Multiscale Progressive Fusion Filter Network for Infrared Small Target Detection
IEEE TRANSACTIONS ON GEOSCIENCE AND REMOTE SENSING(2024)
摘要
Infrared small target detection is widely used in remote sensing fields. However, the application scenes of space-based remote sensing imaging often lead to problems such as small target scale, weak energy, and serious influence by strong clutters. At present, traditional methods are often difficult to adapt to the change of target scale. And deep-learning methods often difficult to extract small target features, and the change in imaging characteristics also brings challenges to the generalization ability. To complement each other's advantages, we propose an infrared small target detection method that combines the traditional methods with the deep-learning methods. First, we construct a multistage feature extraction network for guiding the typical multiscale traditional filtering results to progressively fuse. Second, we propose a multiscale attention supervision (MAS) module to adjust the semantic consistency of different stages, improving the network generalization ability. Next, a dynamic weight convolution (DWC) module is utilized to obtain the optimal distribution of grayscale in the neighborhood. Finally, we use the background modeling results to suppress the background, effectively weakening the influence of background clutters and enhancing the target contrast. Experimental results show that the proposed method has good detection results for targets with different scales and signal-to-clutter ratios in a variety of complex scenes. Compared with the typical methods, our method has better detection performance and generalization ability.
更多查看译文
关键词
Feature extraction,Object detection,Deep learning,Remote sensing,Imaging,Clutter,Information filters,Attention mechanism,complex background,infrared small target detection,multiscale information,spatial filtering
AI 理解论文
溯源树
样例
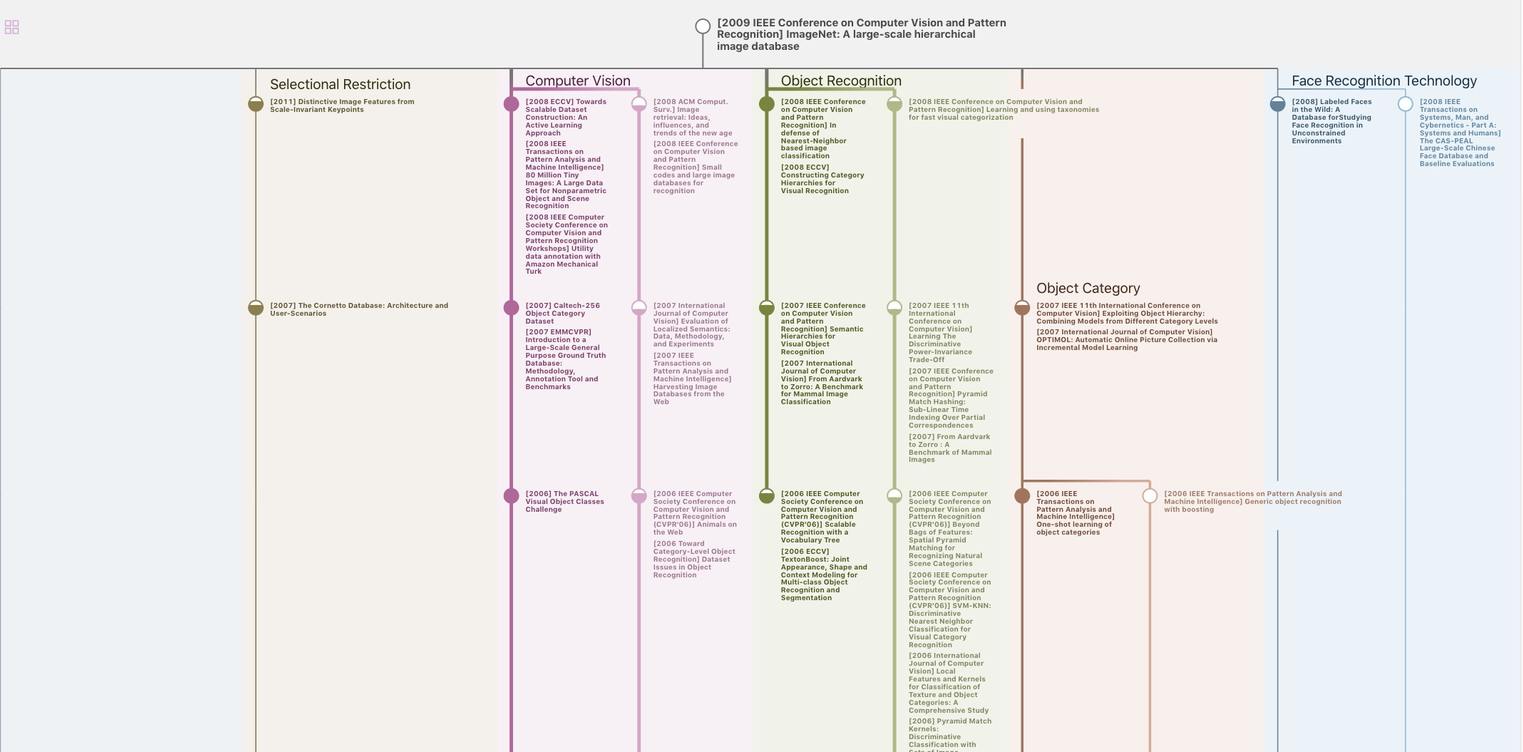
生成溯源树,研究论文发展脉络
Chat Paper
正在生成论文摘要