A blockchain-based framework for federated learning with privacy preservation in power load forecasting
KNOWLEDGE-BASED SYSTEMS(2024)
Abstract
Power load forecasting plays an important role in the efficient operation of power systems. For traditional load forecasting methods, the reliance on centralized data raises privacy concerns. Recently, federated learning has emerged as a promising solution for privacy-preserving forecasting. However, most federated learning frameworks still face security challenges such as single point of failure and data poisoning attacks. To address these issues, a novel federated learning framework based on consortium blockchain is proposed for decentralized and privacy-preserving power load forecasting. The framework includes a model verification mechanism to prevent low-quality model updates, and a flexible model aggregation scheme to improve prediction accuracy. Additionally, differential privacy techniques prevent inference attacks by adding noise to model updates. According to the experimental results, the proposed scheme can efficiently obstruct data poisoning attacks, ensure the privacy of model updates, and maintain the accuracy of power load forecasting.
MoreTranslated text
Key words
Federated learning,Blockchain,Power load forecasting,Differential privacy
AI Read Science
Must-Reading Tree
Example
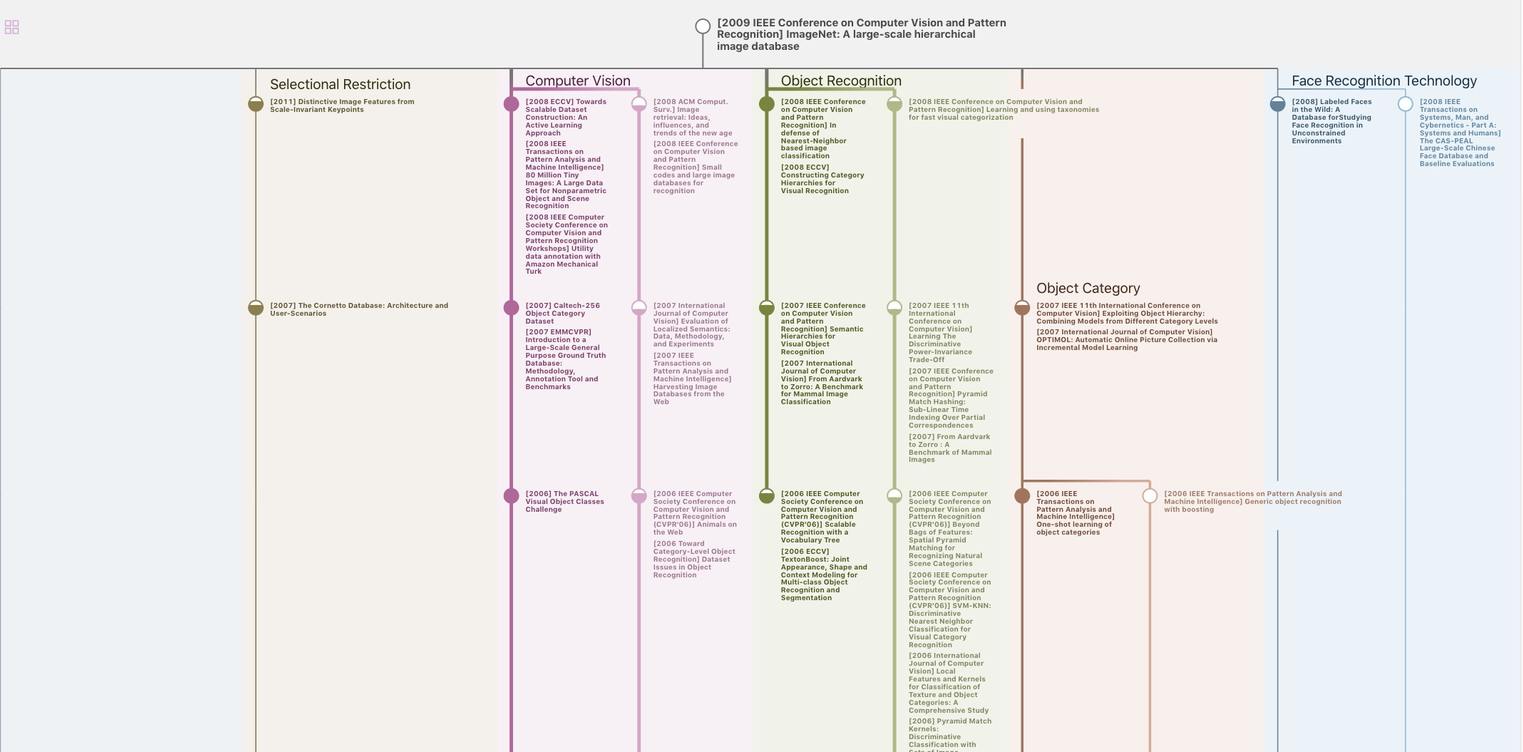
Generate MRT to find the research sequence of this paper
Chat Paper
Summary is being generated by the instructions you defined