Optoelectronic device based failure management using content based multispectral image retrieval and deep learning model
Optical and Quantum Electronics(2024)
摘要
The need for optoelectronic devices is growing right now, but the production of these devices is having a difficult time keeping up with the advancement of the machinery, instruments, and manufacturing techniques they support. Pictures from big, unlabeled image collections are commonly retrieved using a technique called content-based image retrieval (CBIR). The availability of photographs is also growing as internet growth and transmission networks increase. This study suggests brand-new image retrieval methods for multispectral images used in Optoelectronic device monitoring that are based on image segmentation and classification methods with deep learningtechniques for failure management. Optoelectronic device Monitoring field-based multispectral images were used as the input, which was then processed for noise removal, resizing, and smoothing.Fuzzy c-means clustering-based image segmentation was used to divide up this processed image into its component parts. Following that, a hybrid multilayer transfer learning perception was used to classify the clustered segmented picture. The proposed technique has an accuracy of 95%, precision of 85%, recall of 75%, F-1 score of 63%, error rate of 51%, MAP of 55%; existing MRCN accuracy of 85%, precision of 75%, recall of 63%, F-1 score of 55%, error rate of 45%, MAP of 51%, OC-LBP accuracy of 89%, the precision of 79%, recall of 71%, F-1 score of 59%, error rate of 49%, MAP of 53%.
更多查看译文
关键词
Failure management,Optoelectronic device Monitoring,Multispectral image retrieval,Segmentation,Classification,Deep learning
AI 理解论文
溯源树
样例
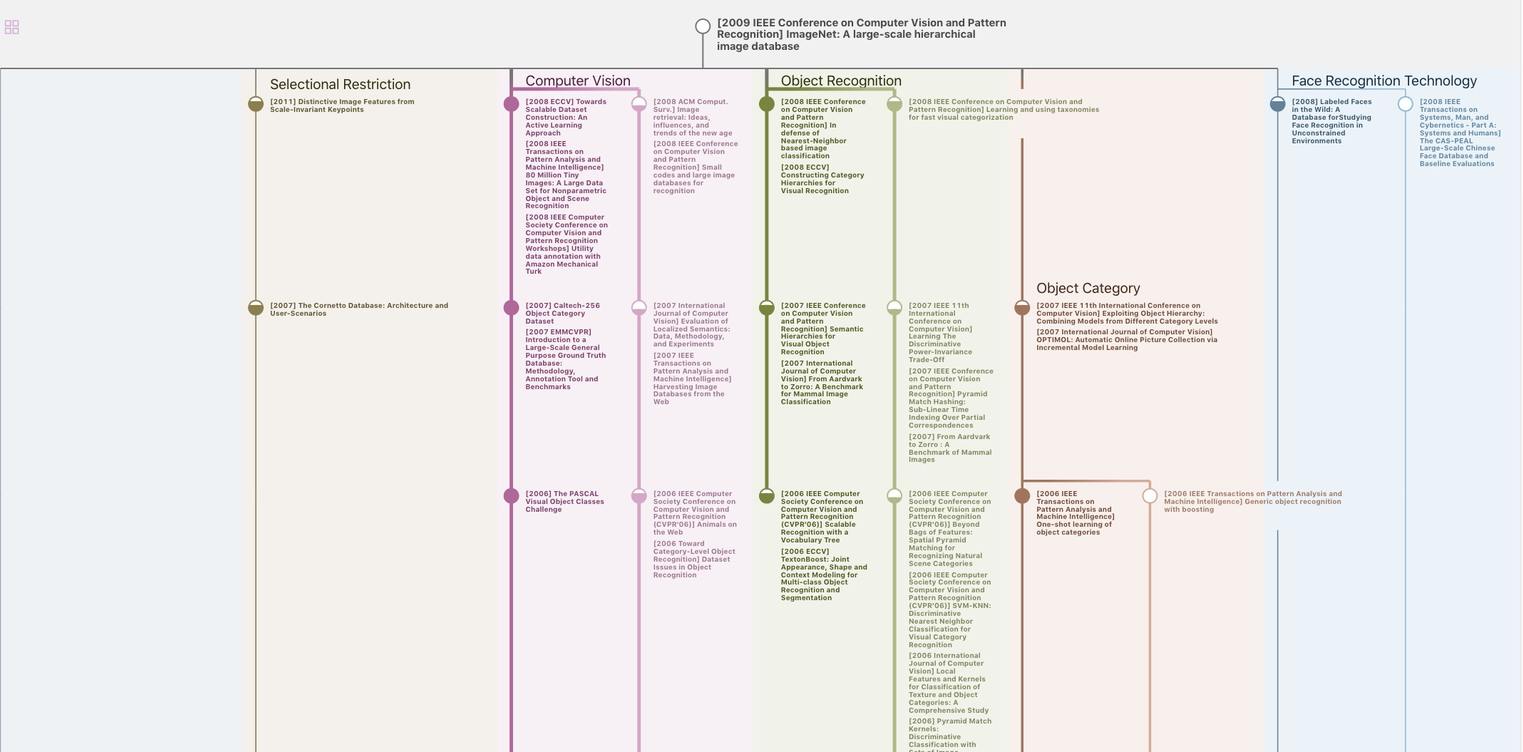
生成溯源树,研究论文发展脉络
Chat Paper
正在生成论文摘要