Mask R-CNN based droplet detection in liquid-liquid systems, Part 3: Model generalization for accurate processing performance independent of image quality
CHEMICAL ENGINEERING RESEARCH & DESIGN(2024)
摘要
Operating processes involving liquid-liquid systems requires a sound understanding of fluid dynamics, mass transfer, and reaction kinetics, which strongly depend on droplet size distribution (DSD). Optical measurement is the most commonly used technique for DSD determination, followed by an appropriate image-processing method. However, the image-processing method is parameterized for a specific image quality, which varies depending on operating conditions and the setup of optical hardware. Generalizing the image-processing method Mask Region-Based Convolutional Neural Network (MRCNN) has the potential to robustly determine DSDs independently from varying image qualities. This work applies three generalization methods, namely augmentation, pre-processing, and training domain diversification, to generalize MRCNN. The droplet detection performance of the resulting generalized MRCNN model is compared with the manual processing for eight image qualities. The generalization of MRCNN by training domain diversification results in a robust model with high DSD detection accuracy. Averaged over the eight image qualities, the relative error in characteristic DSD properties is: Sauter mean diameter - 3 %, minimum diameter - 4%, and maximum diameter - 10 %. Since MRCNN shows an accurate and robust processing performance independent of the photo-optical measurement equipment and operating conditions, it can be implemented in continuous online monitoring of the DSD without training on specific image quality.
更多查看译文
关键词
Droplet size distribution,Process analysis and control,Sauter mean diameter,Object detection,Image processing,Convolutional neural networks
AI 理解论文
溯源树
样例
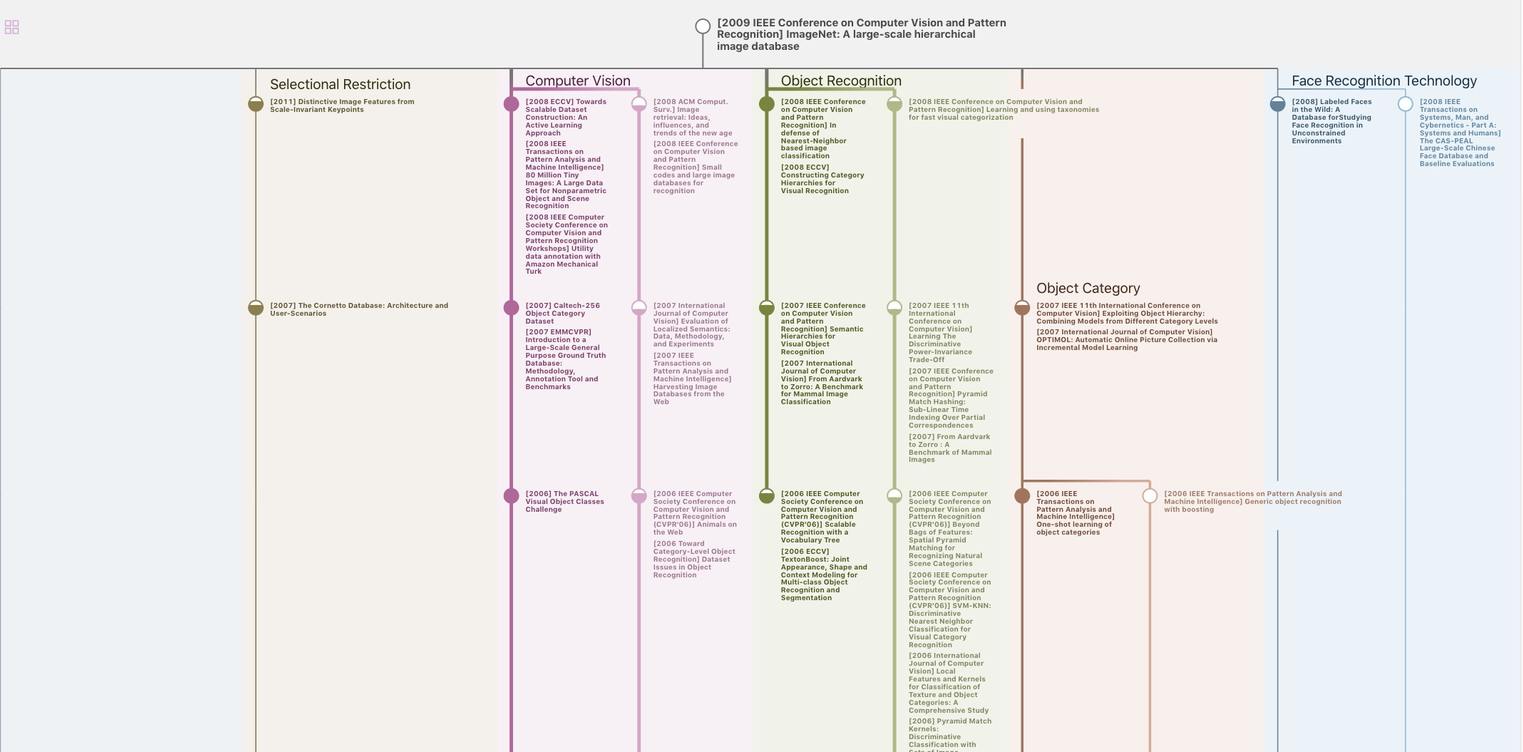
生成溯源树,研究论文发展脉络
Chat Paper
正在生成论文摘要