Pattern recognition system for rapid detection of gases using microfluidic olfaction detector: A case study using methane and ethane
SENSORS AND ACTUATORS B-CHEMICAL(2024)
摘要
Two significant shortcomings of metal oxide semiconductor (MOS) sensors are their low selectivity and prolonged recovery time after exposure to target gases. Microfluidic olfaction detectors (MFOD), having an embedded MOS at the distal end of a microchannel, improve MOS selectivity but further prolong the recovery time due to the delay in the gas movement as the result of diffusion through the microchannel. This paper aims to reduce the sampling time of a single MFOD. Such reduction is achieved here by developing a pattern recognition system involving: (i) an ensemble of four heterogeneous base machine learning models, (ii) a Long-Short Term Memory Neural Network (LSTM) to construct a portion of the transient response, (iii) features from an additional fast response sensor, and (iv) detachment of MOS from the microchannel for accelerated recovery. Using data collected from methane and ethane as a case study, the results of classification and regression demonstrate the need for only 17.5% of the previously used response time without any loss in accuracy. This reduces the total testing cycle of an MFOD by 60%, further enhancing the ability of selective MFODs by enabling faster detection of gases.
更多查看译文
关键词
Time series prediction,Machine learning,Electronic nose,Microfluidic,Gas sensor,Rapid detection
AI 理解论文
溯源树
样例
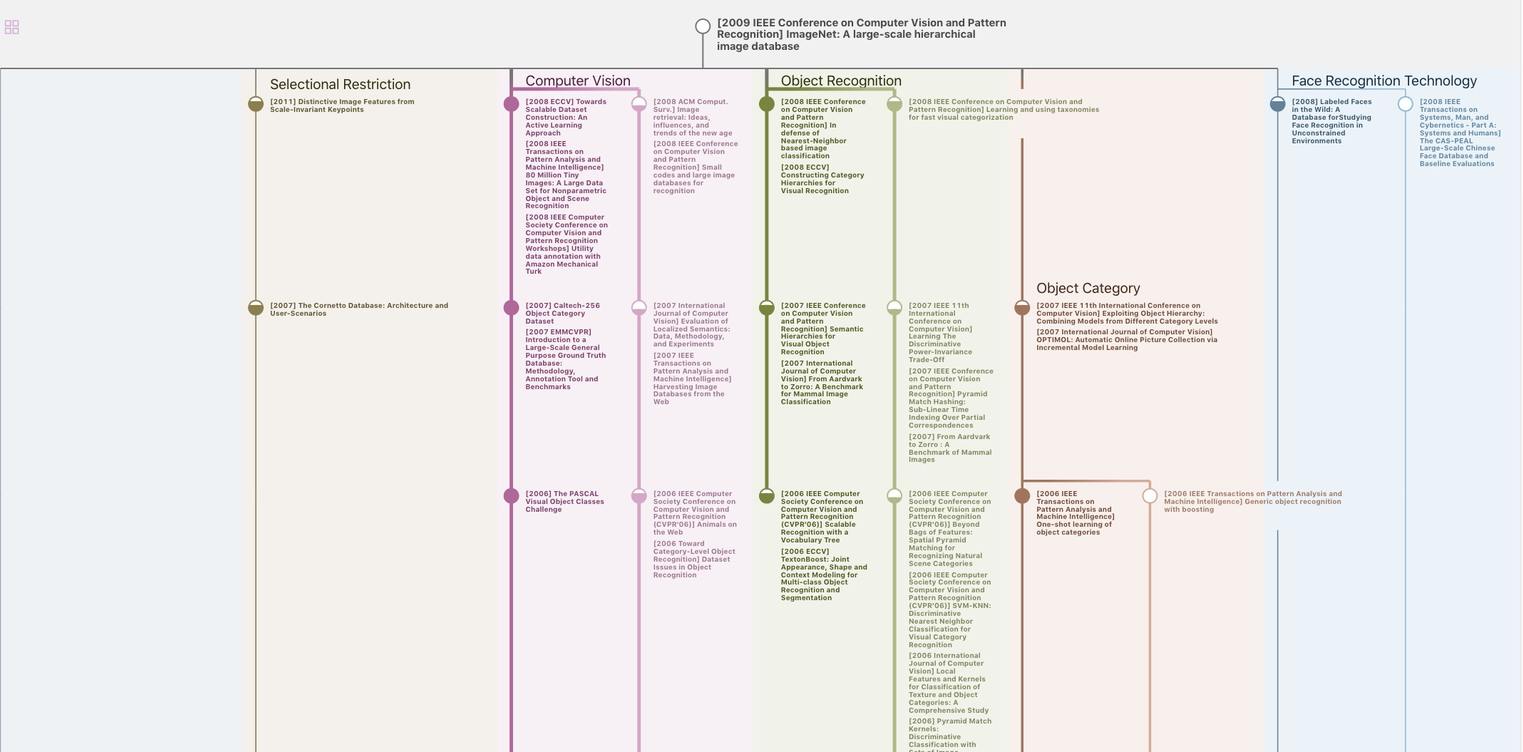
生成溯源树,研究论文发展脉络
Chat Paper
正在生成论文摘要