Meta-TFEN: A Multi-Modal Deep Learning Approach for Encrypted Malicious Traffic Detection.
2023 33rd International Telecommunication Networks and Applications Conference(2023)
Abstract
Malware poses a significant threat to internet security. Existing deep learning-based methods for malware traffic detection typically rely on single-modal features, overlooking the heterogeneity of encrypted traffic, thus limiting their detection performance. To address this limitation, this paper proposes a multi-modal deep learning approach called Meta-TFEN for detecting encrypted malicious traffic. The method utilizes TCN, Bi-GRU, and LSTM to extract multi-modal features including the payload of secure transport layer protocols, statistical features, and features of TLS encryption activities. It employs an fusion network to capture the dependencies between modalities and integrates discriminative features to enhance detection performance. Additionally, this paper uses a meta-learning framework for classification to enable rapid deployment of the model. The performance of Meta-TEEN evaluated on public datasets and its applicability in real-world environments is explored using real samples. The experimental results clearly demonstrate that the Meta-TEEN method surpasses other state-of-the-art methods in terms of accuracy.
MoreTranslated text
Key words
TLS protocol,Malicious traffic,Deep Learning,Multimodal,Meta-learning
AI Read Science
Must-Reading Tree
Example
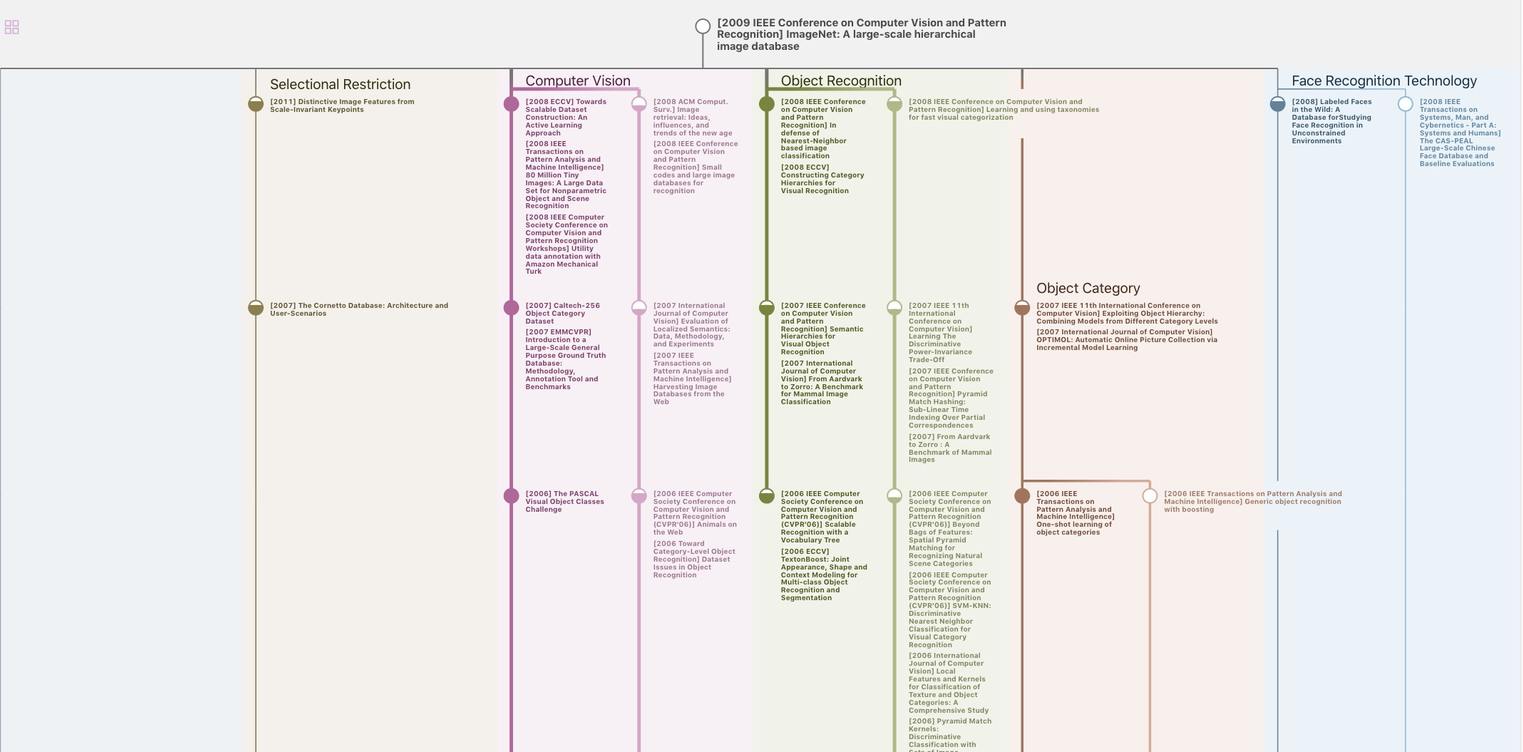
Generate MRT to find the research sequence of this paper
Chat Paper
Summary is being generated by the instructions you defined