KD-SCFNet: Towards more accurate and lightweight salient object detection via knowledge distillation
NEUROCOMPUTING(2024)
摘要
Most existing salient object detection (SOD) models are difficult to apply due to the complex and huge model structures. Although some lightweight models are proposed, the accuracy is barely satisfactory. In this paper, we design a novel semantics-guided contextual fusion network (SCFNet) that focuses on the interactive fusion of multi-level features for accurate and efficient salient object detection. Furthermore, we apply knowledge distillation to SOD task and provide a sizeable dataset KD-SOD80K. In detail, we transfer the rich knowledge from a seasoned teacher to the untrained SCFNet through unlabeled images, enabling SCFNet to learn a strong generalization ability to detect salient objects more accurately. The knowledge distillation based SCFNet (KD-SCFNet) achieves comparable accuracy to the state-of-the-art heavyweight methods with less than 1M parameters and 174 FPS real-time detection speed. Extensive experiments demonstrate the robustness and effectiveness of the proposed distillation method and SOD framework.
更多查看译文
关键词
Deep learning,Salient object detection,Knowledge distillation,Lightweight SOD
AI 理解论文
溯源树
样例
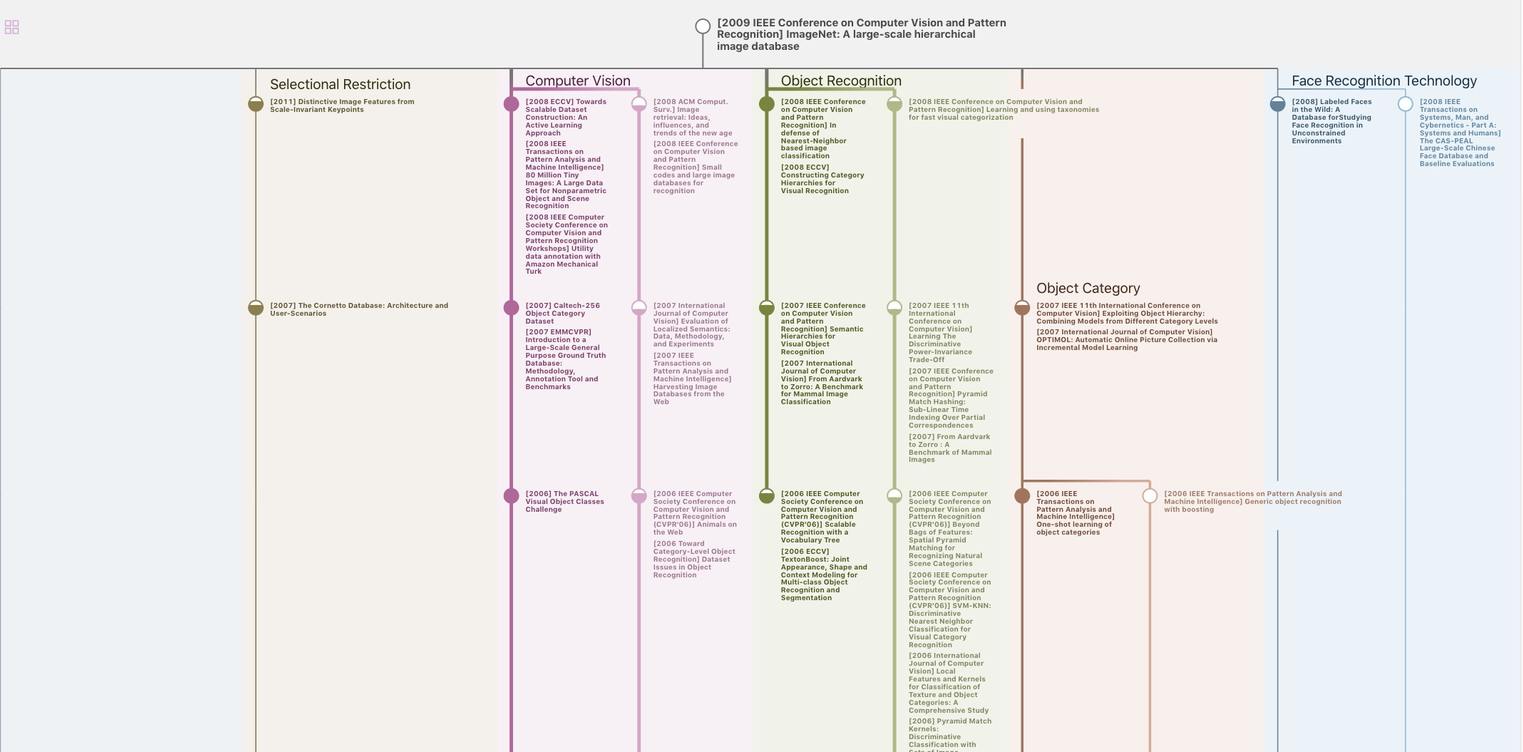
生成溯源树,研究论文发展脉络
Chat Paper
正在生成论文摘要