Some thoughts about transfer learning. What role for the source domain?
INTERNATIONAL JOURNAL OF APPROXIMATE REASONING(2024)
摘要
Transfer learning is called for when the training and test data do not share the same input distributions (PSX # PTX) or/and not the same conditional ones (PSY|X # PTY|X). In the most general case, the input spaces and/or output spaces can be different: XS # XT and/or YS # YT. However, most work assume that XS = XT. Furthermore, a common held assumption is that it is necessary that the source hypothesis be good on the source training data and that the "distance" between the source and the target domains be as small as possible in order to get a good (transferred) target hypothesis. This paper revisits the reasons for these beliefs and discusses the relevance of these conditions. An algorithm is presented which can deal with transfer learning problems where XS # XT, and that furthermore brings a fresh perspective on the role of the source hypothesis (it does not have to be good) and on what is important in the distance between the source and the target domains (translations between them should belong to a limited set). Experiments illustrate the properties of the method and confirm the theoretical analysis. Determining beforehand a relevant source hypothesis remains an open problem, but the vista provided here helps understanding its role.
更多查看译文
关键词
Transfer learning,Domain adaptation,Out of distribution learning
AI 理解论文
溯源树
样例
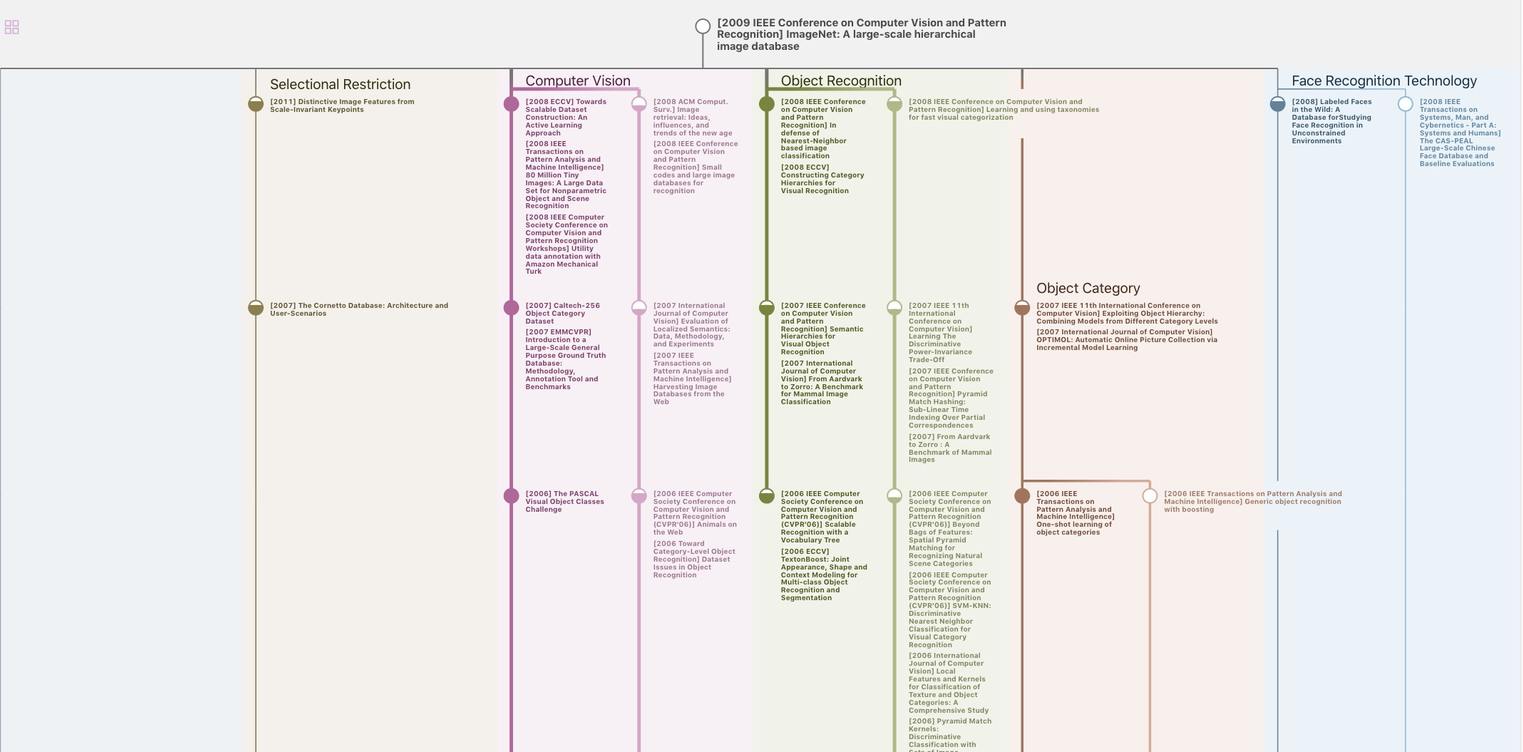
生成溯源树,研究论文发展脉络
Chat Paper
正在生成论文摘要