Stability and performance analysis of the compressed Kalman filter algorithm for sparse stochastic systems
Science China Technological Sciences(2023)
摘要
This paper considers the problem of estimating unknown sparse time-varying signals for stochastic dynamic systems. To deal with the challenges of extensive sparsity, we resort to the compressed sensing method and propose a compressed Kalman filter (KF) algorithm. Our algorithm first compresses the original high-dimensional sparse regression vector via the sensing matrix and then obtains a KF estimate in the compressed low-dimensional space. Subsequently, the original high-dimensional sparse signals can be well recovered by a reconstruction technique. To ensure stability and establish upper bounds on the estimation errors, we introduce a compressed excitation condition without imposing independence or stationarity on the system signal, and therefore suitable for feedback systems. We further present the performance of the compressed KF algorithm. Specifically, we show that the mean square compressed tracking error matrix can be approximately calculated by a linear deterministic difference matrix equation, which can be readily evaluated, analyzed, and optimized. Finally, a numerical example demonstrates that our algorithm outperforms the standard uncompressed KF algorithm and other compressed algorithms for estimating high-dimensional sparse signals.
更多查看译文
关键词
sparse signal,compressed sensing,Kalman filter algorithm,compressed excitation condition,stochastic stability,tracking performance
AI 理解论文
溯源树
样例
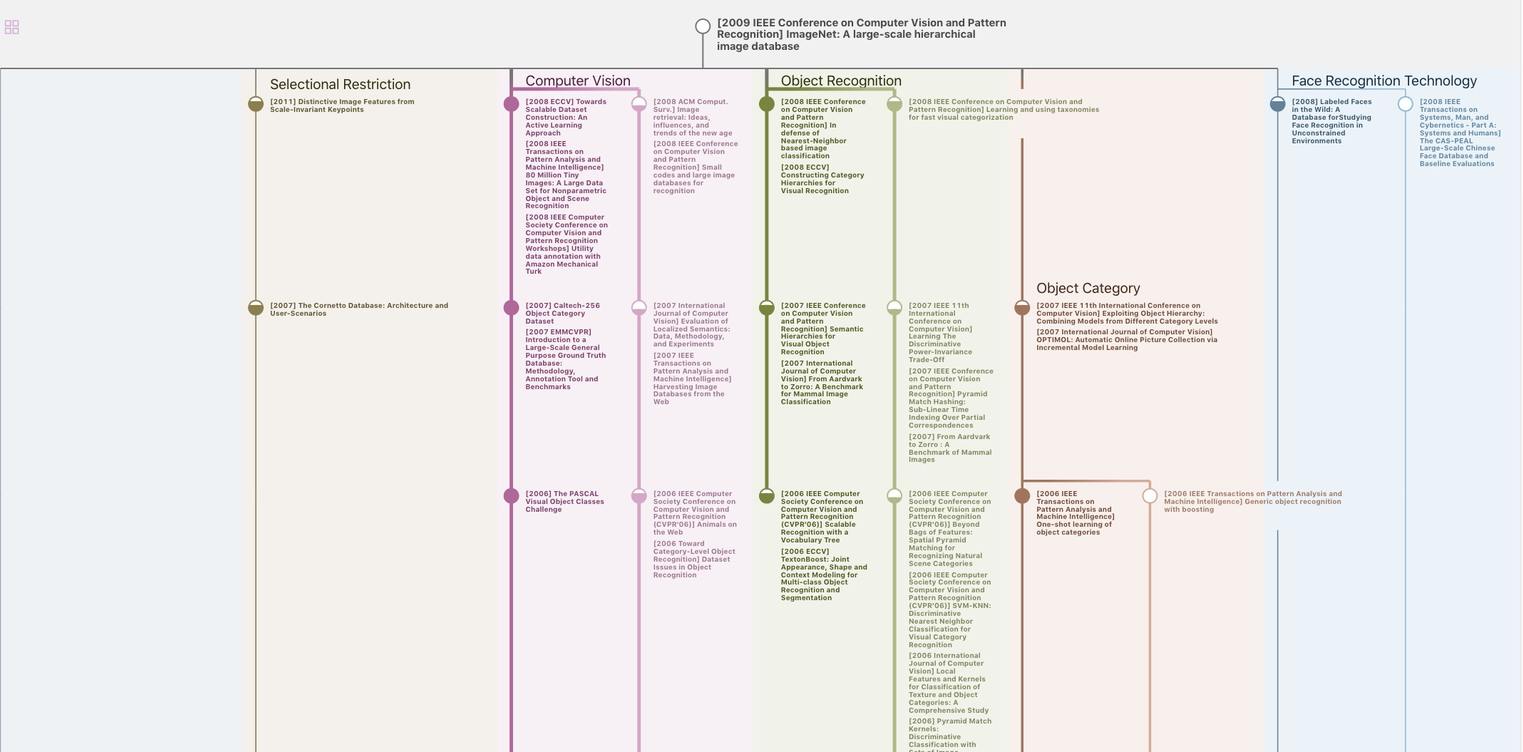
生成溯源树,研究论文发展脉络
Chat Paper
正在生成论文摘要