A Self-Supervised Contrastive Denoising Autoencoder-Based Noise Suppression Method for Micro Thrust Measurement Signals Processing
IEEE TRANSACTIONS ON INSTRUMENTATION AND MEASUREMENT(2024)
摘要
Noise suppression is of great significance in high-precision micro thrust calibration and measurement. Existing methods cannot simultaneously recover both the nonlinear transitions (generated by thrust) and smooth trends of the signals. In addition, they exhibit poor robustness to noise model changes. To address the issues, in this research, we propose a novel self-supervised contrastive denoising autoencoder (SCDAE) model. The SCDAE features end-to-end signal denoising based on a multitask joint learning framework combining contrastive representation learning and denoising autoencoder (DAE) without the need for a clean signal reference. Specifically, SCDAE consists of a representation learner (RPL) and a denoising learner (DEL). In addition, considering the multistaircase property of the measurement signal, a novel parallel hybrid data augmentation strategy is designed in the RPL to create positive sample pairs and greatly force the network to extract robust content-invariant and disentangled features. Besides, in order to solve the blindness of training target creation for the DEL, a new training target creation strategy is proposed based on the variational mode decomposition (VMD). An attention module is introduced to adaptively reinforce the content-invariant disentangled features from the RPL, guiding the DEL to generate the essential dynamic nature from the raw input signal, thus suppressing noise information. Extensive simulations and experimental case study were carried out to demonstrate the effectiveness and advancement of the proposed method in recovering signal details (nonlinear transitions and smooth trends) with comparison to existing classical methods.
更多查看译文
关键词
Noise reduction,Noise measurement,Extraterrestrial measurements,Task analysis,Representation learning,Wiener filters,Physics,Contrastive learning,denoising,denoising autoencoder (DAE),micro thrust measurement signal,self-supervised learning
AI 理解论文
溯源树
样例
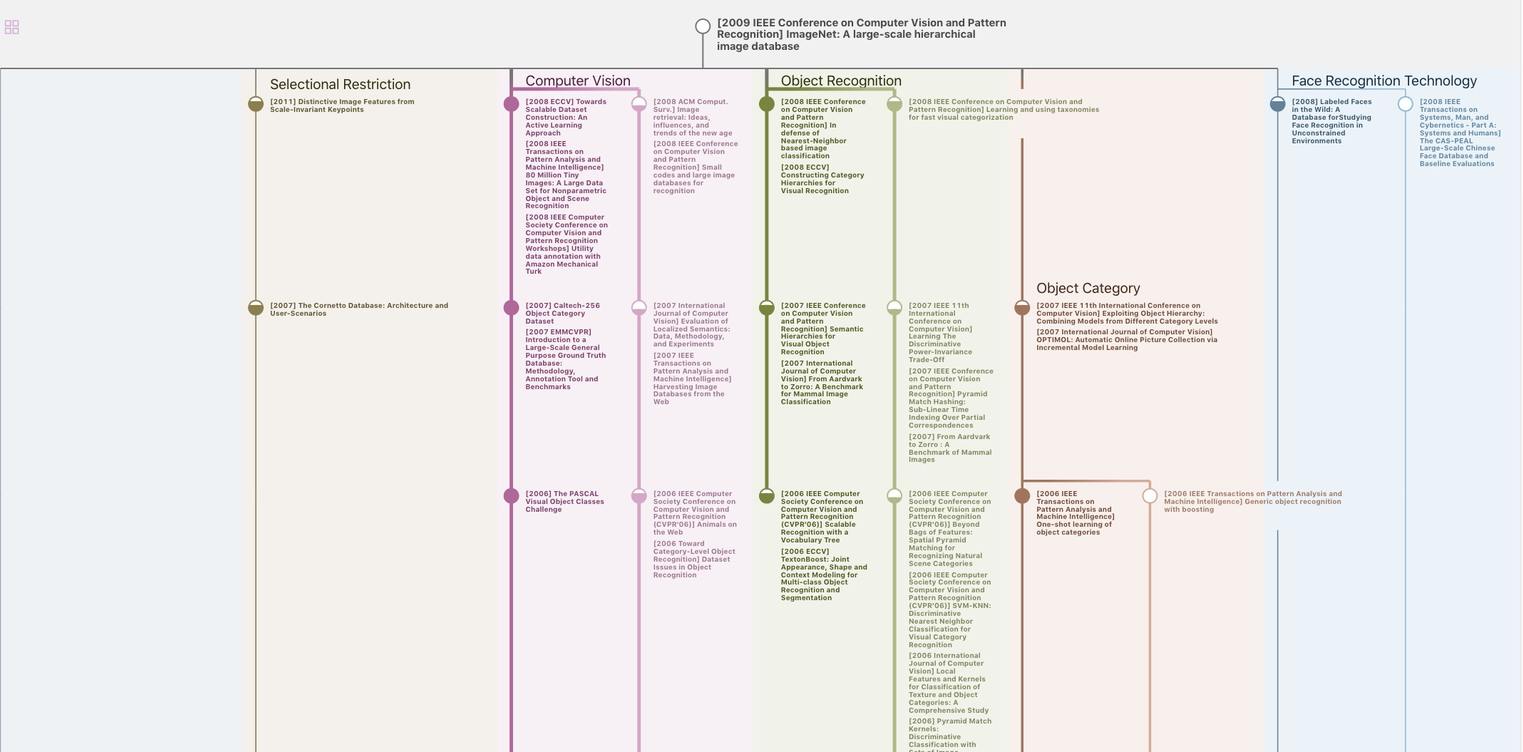
生成溯源树,研究论文发展脉络
Chat Paper
正在生成论文摘要