Learn from orientation prior for radiograph super-resolution: Orientation operator transformer
COMPUTER METHODS AND PROGRAMS IN BIOMEDICINE(2024)
摘要
Background and objective: High -resolution radiographic images play a pivotal role in the early diagnosis and treatment of skeletal muscle -related diseases. It is promising to enhance image quality by introducing singleimage super -resolution (SISR) model into the radiology image field. However, the conventional image pipeline, which can learn a mixed mapping between SR and denoising from the color space and inter -pixel patterns, poses a particular challenge for radiographic images with limited pattern features. To address this issue, this paper introduces a novel approach: Orientation Operator Transformer - O2former. Methods: We incorporate an orientation operator in the encoder to enhance sensitivity to denoising mapping and to integrate orientation prior. Furthermore, we propose a multi -scale feature fusion strategy to amalgamate features captured by different receptive fields with the directional prior, thereby providing a more effective latent representation for the decoder. Based on these innovative components, we propose a transformer -based SISR model, i.e., O2former, specifically designed for radiographic images. Results: The experimental results demonstrate that our method achieves the best or second-best performance in the objective metrics compared with the competitors at x4 upsampling factor. For qualitative, more objective details are observed to be recovered. Conclusions: In this study, we propose a novel framework called O2former for radiological image super -resolution tasks, which improves the reconstruction model's performance by introducing an orientation operator and multi -scale feature fusion strategy. Our approach is promising to further promote the radiographic image enhancement field.
更多查看译文
关键词
Radiographs,Super-resolution,Orientation feature,Feature fusion
AI 理解论文
溯源树
样例
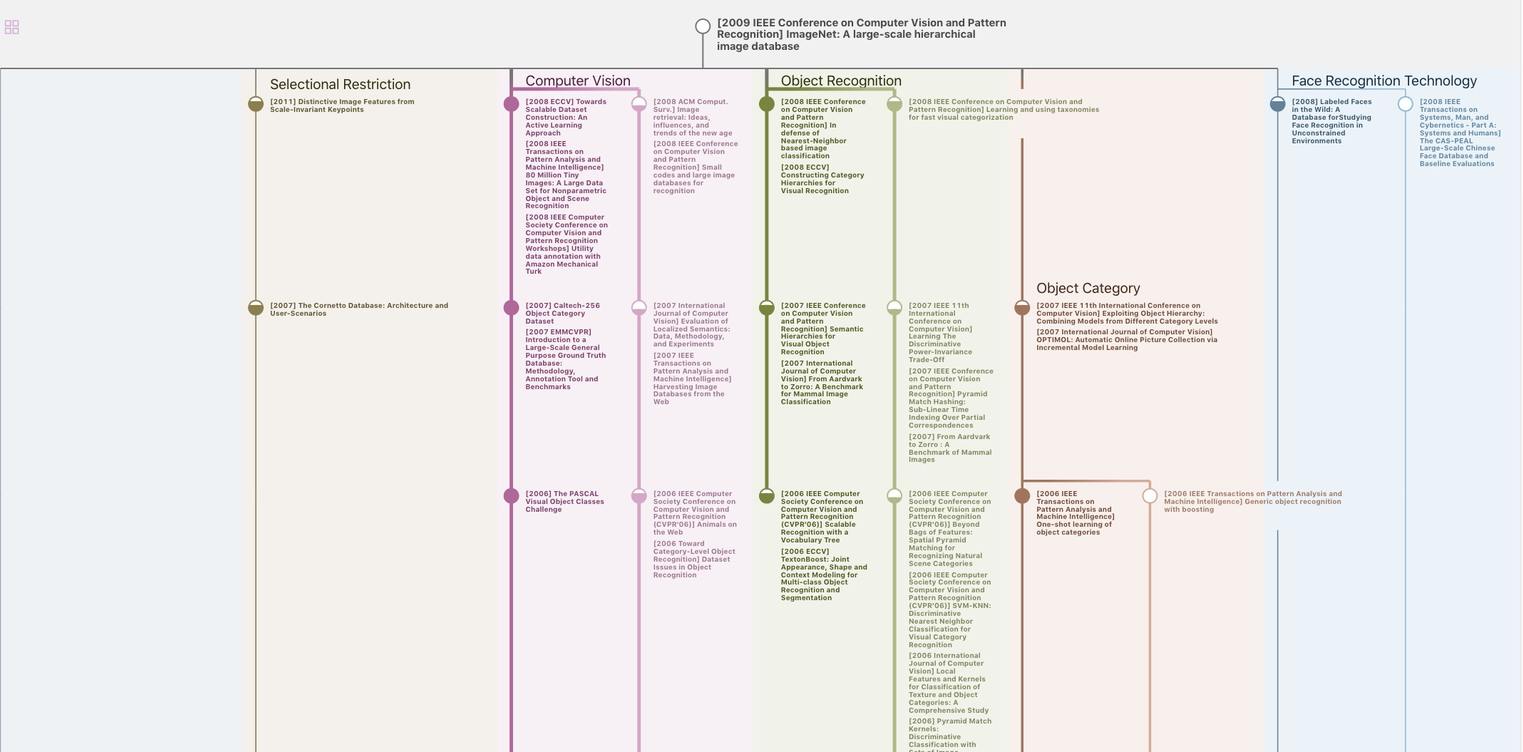
生成溯源树,研究论文发展脉络
Chat Paper
正在生成论文摘要