Infrared Object Detection Method Based on DBD-YOLOv8
IEEE ACCESS(2023)
摘要
An innovative and improved method for infrared object detection, namely DBD-YOLOv8 (DCN-BiRA-DyHeads-YOLOv8), is presented. The inherent limitations of the YOLOv8 model in scenarios with a low signal-to-noise ratio and complex tasks are addressed, with a focus on improving the multi-scale feature representation within the YOLOv8 framework and effectively filtering out irrelevant regions. To achieve this, two crucial modules, D_C2f and D_SPPF, are integrated. Deformable convolutions (DCN) are utilized by these modules to dynamically adjust the visual receptive fields of the network. Furthermore, a Bi-level Routing Attention mechanism (BRA) and Dynamic Heads (DyHeads) are adapted within the feature fusion network, refining feature maps and enhancing semantic representation through attention mechanisms. Significant improvements are demonstrated by DBD-YOLOv8 when compared to the YOLOv8-n\s\m\1\x series models. Notably, improved average mAP@0.5 values on benchmark datasets, including FLIR, OTCBVS (Dataset 01), OTCBVS (Dataset 03), and VEDAI, are achieved by DBD-YOLOv8. The corresponding values are 84.8%, 96.3%, 99.7%, and 76.0%, respectively. These results represent increases of 7.9%, 1.5%, 0.1%, and 3.5%, respectively. Importantly, real-time requirements are met by the model's inference times, which measure 10.9ms, 32.0ms, 37.3ms, and 28.4ms accordingly for the previous datasets.
更多查看译文
关键词
Infrared object detection,deformable convolution,Bi-level routing attention,dynamic head
AI 理解论文
溯源树
样例
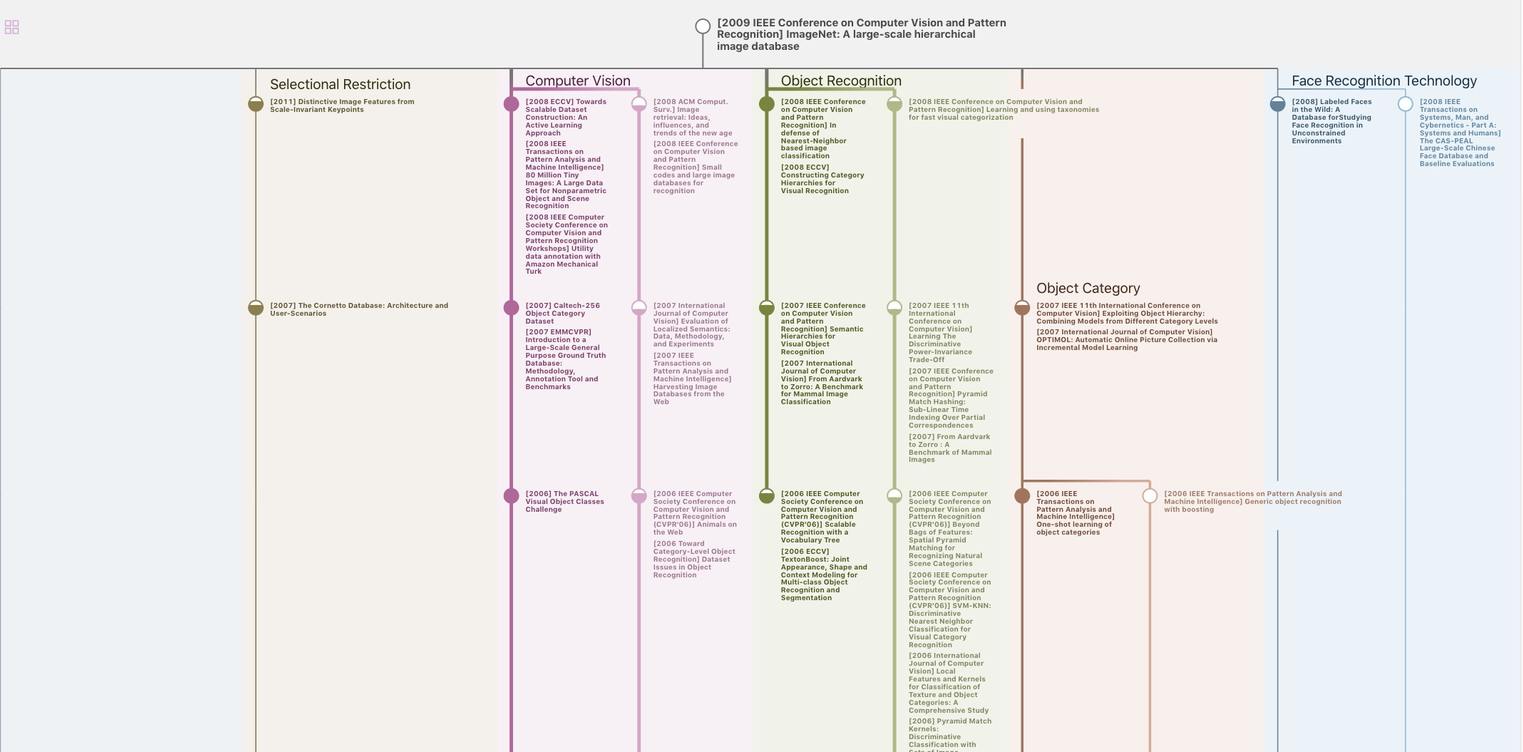
生成溯源树,研究论文发展脉络
Chat Paper
正在生成论文摘要