A self-learning knowledge-based MOEA/D for distributed heterogeneous assembly permutation flowshop scheduling with batch delivery
Knowledge-Based Systems(2024)
Abstract
With the requirements of just-in-time and make-to-order, it is necessary to consider the batch delivery in distributed heterogeneous assembly flow shop scheduling as well as the minimization of inventory and tardiness costs. Hence, this work proposes a new mixed integer linear programming model and a self-learning knowledge-based multi-objective evolutionary algorithm based on decomposition. In this algorithm, 4 problem-specific properties are extracted and embedded in the designed solution representation, forward-reverse objective calculation and local search operators. A new hybrid initialization with six knowledge-based NEH heuristics and a random generation is developed to generate better initial solutions. A self-learning selection strategy is developed to coordinate the above knowledge-based local search operators. The experimental study, based on 900 small and 540 large instances, demonstrates that the designed components such as hybrid initialization and self-learning selection strategy are effective, and the proposed algorithm is superior to 6 existing algorithms in the literature in solving this new problem.
MoreTranslated text
Key words
Distributed heterogeneous scheduling,Assembly permutation flowshop,Inventory,Batch delivery,Multi-objective optimization
AI Read Science
Must-Reading Tree
Example
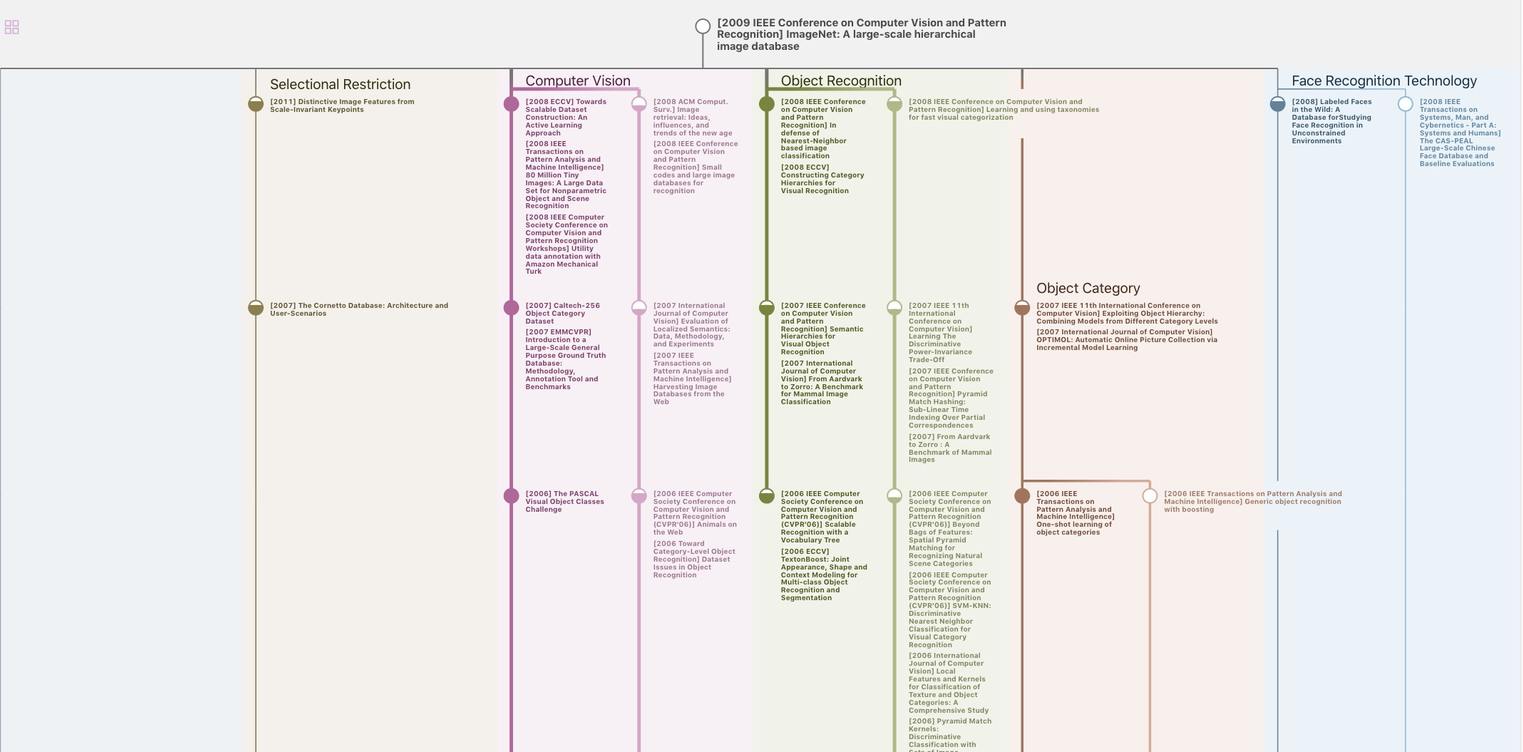
Generate MRT to find the research sequence of this paper
Chat Paper
Summary is being generated by the instructions you defined