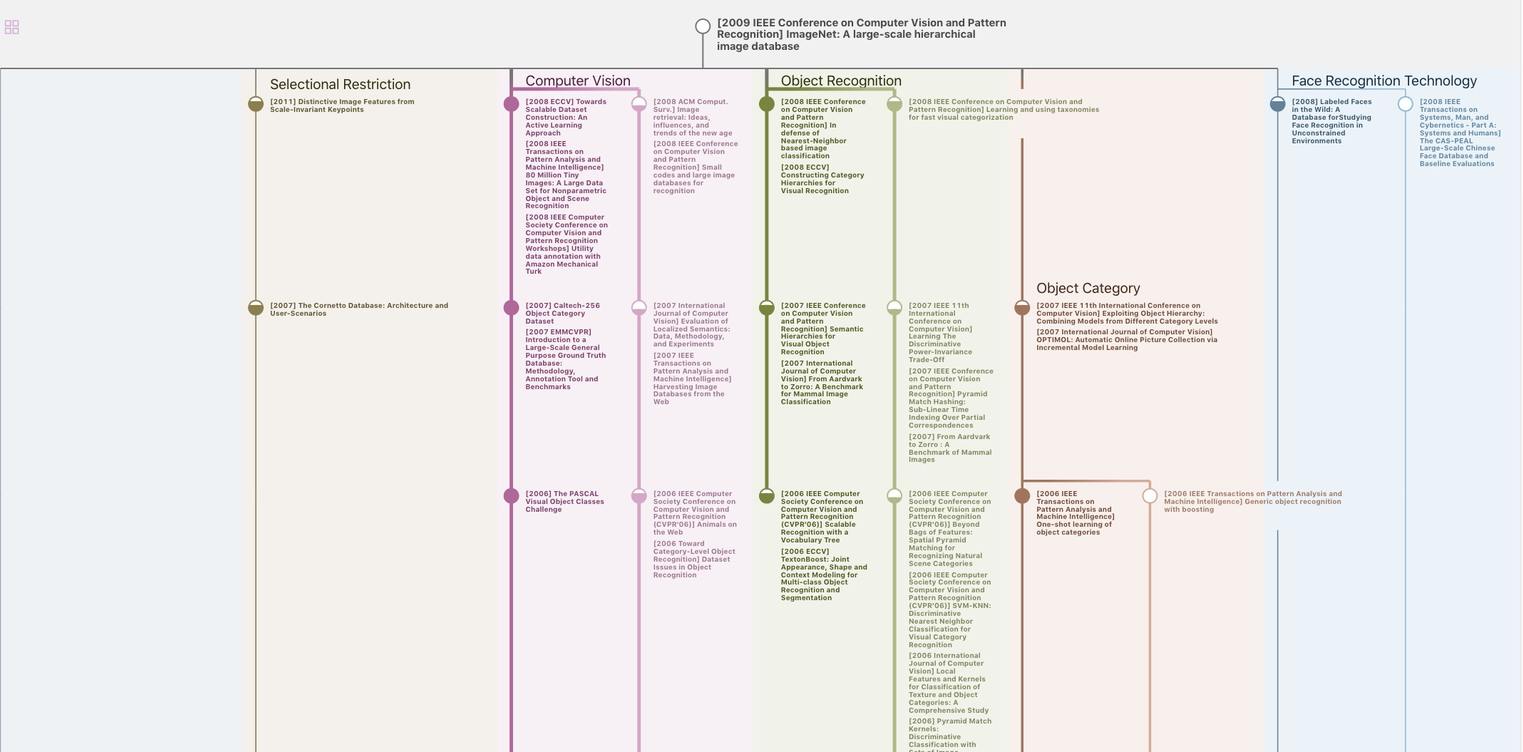
生成溯源树,研究论文发展脉络
Chat Paper
正在生成论文摘要