SwinFG: A fine-grained recognition scheme based on swin transformer
EXPERT SYSTEMS WITH APPLICATIONS(2024)
摘要
Fine-grained image recognition (FGIR) is a challenging task as it requires the recognition of sub-categories with subtle differences. Recently, the swin transformer has shown impressive performance in various fields. Our research has shown that swin transformer applied directly to FGIR is also highly effective compared to many other approaches and can be further enhanced with adaptive improvements. In this paper, we propose a novel swin transformer based architecture, named SwinFG, which enhances FGIR by leveraging shifted window based self-attention to locate discriminative regions. The self-attention computation fuses image patches together based on attention weights, enabling the subsequent influence of each patch to be tracked and its contribution to the extracted feature to be determined. This forms the basis for locating discriminative regions. To this end, we propose a series of transformations that integrate the attention weights of local windows in each block into attention maps, which can be recursively multiplied to track changes in the attention weights. As the discriminative regions are not entirely occupied by the foreground object, the background information is also expressed in the extracted feature inevitably. To address this, we propose conducting contrastive learning on features obtained from both the discriminative and background regions of a single image to enlarge their distance and further eliminate any potential influence from the background. We demonstrate the state-of-the-art performance of our model on four popular fine-grained benchmarks. (The code is available at https://anonymous.4open.science/r/swinFG-1DCE).
更多查看译文
关键词
Swin transformer,Fine-grained image recognition,Image classification,Visual attention,Local region feature,Discriminative foreground
AI 理解论文
溯源树
样例
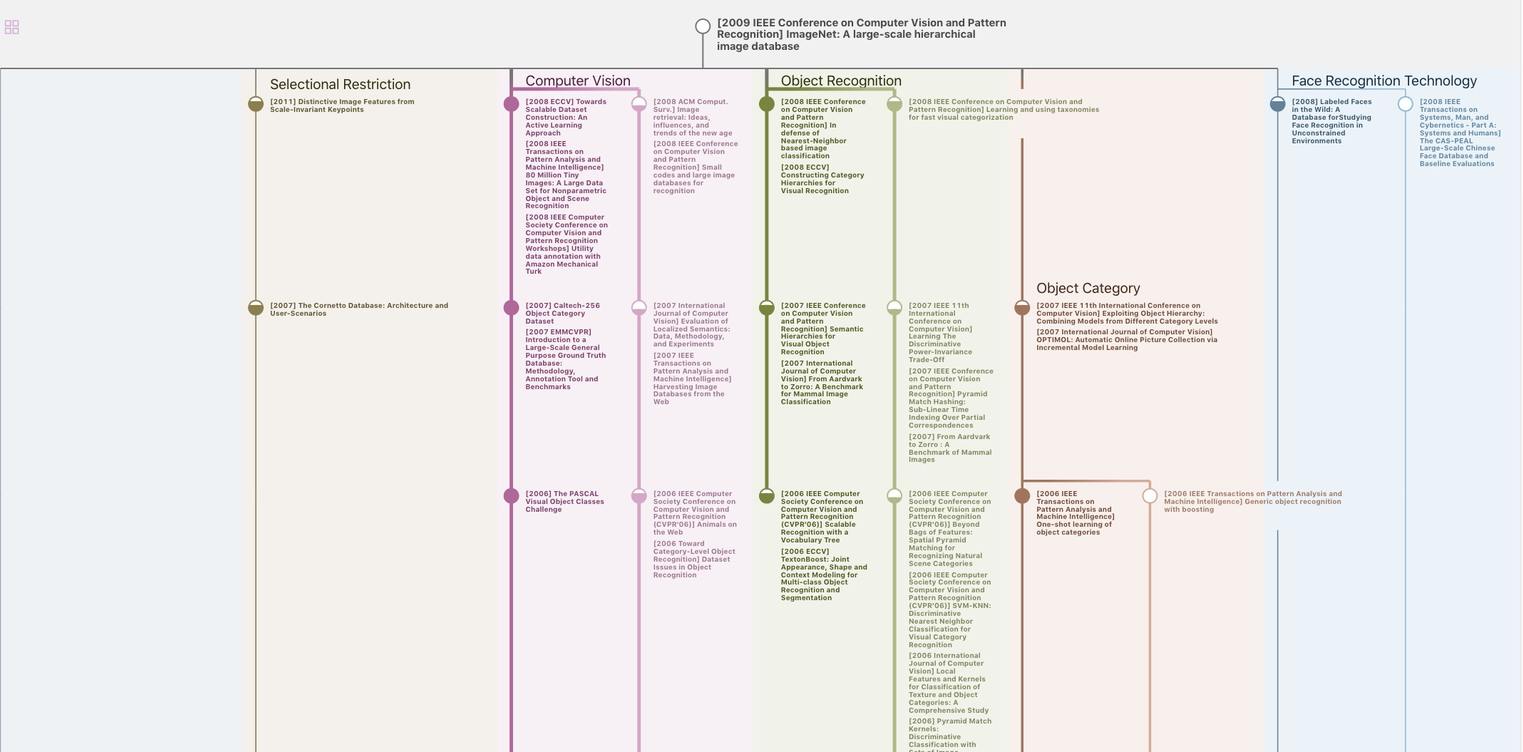
生成溯源树,研究论文发展脉络
Chat Paper
正在生成论文摘要