Uncovering acoustic signatures of pore formation in laser powder bed fusion
The International Journal of Advanced Manufacturing Technology(2023)
摘要
We present a machine learning workflow to discover signatures in acoustic measurements that can be utilized to create a low-dimensional model to accurately predict the location of keyhole pores formed during additive manufacturing processes. Acoustic measurements were sampled at 100 kHz during single-layer laser powder bed fusion (LPBF) experiments, and spatio-temporal registration of pore locations was obtained from post-build radiography. Power spectral density (PSD) estimates of the acoustic data were then decomposed using non-negative matrix factorization with custom k -means clustering (NMF k ) to learn the underlying spectral patterns associated with pore formation. NMF k returned a library of basis signals and matching coefficients to blindly construct a feature space based on the PSD estimates in an optimized fashion. Moreover, the NMF k decomposition led to the development of computationally inexpensive machine learning models which are capable of quickly and accurately identifying pore formation with classification accuracy of supervised and unsupervised label learning greater than 95
更多查看译文
关键词
Additive manufacturing,Laser powder bed fusion,Acoustic monitoring,Unsupervised learning,Non-negative matrix factorization,Dimensionality reduction
AI 理解论文
溯源树
样例
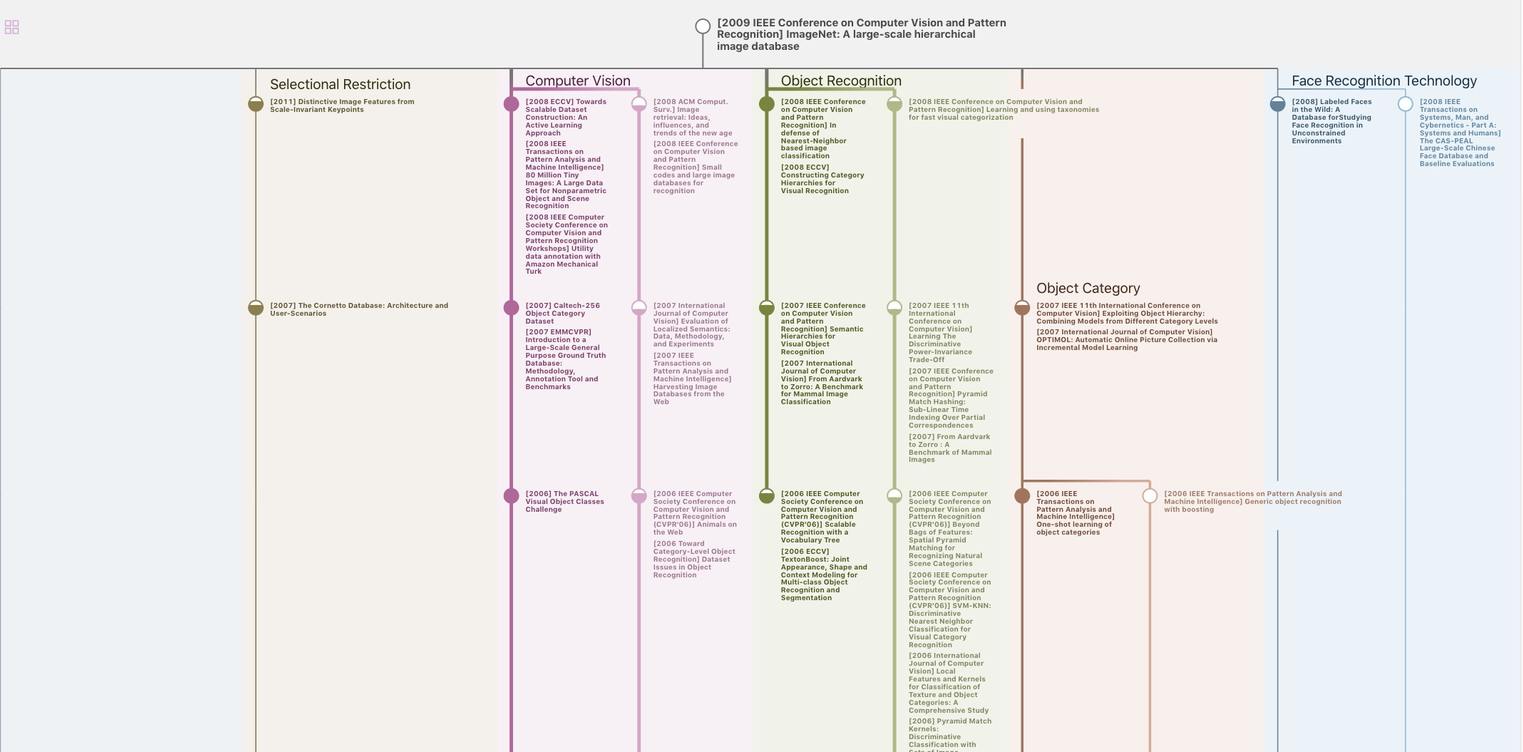
生成溯源树,研究论文发展脉络
Chat Paper
正在生成论文摘要