Price predictability at ultra-high frequency: Entropy-based randomness test
arxiv(2023)
Abstract
We use the statistical properties of Shannon entropy estimator and
Neyman-Pearson statistics to study the predictability of ultra-high frequency
financial data. We develop a statistical test for the predictability of a
sequence based on empirical frequencies. We study stylized facts that cause
price predictability such as persistence of order signs, autocorrelation of
returns, and volatility clustering. We show that the degree of randomness grows
with the increase of aggregation level in transaction time. We also find that
predictable days are usually characterized by high trading activity, i.e., days
with unusually high trading volumes and the number of price changes. We find a
group of stocks for which predictability is caused by a frequent change of
price direction. We perform multiple testing for sub-intervals of days to
identify whether there is predictability at a specific time period during the
day.
MoreTranslated text
AI Read Science
Must-Reading Tree
Example
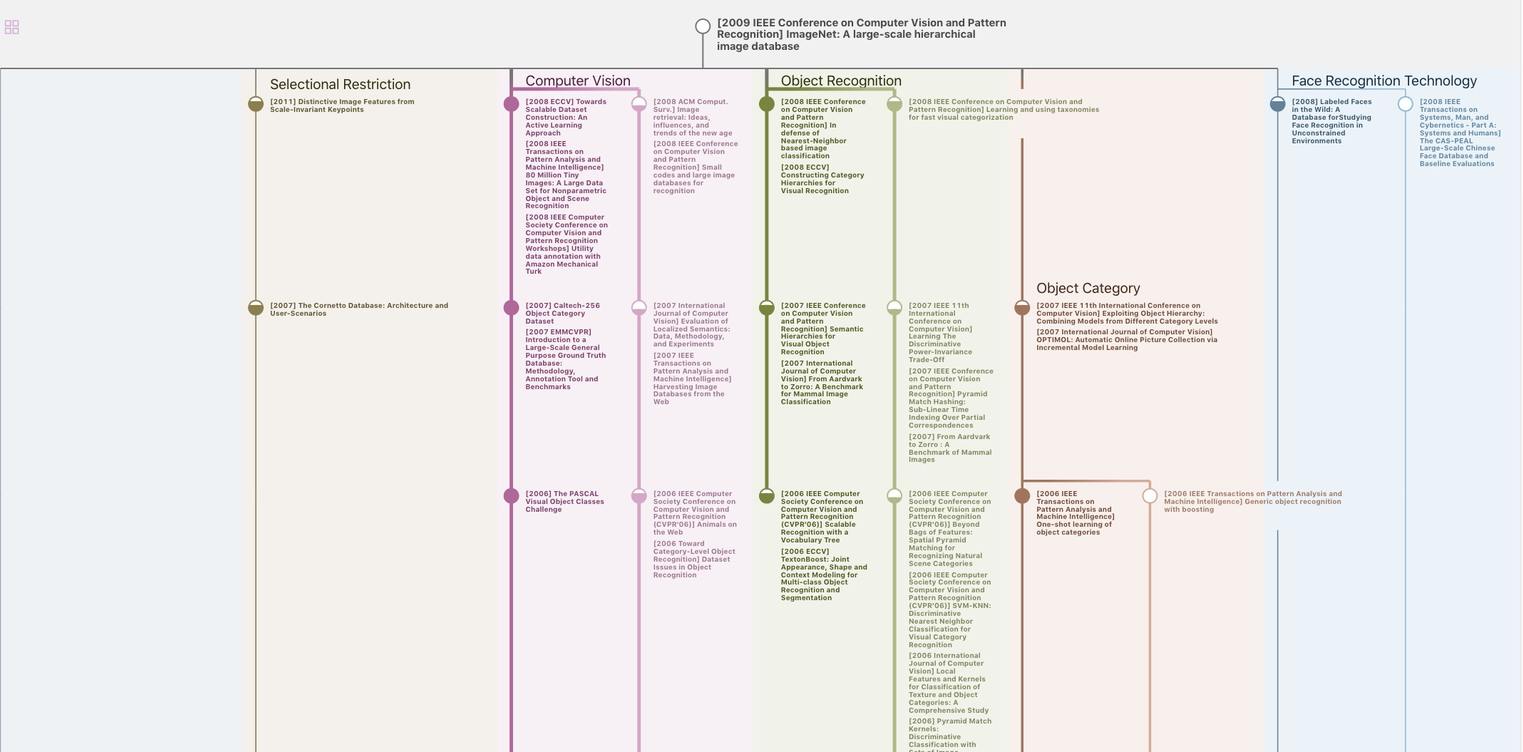
Generate MRT to find the research sequence of this paper
Chat Paper
Summary is being generated by the instructions you defined