Multi-view robust regression for feature extraction
PATTERN RECOGNITION(2024)
Abstract
Recently, Multi -view Discriminant Analysis (MVDA) has been proposed and achieves good performance in multi -view recognition tasks. However, as an extension of LDA, this method still suffers from the small -class problem and has the sensitivity to outliers. In order to address these drawbacks and achieve better performance on multi -view recognition tasks, we proposed Multi -view Robust Regression (MVRR) for multi -view feature extraction. MVRR is a regression based method that imposes L21 norm as the metric of the loss function and the regularization term to improve robustness and obtain jointly sparse projection matrices for effective feature extraction. Moreover, we incorporate an orthogonal matrix to regress the extracted features to their scaled label to avoid the small -class problem. Therefore, MVRR guarantees the projection matrix to break through the restriction of the number of class for solving the small -class problem. We also propose an iterative algorithm to compute the optimal solution of MVRR and the convergence of MVRR is proved. Experiments are conducted on four databases to verify the performance of MVRR and the result illustrates that MVRR is robust on multi -view feature extraction.
MoreTranslated text
Key words
Image classification,Small-class problem,Linear regression (LR)
AI Read Science
Must-Reading Tree
Example
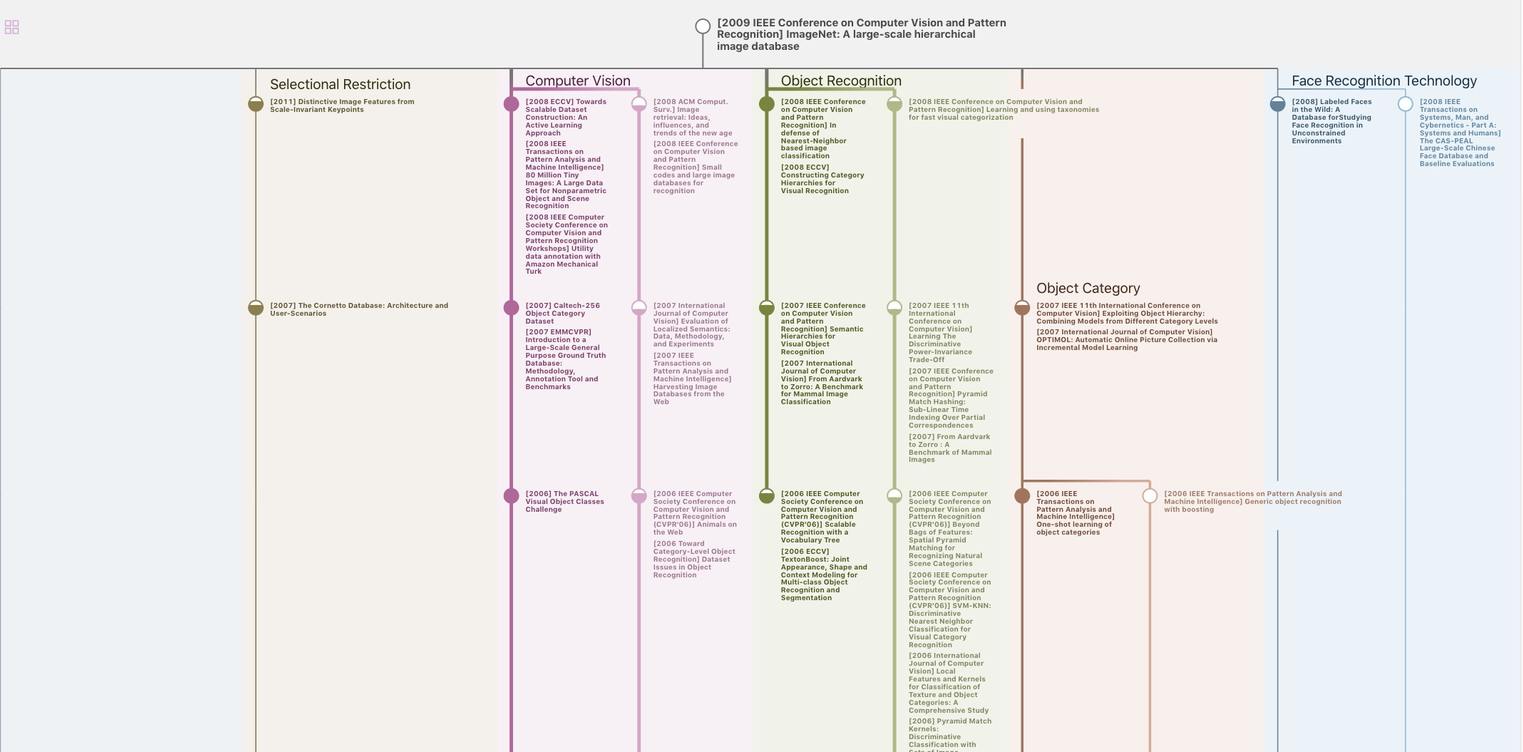
Generate MRT to find the research sequence of this paper
Chat Paper
Summary is being generated by the instructions you defined