Deep reinforcement learning-based air combat maneuver decision-making: literature review, implementation tutorial and future direction
Artificial Intelligence Review(2024)
摘要
Nowadays, various innovative air combat paradigms that rely on unmanned aerial vehicles (UAVs), i.e., UAV swarm and UAV-manned aircraft cooperation, have received great attention worldwide. During the operation, UAVs are expected to perform agile and safe maneuvers according to the dynamic mission requirement and complicated battlefield environment. Deep reinforcement learning (DRL), which is suitable for sequential decision-making process, provides a powerful solution tool for air combat maneuver decision-making (ACMD), and hundreds of related research papers have been published in the last five years. However, as an emerging topic, there lacks a systematic review and tutorial. For this reason, this paper first provides a comprehensive literature review to help people grasp a whole picture of this field. It starts from the DRL itself and then extents to its application in ACMD. And special attentions are given to the design of reward function, which is the core of DRL-based ACMD. Then, a maneuver decision-making method based on one-to-one dogfight scenarios is proposed to enable UAV to win short-range air combat. The model establishment, program design, training methods and performance evaluation are described in detail. And the associated Python codes are available at gitee.com/wangyyhhh, thus enabling a quick-start for researchers to build their own ACMD applications by slight modifications. Finally, limitations of the considered model, as well as the possible future research direction for intelligent air combat, are also discussed.
更多查看译文
关键词
Artificial intelligence,Unmanned aerial vehicle (UAV),Deep reinforcement learning (DRL),Air combat maneuver decision-making (ACMD)
AI 理解论文
溯源树
样例
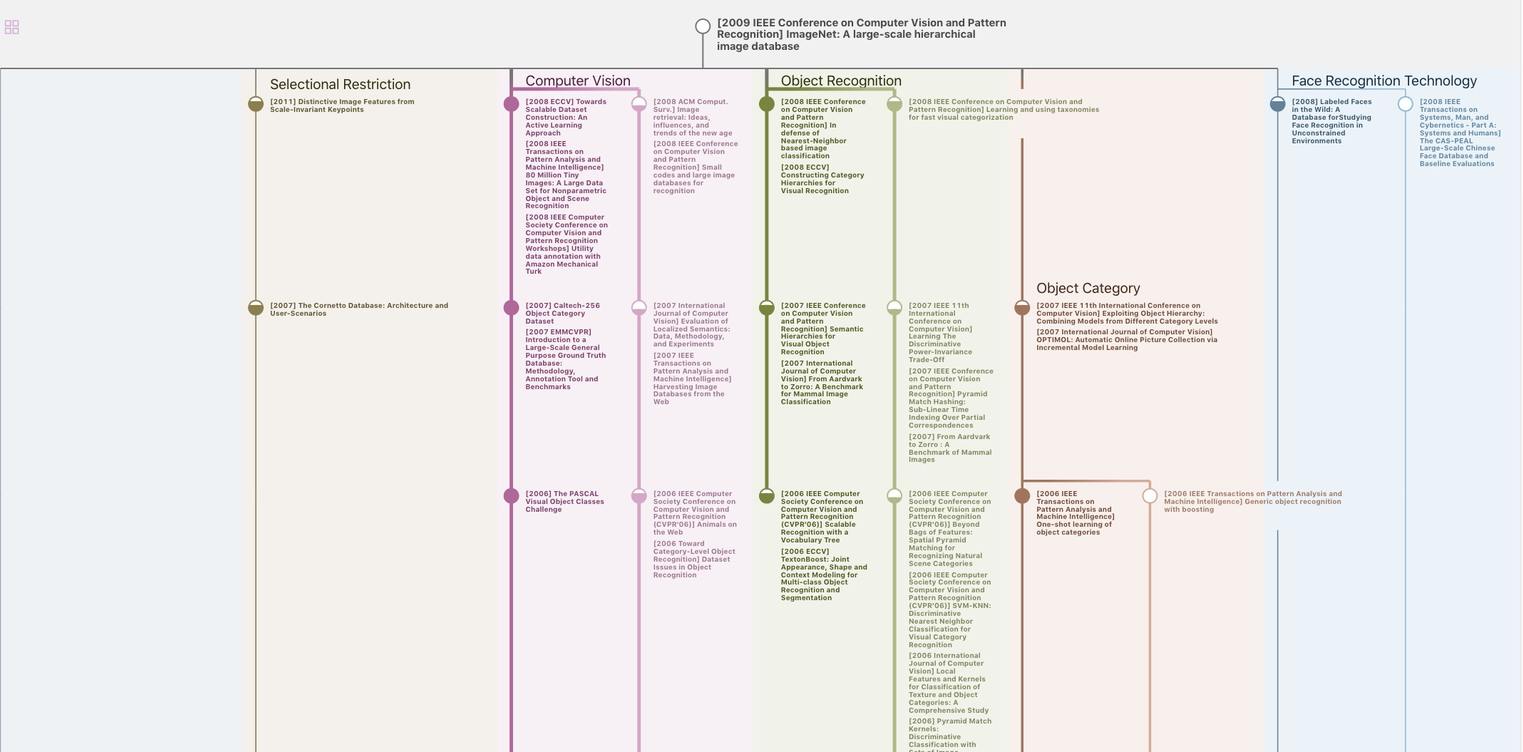
生成溯源树,研究论文发展脉络
Chat Paper
正在生成论文摘要